


Share a case of how Python uses plotly to draw data charts (pictures and texts)
This article mainly introduces the method of using plotly to draw data charts in Python. It analyzes the techniques of plotly drawing with examples and has certain reference value. Interested friends can refer to it
Introduction: Use The python-plotly module is used to draw stress test data and generate static html page results for display.
Many friends have the experience of stress testing modules during the development process. After the stress test, everyone often likes to use Excel to process the stress test data and draw data visualization views, but this is not very convenient to use. Web page for data display. This article will introduce the use of the python-plotly module to draw stress test data and generate a static HTML page to facilitate the display of results.
Introduction to Plotly
Plotly is a charting tool developed using JavaScript, providing an API to interact with mainstream data analysis languages (such as: Python, R, MATLAB). You can go to the official website https://plot.ly/ for more detailed information. Plotly is capable of drawing beautiful charts with user interaction.
Python-Plotly installation
This document mainly introduces the use of plotly’s Python API to perform several A simple chart drawing. For more usage of Plotly, please refer to https://plot.ly/python/
Python-Plotly can be installed using pip, and it is best to install and use it in Python version 2.7 and above. If you use Python2.6 version, please install Python2.7 and the corresponding pip yourself.
Plotly drawing example
line-plots
Drawing effect:
The generated html page provides rich interactive tools in the upper right corner.
Code:
def line_plots(name): ''' 绘制普通线图 ''' #数据,x为横坐标,y,z为纵坐标的两项指标,三个array长度相同 dataset = {'x':[0,1,2,3,4,5,6,7,8,9], 'y':[5,4,1,3,11,2,6,7,19,20], 'z':[12,9,0,0,3,25,8,17,22,5]} data_g = [] #分别插入 y, z tr_x = Scatter( x = dataset['x'], y = dataset['y'], name = 'y' ) data_g.append(tr_x) tr_z = Scatter( x = dataset['x'], y = dataset['z'], name = 'z' ) data_g.append(tr_z) #设置layout,指定图表title,x轴和y轴名称 layout = Layout(title="line plots", xaxis={'title':'x'}, yaxis={'title':'value'}) #将layout设置到图表 fig = Figure(data=data_g, layout=layout) #绘图,输出路径为name参数指定 pltoff.plot(fig, filename=name)
scatter-plots
Plotting effect :
Code:
def scatter_plots(name): ''' 绘制散点图 ''' dataset = {'x':[0,1,2,3,4,5,6,7,8,9], 'y':[5,4,1,3,11,2,6,7,19,20], 'text':['5_txt','4_txt','1_txt','3_txt','11_txt','2_txt','6_txt','7_txt','19_txt','20_txt']} data_g = [] tr_x = Scatter( x = dataset['x'], y = dataset['y'], text = dataset['text'], textposition='top center', mode='markers+text', name = 'y' ) data_g.append(tr_x) layout = Layout(title="scatter plots", xaxis={'title':'x'}, yaxis={'title':'value'}) fig = Figure(data=data_g, layout=layout) pltoff.plot(fig, filename=name)
bar-charts
Drawing Effect:
Code:
def bar_charts(name): ''' 绘制柱状图 ''' dataset = {'x':['Windows', 'Linux', 'Unix', 'MacOS'], 'y1':[45, 26, 37, 13], 'y2':[19, 27, 33, 21]} data_g = [] tr_y1 = Bar( x = dataset['x'], y = dataset['y1'], name = 'v1' ) data_g.append(tr_y1) tr_y2 = Bar( x = dataset['x'], y = dataset['y2'], name = 'v2' ) data_g.append(tr_y2) layout = Layout(title="bar charts", xaxis={'title':'x'}, yaxis={'title':'value'}) fig = Figure(data=data_g, layout=layout) pltoff.plot(fig, filename=name)
pie-charts
Plotting effect:
Code:
def pie_charts(name): ''' 绘制饼图 ''' dataset = {'labels':['Windows', 'Linux', 'Unix', 'MacOS', 'Android', 'iOS'], 'values':[280, 25, 10, 100, 250, 270]} data_g = [] tr_p = Pie( labels = dataset['labels'], values = dataset['values'] ) data_g.append(tr_p) layout = Layout(title="pie charts") fig = Figure(data=data_g, layout=layout) pltoff.plot(fig, filename=name)
filled-area-plots
This example is to draw a stacked line chart with a fill effect, which is suitable for analyzing data with stacked percentage attributes
Drawing effect:
Code:
def filled_area_plots(name): ''' 绘制堆叠填充的线图 ''' dataset = {'x':[0,1,2,3,4,5,6,7,8,9], 'y1':[5,4,1,3,11,2,6,7,19,20], 'y2':[12,9,0,0,3,25,8,17,22,5], 'y3':[13,22,46,1,15,4,18,11,17,20]} #计算y1,y2,y3的堆叠占比 dataset['y1_stack'] = dataset['y1'] dataset['y2_stack'] = [y1+y2 for y1, y2 in zip(dataset['y1'], dataset['y2'])] dataset['y3_stack'] = [y1+y2+y3 for y1, y2, y3 in zip(dataset['y1'], dataset['y2'], dataset['y3'])] dataset['y1_text'] = ['%s(%s%%)'%(y1, y1*100/y3_s) for y1, y3_s in zip(dataset['y1'], dataset['y3_stack'])] dataset['y2_text'] = ['%s(%s%%)'%(y2, y2*100/y3_s) for y2, y3_s in zip(dataset['y2'], dataset['y3_stack'])] dataset['y3_text'] = ['%s(%s%%)'%(y3, y3*100/y3_s) for y3, y3_s in zip(dataset['y3'], dataset['y3_stack'])] data_g = [] tr_1 = Scatter( x = dataset['x'], y = dataset['y1_stack'], text = dataset['y1_text'], hoverinfo = 'x+text', mode = 'lines', name = 'y1', fill = 'tozeroy' #填充方式: 到x轴 ) data_g.append(tr_1) tr_2 = Scatter( x = dataset['x'], y = dataset['y2_stack'], text = dataset['y2_text'], hoverinfo = 'x+text', mode = 'lines', name = 'y2', fill = 'tonexty' #填充方式:到下方的另一条线 ) data_g.append(tr_2) tr_3 = Scatter( x = dataset['x'], y = dataset['y3_stack'], text = dataset['y3_text'], hoverinfo = 'x+text', mode = 'lines', name = 'y3', fill = 'tonexty' ) data_g.append(tr_3) layout = Layout(title="field area plots", xaxis={'title':'x'}, yaxis={'title':'value'}) fig = Figure(data=data_g, layout=layout) pltoff.plot(fig, filename=name)
Summary
This article introduces the method of using python-plotly to draw data graphs, including line plots and scatter plots in examples. These five typical charts, scatter plots, bar charts, pie charts, and filled area plots, basically cover most types of test data. Dear friends, It can be deformed to draw more beautiful icons.
The above is the detailed content of Share a case of how Python uses plotly to draw data charts (pictures and texts). For more information, please follow other related articles on the PHP Chinese website!

Hot AI Tools

Undresser.AI Undress
AI-powered app for creating realistic nude photos

AI Clothes Remover
Online AI tool for removing clothes from photos.

Undress AI Tool
Undress images for free

Clothoff.io
AI clothes remover

Video Face Swap
Swap faces in any video effortlessly with our completely free AI face swap tool!

Hot Article

Hot Tools

Notepad++7.3.1
Easy-to-use and free code editor

SublimeText3 Chinese version
Chinese version, very easy to use

Zend Studio 13.0.1
Powerful PHP integrated development environment

Dreamweaver CS6
Visual web development tools

SublimeText3 Mac version
God-level code editing software (SublimeText3)

Hot Topics










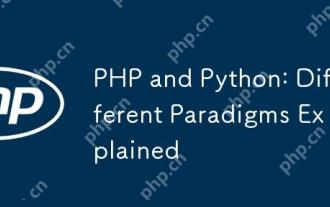
PHP is mainly procedural programming, but also supports object-oriented programming (OOP); Python supports a variety of paradigms, including OOP, functional and procedural programming. PHP is suitable for web development, and Python is suitable for a variety of applications such as data analysis and machine learning.
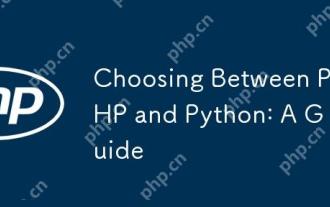
PHP is suitable for web development and rapid prototyping, and Python is suitable for data science and machine learning. 1.PHP is used for dynamic web development, with simple syntax and suitable for rapid development. 2. Python has concise syntax, is suitable for multiple fields, and has a strong library ecosystem.
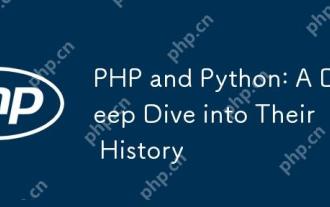
PHP originated in 1994 and was developed by RasmusLerdorf. It was originally used to track website visitors and gradually evolved into a server-side scripting language and was widely used in web development. Python was developed by Guidovan Rossum in the late 1980s and was first released in 1991. It emphasizes code readability and simplicity, and is suitable for scientific computing, data analysis and other fields.
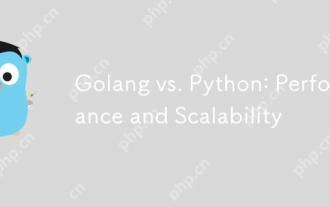
Golang is better than Python in terms of performance and scalability. 1) Golang's compilation-type characteristics and efficient concurrency model make it perform well in high concurrency scenarios. 2) Python, as an interpreted language, executes slowly, but can optimize performance through tools such as Cython.
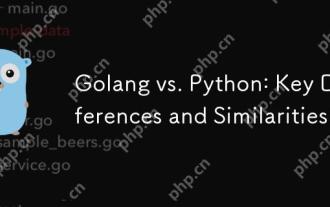
Golang and Python each have their own advantages: Golang is suitable for high performance and concurrent programming, while Python is suitable for data science and web development. Golang is known for its concurrency model and efficient performance, while Python is known for its concise syntax and rich library ecosystem.
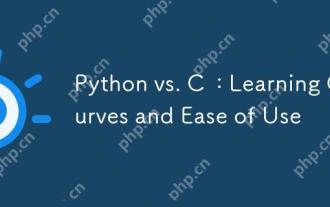
Python is easier to learn and use, while C is more powerful but complex. 1. Python syntax is concise and suitable for beginners. Dynamic typing and automatic memory management make it easy to use, but may cause runtime errors. 2.C provides low-level control and advanced features, suitable for high-performance applications, but has a high learning threshold and requires manual memory and type safety management.
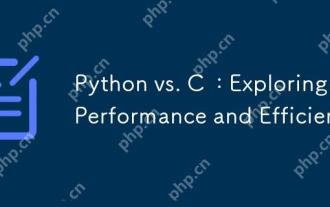
Python is better than C in development efficiency, but C is higher in execution performance. 1. Python's concise syntax and rich libraries improve development efficiency. 2.C's compilation-type characteristics and hardware control improve execution performance. When making a choice, you need to weigh the development speed and execution efficiency based on project needs.
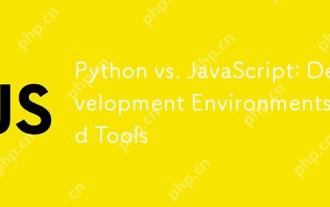
Both Python and JavaScript's choices in development environments are important. 1) Python's development environment includes PyCharm, JupyterNotebook and Anaconda, which are suitable for data science and rapid prototyping. 2) The development environment of JavaScript includes Node.js, VSCode and Webpack, which are suitable for front-end and back-end development. Choosing the right tools according to project needs can improve development efficiency and project success rate.
