解析一个通过添加本地分区索引提高SQL性能的案例
今天接到同事求助,说有一个select query,在Oracle上要跑一分多钟,他希望能在5s内出结果,以下就是解决这个问题的方法,需要的朋友可以参考下 该sql如下: 复制代码 代码如下: Select /*+ parallel(src, 8) */ distinct src.systemname as systemname ,
今天接到同事求助,说有一个select query,在Oracle上要跑一分多钟,他希望能在5s内出结果,以下就是解决这个问题的方法,需要的朋友可以参考下
该sql如下:
复制代码 代码如下:
Select /*+ parallel(src, 8) */ distinct
src.systemname as systemname
, src.databasename as databasename
, src.tablename as tablename
, src.username as username
from meta_dbql_table_usage_exp_hst src
inner join DR_QRY_LOG_EXP_HST rl on
src.acctstringdate = rl.acctstringdate
and src.queryid = rl.queryid
And Src.Systemname = Rl.Systemname
and src.acctstringdate > sysdate - 30
And Rl.Acctstringdate > Sysdate - 30
inner join meta_dr_qry_log_tgt_all_hst tgt on
upper(tgt.systemname) = upper('MOZART')
And Upper(tgt.Databasename) = Upper('GDW_TABLES')
And Upper(tgt.Tablename) = Upper('SSA_SLNG_LSTG_MTRC_SD')
AND src.acctstringdate = tgt.acctstringdate
and rl.statement_id = tgt.statement_id
and rl.systemname = tgt.systemname
And Tgt.Acctstringdate > Sysdate - 30
And Not(
Upper(Tgt.Systemname)=Upper(src.systemname)
And
Upper(Tgt.Databasename) = Upper(Src.Databasename)
And
Upper(Tgt.Tablename) = Upper(Src.Tablename)
)
And tgt.Systemname is not null
And tgt.Databasename Is Not Null
And tgt.tablename is not null
SQL的简单分析
总 得来看,这个SQL就是三个表 (meta_dbql_table_usage_exp_hst,DR_QRY_LOG_EXP_HST,meta_dr_qry_log_tgt_all_hst) 的INNER JOIN,这三个表数据量都在百万级别,且都是分区表(以acctstringdate为分区键),执行计划如下:
复制代码 代码如下:
------------------------------------------------------------------------------------------------------------------------
| Id | Operation | Name | Rows | Bytes | Cost | Pstart| Pstop |
------------------------------------------------------------------------------------------------------------------------
| 0 | SELECT STATEMENT | | 1 | 159 | 8654 | | |
| 1 | PX COORDINATOR | | | | | | |
| 2 | PX SEND QC (RANDOM) | :TQ10002 | 1 | 159 | 8654 | | |
| 3 | SORT UNIQUE | | 1 | 159 | 8654 | | |
| 4 | PX RECEIVE | | 1 | 36 | 3 | | |
| 5 | PX SEND HASH | :TQ10001 | 1 | 36 | 3 | | |
|* 6 | TABLE ACCESS BY LOCAL INDEX ROWID| DR_QRY_LOG_EXP_HST | 1 | 36 | 3 | | |
| 7 | NESTED LOOPS | | 1 | 159 | 8633 | | |
| 8 | NESTED LOOPS | | 8959 | 1076K| 4900 | | |
| 9 | BUFFER SORT | | | | | | |
| 10 | PX RECEIVE | | | | | | |
| 11 | PX SEND BROADCAST | :TQ10000 | | | | | |
| 12 | PARTITION RANGE ITERATOR | | 1 | 56 | 4746 | KEY | 14 |
|* 13 | TABLE ACCESS FULL | META_DR_QRY_LOG_TGT_ALL_HST | 1 | 56 | 4746 | KEY | 14 |
| 14 | PX BLOCK ITERATOR | | 8959 | 586K| 154 | KEY | KEY |
|* 15 | TABLE ACCESS FULL | META_DBQL_TABLE_USAGE_EXP_HST | 8959 | 586K| 154 | KEY | KEY |
| 16 | PARTITION RANGE ITERATOR | | 1 | | 2 | KEY | KEY |
|* 17 | INDEX RANGE SCAN | DR_QRY_LOG_EXP_HST_IDX | 1 | | 2 | KEY | KEY |
------------------------------------------------------------------------------------------------------------------------
Predicate Information (identified by operation id):
---------------------------------------------------
6 - filter("RL"."STATEMENT_ID"="TGT"."STATEMENT_ID" AND "RL"."SYSTEMNAME"="TGT"."SYSTEMNAME" AND "SRC"."SYSTEMNAME"="RL"."SYSTEMNAME")
13 - filter(UPPER("TGT"."SYSTEMNAME")='MOZART' AND UPPER("TGT"."DATABASENAME")='GDW_TABLES' AND
UPPER("TGT"."TABLENAME")='SSA_SLNG_LSTG_MTRC_SD' AND "TGT"."ACCTSTRINGDATE">SYSDATE@!-30 AND "TGT"."SYSTEMNAME" IS NOT NULL
"TGT"."DATABASENAME" IS NOT NULL AND "TGT"."TABLENAME" IS NOT NULL)
15 - filter("SRC"."ACCTSTRINGDATE"="TGT"."ACCTSTRINGDATE" AND (UPPER("TGT"."SYSTEMNAME")UPPER("SRC"."SYSTEMNAME") OR
UPPER("TGT"."DATABASENAME")UPPER("SRC"."DATABASENAME") OR UPPER("TGT"."TABLENAME")UPPER("SRC"."TABLENAME")) AND
"SRC"."ACCTSTRINGDATE">SYSDATE@!-30)
17 - access("SRC"."QUERYID"="RL"."QUERYID" AND "SRC"."ACCTSTRINGDATE"="RL"."ACCTSTRINGDATE")
filter("RL"."ACCTSTRINGDATE">SYSDATE@!-30)

Hot AI Tools

Undresser.AI Undress
AI-powered app for creating realistic nude photos

AI Clothes Remover
Online AI tool for removing clothes from photos.

Undress AI Tool
Undress images for free

Clothoff.io
AI clothes remover

Video Face Swap
Swap faces in any video effortlessly with our completely free AI face swap tool!

Hot Article

Hot Tools

Notepad++7.3.1
Easy-to-use and free code editor

SublimeText3 Chinese version
Chinese version, very easy to use

Zend Studio 13.0.1
Powerful PHP integrated development environment

Dreamweaver CS6
Visual web development tools

SublimeText3 Mac version
God-level code editing software (SublimeText3)

Hot Topics
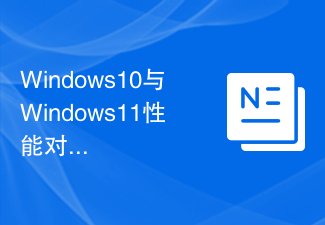
Windows 10 vs. Windows 11 performance comparison: Which one is better? With the continuous development and advancement of technology, operating systems are constantly updated and upgraded. As one of the world's largest operating system developers, Microsoft's Windows series of operating systems have always attracted much attention from users. In 2021, Microsoft released the Windows 11 operating system, which triggered widespread discussion and attention. So, what is the difference in performance between Windows 10 and Windows 11? Which
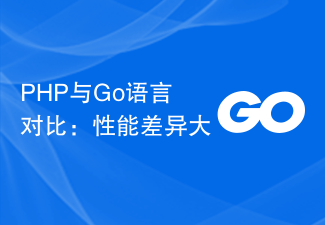
PHP and Go are two commonly used programming languages, and they have different characteristics and advantages. Among them, performance difference is an issue that everyone is generally concerned about. This article will compare PHP and Go languages from a performance perspective, and demonstrate their performance differences through specific code examples. First, let us briefly introduce the basic features of PHP and Go language. PHP is a scripting language originally designed for web development. It is easy to learn and use and is widely used in the field of web development. The Go language is a compiled language developed by Google.
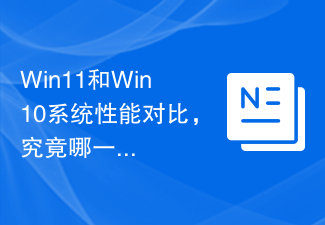
The Windows operating system has always been one of the most widely used operating systems on personal computers, and Windows 10 has long been Microsoft's flagship operating system until recently when Microsoft launched the new Windows 11 system. With the launch of Windows 11 system, people have become interested in the performance differences between Windows 10 and Windows 11 systems. Which one is better between the two? First, let’s take a look at W
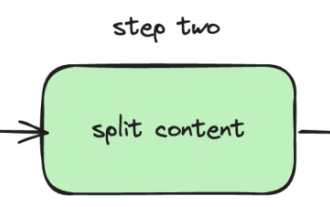
Ollama is a super practical tool that allows you to easily run open source models such as Llama2, Mistral, and Gemma locally. In this article, I will introduce how to use Ollama to vectorize text. If you have not installed Ollama locally, you can read this article. In this article we will use the nomic-embed-text[2] model. It is a text encoder that outperforms OpenAI text-embedding-ada-002 and text-embedding-3-small on short context and long context tasks. Start the nomic-embed-text service when you have successfully installed o
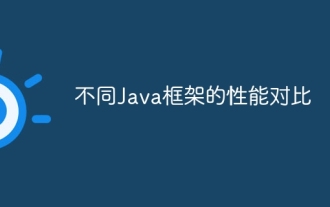
Performance comparison of different Java frameworks: REST API request processing: Vert.x is the best, with a request rate of 2 times SpringBoot and 3 times Dropwizard. Database query: SpringBoot's HibernateORM is better than Vert.x and Dropwizard's ORM. Caching operations: Vert.x's Hazelcast client is superior to SpringBoot and Dropwizard's caching mechanisms. Suitable framework: Choose according to application requirements. Vert.x is suitable for high-performance web services, SpringBoot is suitable for data-intensive applications, and Dropwizard is suitable for microservice architecture.
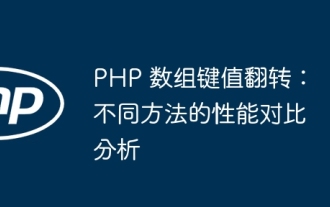
The performance comparison of PHP array key value flipping methods shows that the array_flip() function performs better than the for loop in large arrays (more than 1 million elements) and takes less time. The for loop method of manually flipping key values takes a relatively long time.
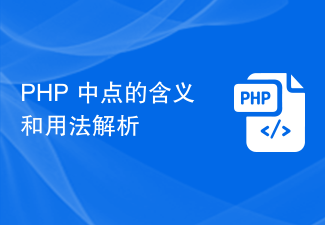
[Analysis of the meaning and usage of midpoint in PHP] In PHP, midpoint (.) is a commonly used operator used to connect two strings or properties or methods of objects. In this article, we’ll take a deep dive into the meaning and usage of midpoints in PHP, illustrating them with concrete code examples. 1. Connect string midpoint operator. The most common usage in PHP is to connect two strings. By placing . between two strings, you can splice them together to form a new string. $string1=&qu
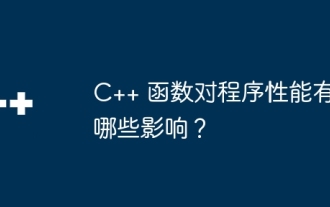
The impact of functions on C++ program performance includes function call overhead, local variable and object allocation overhead: Function call overhead: including stack frame allocation, parameter transfer and control transfer, which has a significant impact on small functions. Local variable and object allocation overhead: A large number of local variable or object creation and destruction can cause stack overflow and performance degradation.
