


Metas offizieller Prompt-Projektleitfaden: Llama 2 ist effizienter, wenn es auf diese Weise verwendet wird
Mit zunehmender Reife der LLM-Technologie (Large Language Model) wird schnelles Engineering immer wichtiger. Mehrere Forschungseinrichtungen haben LLM-Prompt-Engineering-Richtlinien veröffentlicht, darunter Microsoft, OpenAI und mehr.
Vor Kurzem hat Meta einen interaktiven Prompt-Engineering-Leitfaden speziell für sein Open-Source-Modell Llama 2 bereitgestellt. Dieser Leitfaden behandelt schnelles Engineering und Best Practices für die Verwendung von Llama 2.
Das Folgende ist der Kerninhalt dieses Leitfadens.
Llama-Modell
Im Jahr 2023 brachte Meta die Modelle Llama und Llama 2 auf den Markt. Kleinere Modelle sind kostengünstiger in der Bereitstellung und im Betrieb, während größere Modelle leistungsfähiger sind.
Die Modellparameterskalen der Llama 2-Serie lauten wie folgt:
Code Llama ist ein codezentriertes LLM, das auf der Basis von Llama 2 erstellt wurde. Darüber hinaus gibt es verschiedene Parameterskalen und Feinabstimmungsvarianten:
Bereitstellen von LLM
LLM kann auf verschiedene Arten bereitgestellt und darauf zugegriffen werden, darunter:
Selbsthosting: Verwenden Sie lokale Hardware, um Inferenz auszuführen, z. B. mit llama.cpp in Llama 2, das auf dem Macbook Pro läuft. Vorteile: Selbst gehostet ist am besten, wenn Sie Datenschutz-/Sicherheitsanforderungen haben oder über genügend GPUs verfügen.
Cloud-Hosting: Verlassen Sie sich auf einen Cloud-Anbieter, um Instanzen bereitzustellen, die bestimmte Modelle hosten, z. B. die Ausführung von Llama 2 über Cloud-Anbieter wie AWS, Azure, GCP usw. Vorteile: Cloud-Hosting ist die beste Möglichkeit, Ihr Modell und seine Laufzeit anzupassen.
Gehostete API: Rufen Sie LLM direkt über die API auf. Es gibt viele Unternehmen, die Llama 2-Inferenz-APIs anbieten, darunter AWS Bedrock, Replicate, Anyscale, Together und andere. Vorteile: Die gehostete API ist insgesamt die einfachste Option.
Gehostete API
Gehostete API hat normalerweise zwei Hauptendpunkte:
1. Abschluss: Erzeugt eine Antwort auf die gegebene Eingabeaufforderung.
2. chat_completion: Erzeugt die nächste Nachricht in der Nachrichtenliste und bietet klarere Anweisungen und Kontext für Anwendungsfälle wie Chatbots.
Token
LLM verarbeitet Eingabe und Ausgabe in Form von Blöcken, die als Token bezeichnet werden, und jedes Modell verfügt über sein eigenes Tokenisierungsschema. Zum Beispiel der folgende Satz:
Unser Schicksal steht in den Sternen.
Die Tokenisierung von Lama 2 ist [„our“, „dest“, „iny“, „is“, „writing“, „in“, "die Sterne"]. Token sind besonders wichtig, wenn es um API-Preise und internes Verhalten (z. B. Hyperparameter) geht. Jedes Modell hat eine maximale Kontextlänge, die die Eingabeaufforderung nicht überschreiten darf, Llama 2 beträgt 4096 Token und Code Llama beträgt 100.000 Token.
Notebook-Setup
Als Beispiel verwenden wir Replicate, um den Llama 2-Chat aufzurufen, und verwenden LangChain, um die Chat-Abschluss-API einfach einzurichten. 🔜 Diese Nachrichtenliste liefert LLM einige „Hintergrund“- oder „historische“ Informationen, mit denen es fortfahren kann.
Typischerweise enthält jede Nachricht Rollen und Inhalte:
Nachrichten mit Systemrollen werden von Entwicklern verwendet, um LLM Kernanweisungen bereitzustellen.
Nachrichten mit Benutzerrollen sind normalerweise von Menschen bereitgestellte Nachrichten.Nachrichten mit Assistentenrolle werden in der Regel von LLM generiert.
pip install langchain replicate
LLM-Hyperparameter
LLM API 通常会采用影响输出的创造性和确定性的参数。在每一步中,LLM 都会生成 token 及其概率的列表。可能性最小的 token 会从列表中「剪切」(基于 top_p),然后从剩余候选者中随机(温度参数 temperature)选择一个 token。换句话说:top_p 控制生成中词汇的广度,温度控制词汇的随机性,温度参数 temperature 为 0 会产生几乎确定的结果。
def print_tuned_completion (temperature: float, top_p: float):response = completion ("Write a haiku about llamas", temperature=temperature, top_p=top_p)print (f'[temperature: {temperature} | top_p: {top_p}]\n {response.strip ()}\n')print_tuned_completion (0.01, 0.01)print_tuned_completion (0.01, 0.01)# These two generations are highly likely to be the sameprint_tuned_completion (1.0, 1.0)print_tuned_completion (1.0, 1.0)# These two generations are highly likely to be different
prompt 技巧
详细、明确的指令会比开放式 prompt 产生更好的结果:
complete_and_print (prompt="Describe quantum physics in one short sentence of no more than 12 words")# Returns a succinct explanation of quantum physics that mentions particles and states existing simultaneously.
我们可以给定使用规则和限制,以给出明确的指令。
- 风格化,例如:
- 向我解释一下这一点,就像儿童教育网络节目中教授小学生一样;
- 我是一名软件工程师,使用大型语言模型进行摘要。用 250 字概括以下文字;
- 像私家侦探一样一步步追查案件,给出你的答案。
- 格式化
使用要点;
以 JSON 对象形式返回;
使用较少的技术术语并用于工作交流中。
- 限制
- 仅使用学术论文;
- 切勿提供 2020 年之前的来源;
- 如果你不知道答案,就说你不知道。
以下是给出明确指令的例子:
complete_and_print ("Explain the latest advances in large language models to me.")# More likely to cite sources from 2017complete_and_print ("Explain the latest advances in large language models to me. Always cite your sources. Never cite sources older than 2020.")# Gives more specific advances and only cites sources from 2020
零样本 prompting
一些大型语言模型(例如 Llama 2)能够遵循指令并产生响应,而无需事先看过任务示例。没有示例的 prompting 称为「零样本 prompting(zero-shot prompting)」。例如:
complete_and_print ("Text: This was the best movie I've ever seen! \n The sentiment of the text is:")# Returns positive sentimentcomplete_and_print ("Text: The director was trying too hard. \n The sentiment of the text is:")# Returns negative sentiment
少样本 prompting
添加所需输出的具体示例通常会产生更加准确、一致的输出。这种方法称为「少样本 prompting(few-shot prompting)」。例如:
def sentiment (text):response = chat_completion (messages=[user ("You are a sentiment classifier. For each message, give the percentage of positive/netural/negative."),user ("I liked it"),assistant ("70% positive 30% neutral 0% negative"),user ("It could be better"),assistant ("0% positive 50% neutral 50% negative"),user ("It's fine"),assistant ("25% positive 50% neutral 25% negative"),user (text),])return responsedef print_sentiment (text):print (f'INPUT: {text}')print (sentiment (text))print_sentiment ("I thought it was okay")# More likely to return a balanced mix of positive, neutral, and negativeprint_sentiment ("I loved it!")# More likely to return 100% positiveprint_sentiment ("Terrible service 0/10")# More likely to return 100% negative
Role Prompting
Llama 2 在指定角色时通常会给出更一致的响应,角色为 LLM 提供了所需答案类型的背景信息。
例如,让 Llama 2 对使用 PyTorch 的利弊问题创建更有针对性的技术回答:
complete_and_print ("Explain the pros and cons of using PyTorch.")# More likely to explain the pros and cons of PyTorch covers general areas like documentation, the PyTorch community, and mentions a steep learning curvecomplete_and_print ("Your role is a machine learning expert who gives highly technical advice to senior engineers who work with complicated datasets. Explain the pros and cons of using PyTorch.")# Often results in more technical benefits and drawbacks that provide more technical details on how model layers
思维链
简单地添加一个「鼓励逐步思考」的短语可以显著提高大型语言模型执行复杂推理的能力(Wei et al. (2022)),这种方法称为 CoT 或思维链 prompting:
complete_and_print ("Who lived longer Elvis Presley or Mozart?")# Often gives incorrect answer of "Mozart"complete_and_print ("Who lived longer Elvis Presley or Mozart? Let's think through this carefully, step by step.")# Gives the correct answer "Elvis"
自洽性(Self-Consistency)
LLM 是概率性的,因此即使使用思维链,一次生成也可能会产生不正确的结果。自洽性通过从多次生成中选择最常见的答案来提高准确性(以更高的计算成本为代价):
import refrom statistics import modedef gen_answer ():response = completion ("John found that the average of 15 numbers is 40.""If 10 is added to each number then the mean of the numbers is?""Report the answer surrounded by three backticks, for example:```123```",model = LLAMA2_70B_CHAT)match = re.search (r'```(\d+)```', response)if match is None:return Nonereturn match.group (1)answers = [gen_answer () for i in range (5)]print (f"Answers: {answers}\n",f"Final answer: {mode (answers)}",)# Sample runs of Llama-2-70B (all correct):# [50, 50, 750, 50, 50]-> 50# [130, 10, 750, 50, 50] -> 50# [50, None, 10, 50, 50] -> 50
检索增强生成
有时我们可能希望在应用程序中使用事实知识,那么可以从开箱即用(即仅使用模型权重)的大模型中提取常见事实:
complete_and_print ("What is the capital of the California?", model = LLAMA2_70B_CHAT)# Gives the correct answer "Sacramento"
然而,LLM 往往无法可靠地检索更具体的事实或私人信息。模型要么声明它不知道,要么幻想出一个错误的答案:
complete_and_print ("What was the temperature in Menlo Park on December 12th, 2023?")# "I'm just an AI, I don't have access to real-time weather data or historical weather records."complete_and_print ("What time is my dinner reservation on Saturday and what should I wear?")# "I'm not able to access your personal information [..] I can provide some general guidance"
检索增强生成(RAG)是指在 prompt 中包含从外部数据库检索的信息(Lewis et al. (2020))。RAG 是将事实纳入 LLM 应用的有效方法,并且比微调更经济实惠,微调可能成本高昂并对基础模型的功能产生负面影响。
MENLO_PARK_TEMPS = {"2023-12-11": "52 degrees Fahrenheit","2023-12-12": "51 degrees Fahrenheit","2023-12-13": "51 degrees Fahrenheit",}def prompt_with_rag (retrived_info, question):complete_and_print (f"Given the following information: '{retrived_info}', respond to: '{question}'")def ask_for_temperature (day):temp_on_day = MENLO_PARK_TEMPS.get (day) or "unknown temperature"prompt_with_rag (f"The temperature in Menlo Park was {temp_on_day} on {day}'",# Retrieved factf"What is the temperature in Menlo Park on {day}?",# User question)ask_for_temperature ("2023-12-12")# "Sure! The temperature in Menlo Park on 2023-12-12 was 51 degrees Fahrenheit."ask_for_temperature ("2023-07-18")# "I'm not able to provide the temperature in Menlo Park on 2023-07-18 as the information provided states that the temperature was unknown."
程序辅助语言模型
LLM 本质上不擅长执行计算,例如:
complete_and_print ("""Calculate the answer to the following math problem:((-5 + 93 * 4 - 0) * (4^4 + -7 + 0 * 5))""")# Gives incorrect answers like 92448, 92648, 95463
Gao et al. (2022) 提出「程序辅助语言模型(Program-aided Language Models,PAL)」的概念。虽然 LLM 不擅长算术,但它们非常擅长代码生成。PAL 通过指示 LLM 编写代码来解决计算任务。
complete_and_print ("""# Python code to calculate: ((-5 + 93 * 4 - 0) * (4^4 + -7 + 0 * 5))""",model="meta/codellama-34b:67942fd0f55b66da802218a19a8f0e1d73095473674061a6ea19f2dc8c053152")
# The following code was generated by Code Llama 34B:num1 = (-5 + 93 * 4 - 0)num2 = (4**4 + -7 + 0 * 5)answer = num1 * num2print (answer)
Das obige ist der detaillierte Inhalt vonMetas offizieller Prompt-Projektleitfaden: Llama 2 ist effizienter, wenn es auf diese Weise verwendet wird. Für weitere Informationen folgen Sie bitte anderen verwandten Artikeln auf der PHP chinesischen Website!

Heiße KI -Werkzeuge

Undresser.AI Undress
KI-gestützte App zum Erstellen realistischer Aktfotos

AI Clothes Remover
Online-KI-Tool zum Entfernen von Kleidung aus Fotos.

Undress AI Tool
Ausziehbilder kostenlos

Clothoff.io
KI-Kleiderentferner

AI Hentai Generator
Erstellen Sie kostenlos Ai Hentai.

Heißer Artikel

Heiße Werkzeuge

Notepad++7.3.1
Einfach zu bedienender und kostenloser Code-Editor

SublimeText3 chinesische Version
Chinesische Version, sehr einfach zu bedienen

Senden Sie Studio 13.0.1
Leistungsstarke integrierte PHP-Entwicklungsumgebung

Dreamweaver CS6
Visuelle Webentwicklungstools

SublimeText3 Mac-Version
Codebearbeitungssoftware auf Gottesniveau (SublimeText3)

Heiße Themen
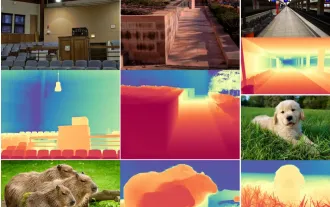
0.Was bewirkt dieser Artikel? Wir schlagen DepthFM vor: ein vielseitiges und schnelles generatives monokulares Tiefenschätzungsmodell auf dem neuesten Stand der Technik. Zusätzlich zu herkömmlichen Tiefenschätzungsaufgaben demonstriert DepthFM auch hochmoderne Fähigkeiten bei nachgelagerten Aufgaben wie dem Tiefen-Inpainting. DepthFM ist effizient und kann Tiefenkarten innerhalb weniger Inferenzschritte synthetisieren. Lassen Sie uns diese Arbeit gemeinsam lesen ~ 1. Titel der Papierinformationen: DepthFM: FastMonocularDepthEstimationwithFlowMatching Autor: MingGui, JohannesS.Fischer, UlrichPrestel, PingchuanMa, Dmytr
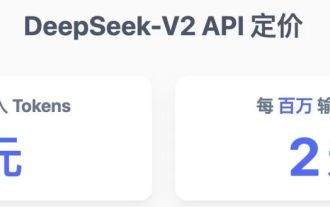
Stellen Sie sich ein Modell der künstlichen Intelligenz vor, das nicht nur die Fähigkeit besitzt, die traditionelle Datenverarbeitung zu übertreffen, sondern auch eine effizientere Leistung zu geringeren Kosten erzielt. Dies ist keine Science-Fiction, DeepSeek-V2[1], das weltweit leistungsstärkste Open-Source-MoE-Modell, ist da. DeepSeek-V2 ist ein leistungsstarkes MoE-Sprachmodell (Mix of Experts) mit den Merkmalen eines wirtschaftlichen Trainings und einer effizienten Inferenz. Es besteht aus 236B Parametern, von denen 21B zur Aktivierung jedes Markers verwendet werden. Im Vergleich zu DeepSeek67B bietet DeepSeek-V2 eine stärkere Leistung, spart gleichzeitig 42,5 % der Trainingskosten, reduziert den KV-Cache um 93,3 % und erhöht den maximalen Generierungsdurchsatz auf das 5,76-fache. DeepSeek ist ein Unternehmen, das sich mit allgemeiner künstlicher Intelligenz beschäftigt
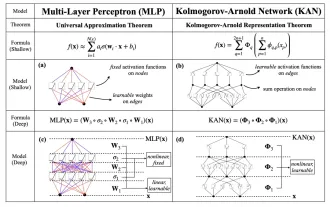
Anfang dieses Monats schlugen Forscher des MIT und anderer Institutionen eine vielversprechende Alternative zu MLP vor – KAN. KAN übertrifft MLP in Bezug auf Genauigkeit und Interpretierbarkeit. Und es kann MLP, das mit einer größeren Anzahl von Parametern ausgeführt wird, mit einer sehr kleinen Anzahl von Parametern übertreffen. Beispielsweise gaben die Autoren an, dass sie KAN nutzten, um die Ergebnisse von DeepMind mit einem kleineren Netzwerk und einem höheren Automatisierungsgrad zu reproduzieren. Konkret verfügt DeepMinds MLP über etwa 300.000 Parameter, während KAN nur etwa 200 Parameter hat. KAN hat eine starke mathematische Grundlage wie MLP und basiert auf dem universellen Approximationssatz, während KAN auf dem Kolmogorov-Arnold-Darstellungssatz basiert. Wie in der folgenden Abbildung gezeigt, hat KAN
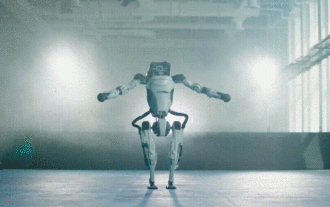
Boston Dynamics Atlas tritt offiziell in die Ära der Elektroroboter ein! Gestern hat sich der hydraulische Atlas einfach „unter Tränen“ von der Bühne der Geschichte zurückgezogen. Heute gab Boston Dynamics bekannt, dass der elektrische Atlas im Einsatz ist. Es scheint, dass Boston Dynamics im Bereich kommerzieller humanoider Roboter entschlossen ist, mit Tesla zu konkurrieren. Nach der Veröffentlichung des neuen Videos wurde es innerhalb von nur zehn Stunden bereits von mehr als einer Million Menschen angesehen. Die alten Leute gehen und neue Rollen entstehen. Das ist eine historische Notwendigkeit. Es besteht kein Zweifel, dass dieses Jahr das explosive Jahr der humanoiden Roboter ist. Netizens kommentierten: Die Weiterentwicklung der Roboter hat dazu geführt, dass die diesjährige Eröffnungsfeier wie Menschen aussieht, und der Freiheitsgrad ist weitaus größer als der von Menschen. Aber ist das wirklich kein Horrorfilm? Zu Beginn des Videos liegt Atlas ruhig auf dem Boden, scheinbar auf dem Rücken. Was folgt, ist atemberaubend
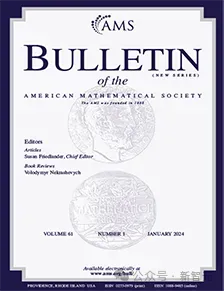
KI verändert tatsächlich die Mathematik. Vor kurzem hat Tao Zhexuan, der diesem Thema große Aufmerksamkeit gewidmet hat, die neueste Ausgabe des „Bulletin of the American Mathematical Society“ (Bulletin der American Mathematical Society) weitergeleitet. Zum Thema „Werden Maschinen die Mathematik verändern?“ äußerten viele Mathematiker ihre Meinung. Der gesamte Prozess war voller Funken, knallhart und aufregend. Der Autor verfügt über eine starke Besetzung, darunter der Fields-Medaillengewinner Akshay Venkatesh, der chinesische Mathematiker Zheng Lejun, der NYU-Informatiker Ernest Davis und viele andere bekannte Wissenschaftler der Branche. Die Welt der KI hat sich dramatisch verändert. Viele dieser Artikel wurden vor einem Jahr eingereicht.
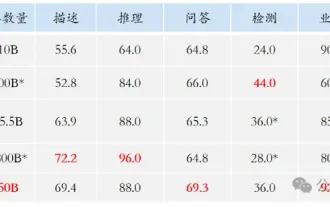
Ich weine zu Tode. Die Daten im Internet reichen überhaupt nicht aus. Das Trainingsmodell sieht aus wie „Die Tribute von Panem“, und KI-Forscher auf der ganzen Welt machen sich Gedanken darüber, wie sie diese datenhungrigen Esser ernähren sollen. Dieses Problem tritt insbesondere bei multimodalen Aufgaben auf. Zu einer Zeit, als sie ratlos waren, nutzte ein Start-up-Team der Abteilung der Renmin-Universität von China sein eigenes neues Modell, um als erstes in China einen „modellgenerierten Datenfeed selbst“ in die Realität umzusetzen. Darüber hinaus handelt es sich um einen zweigleisigen Ansatz auf der Verständnisseite und der Generierungsseite. Beide Seiten können hochwertige, multimodale neue Daten generieren und Datenrückmeldungen an das Modell selbst liefern. Was ist ein Modell? Awaker 1.0, ein großes multimodales Modell, das gerade im Zhongguancun-Forum erschienen ist. Wer ist das Team? Sophon-Motor. Gegründet von Gao Yizhao, einem Doktoranden an der Hillhouse School of Artificial Intelligence der Renmin University.
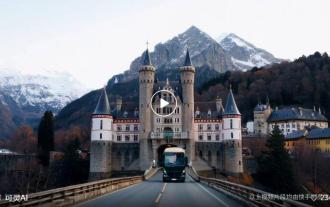
Was? Wird Zootopia durch heimische KI in die Realität umgesetzt? Zusammen mit dem Video wird ein neues groß angelegtes inländisches Videogenerationsmodell namens „Keling“ vorgestellt. Sora geht einen ähnlichen technischen Weg und kombiniert eine Reihe selbst entwickelter technologischer Innovationen, um Videos zu produzieren, die nicht nur große und vernünftige Bewegungen aufweisen, sondern auch die Eigenschaften der physischen Welt simulieren und über starke konzeptionelle Kombinationsfähigkeiten und Vorstellungskraft verfügen. Den Daten zufolge unterstützt Keling die Erstellung ultralanger Videos von bis zu 2 Minuten mit 30 Bildern pro Sekunde, mit Auflösungen von bis zu 1080p und unterstützt mehrere Seitenverhältnisse. Ein weiterer wichtiger Punkt ist, dass es sich bei Keling nicht um eine vom Labor veröffentlichte Demo oder Video-Ergebnisdemonstration handelt, sondern um eine Anwendung auf Produktebene, die von Kuaishou, einem führenden Anbieter im Bereich Kurzvideos, gestartet wurde. Darüber hinaus liegt das Hauptaugenmerk darauf, pragmatisch zu sein, keine Blankoschecks auszustellen und sofort nach der Veröffentlichung online zu gehen. Das große Modell von Ke Ling wurde bereits in Kuaiying veröffentlicht.
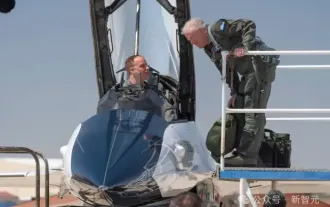
Kürzlich wurde die Militärwelt von der Nachricht überwältigt: US-Militärkampfflugzeuge können jetzt mithilfe von KI vollautomatische Luftkämpfe absolvieren. Ja, erst kürzlich wurde der KI-Kampfjet des US-Militärs zum ersten Mal der Öffentlichkeit zugänglich gemacht und sein Geheimnis gelüftet. Der vollständige Name dieses Jägers lautet „Variable Stability Simulator Test Aircraft“ (VISTA). Er wurde vom Minister der US-Luftwaffe persönlich geflogen, um einen Eins-gegen-eins-Luftkampf zu simulieren. Am 2. Mai startete US-Luftwaffenminister Frank Kendall mit einer X-62AVISTA auf der Edwards Air Force Base. Beachten Sie, dass während des einstündigen Fluges alle Flugaktionen autonom von der KI durchgeführt wurden! Kendall sagte: „In den letzten Jahrzehnten haben wir über das unbegrenzte Potenzial des autonomen Luft-Luft-Kampfes nachgedacht, aber es schien immer unerreichbar.“ Nun jedoch,
