TI TPA2016D2 D类音频放大方案
欢迎进入IT技术社区论坛,与200万技术人员互动交流 >>进入 TI 公司的TPA2016D2 是立体声无滤波器的D类音频功率放大器,带有音量控制,动态范围压缩(DRC)和自动增益控制(AGC),5V工作时每路能向8欧姆负载1.7W的功率.器件具有独立的软件关断特性,并提供热保护和短
欢迎进入IT技术社区论坛,与200万技术人员互动交流 >>进入
TI 公司的TPA2016D2 是立体声无滤波器的D类音频功率放大器,带有音量控制,动态范围压缩(DRC)和自动增益控制(AGC),5V工作时每路能向8欧姆负载1.7W的功率.器件具有独立的软件关断特性,并提供热保护和短路保护,广泛应用在无线手机和PDA,手提导航设备,手提DVD播放器,笔记本电脑,收音机,游戏机以及智力玩具等.本文介绍了TPA2016D2的主要特性,功能方框图和应用电路以及TPA2016D2EVM评估板电路图和所用元件列表.The TPA2016D2 is a stereo, filter-free Class-D audio power amplifier with volume control, dynamic range compression (DRC) and automatic gain control (AGC). It is available in a 2.2 mm x 2.2 mm WCSP package.
The DRC/AGC function in the TPA2016D2 is programmable via a digital I2C interface. The DRC/AGC function can be configured to automatically prevent distortion of the audio signal and enhance quiet passages that are normally not heard. The DRC/AGC can also be configured to protect the speaker from damage at high power levels and compress the dynamic range of music to fit within the dynamic range of the speaker. The gain can be selected from -28 dB to +30 dB in 1-dB steps. The TPA2016D2 is capable of driving 1.7 W/Ch at 5 V or 750 mW/Ch at 3.6 V into 8 Ω load. The device features independent software shutdown controls for each channel and also provides thermal and short circuit protection.
图1. TPA2016D2外形图
TPA2016D2 主要特性:
Filter-Free Class-D Architecture
1.7 W/Ch Into 8 Ω at 5 V (10% THD+N)
750 mW/Ch Into 8 Ω at 3.6 V (10% THD+N)
Power Supply Range: 2.5 V to 5.5 V
Flexible Operation With/Without I2C
Programmable DRC/AGC Parameters
Digital I2C Volume Control
Selectable Gain from -28 dB to 30 dB in 1-dB Steps (when compression is used)
Selectable Attack, Release and Hold Times
4 Selectable Compression Ratios
Low Supply Current: 3.5 mA
Low Shutdown Current: 0.2 µA
High PSRR: 80 dB
Fast Start-up Time: 5 ms
AGC Enable/Disable Function
Limiter Enable/Disable Function
Short-Circuit and Thermal Protection
Space-Saving Package 2,2 mm × 2,2 mm Nano-Free WCSP (YZH)
应用范围:
Wireless or Cellular Handsets and PDAs
Portable Navigation Devices
Portable DVD Player
Notebook PCs
Portable Radio
Portable Games
Educational Toys
USB Speakers
图2. TPA2016D2功能方框图
图3. TPA2016D2简化应用电路
The TPA2016D2 evaluation module (EVM) is a complete, stand-alone audio board. It contains the TPA2016D2 WCSP (YZH) Class-D audio power amplifier.
图4. TPA2016D2EVM
[1] [2]

Hot AI Tools

Undresser.AI Undress
AI-powered app for creating realistic nude photos

AI Clothes Remover
Online AI tool for removing clothes from photos.

Undress AI Tool
Undress images for free

Clothoff.io
AI clothes remover

AI Hentai Generator
Generate AI Hentai for free.

Hot Article

Hot Tools

Notepad++7.3.1
Easy-to-use and free code editor

SublimeText3 Chinese version
Chinese version, very easy to use

Zend Studio 13.0.1
Powerful PHP integrated development environment

Dreamweaver CS6
Visual web development tools

SublimeText3 Mac version
God-level code editing software (SublimeText3)

Hot Topics


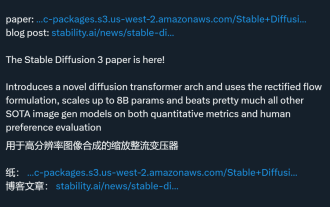
StableDiffusion3’s paper is finally here! This model was released two weeks ago and uses the same DiT (DiffusionTransformer) architecture as Sora. It caused quite a stir once it was released. Compared with the previous version, the quality of the images generated by StableDiffusion3 has been significantly improved. It now supports multi-theme prompts, and the text writing effect has also been improved, and garbled characters no longer appear. StabilityAI pointed out that StableDiffusion3 is a series of models with parameter sizes ranging from 800M to 8B. This parameter range means that the model can be run directly on many portable devices, significantly reducing the use of AI
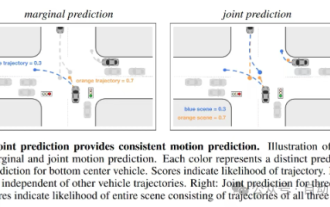
Trajectory prediction plays an important role in autonomous driving. Autonomous driving trajectory prediction refers to predicting the future driving trajectory of the vehicle by analyzing various data during the vehicle's driving process. As the core module of autonomous driving, the quality of trajectory prediction is crucial to downstream planning control. The trajectory prediction task has a rich technology stack and requires familiarity with autonomous driving dynamic/static perception, high-precision maps, lane lines, neural network architecture (CNN&GNN&Transformer) skills, etc. It is very difficult to get started! Many fans hope to get started with trajectory prediction as soon as possible and avoid pitfalls. Today I will take stock of some common problems and introductory learning methods for trajectory prediction! Introductory related knowledge 1. Are the preview papers in order? A: Look at the survey first, p
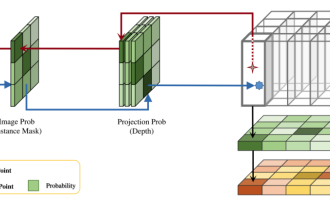
This paper explores the problem of accurately detecting objects from different viewing angles (such as perspective and bird's-eye view) in autonomous driving, especially how to effectively transform features from perspective (PV) to bird's-eye view (BEV) space. Transformation is implemented via the Visual Transformation (VT) module. Existing methods are broadly divided into two strategies: 2D to 3D and 3D to 2D conversion. 2D-to-3D methods improve dense 2D features by predicting depth probabilities, but the inherent uncertainty of depth predictions, especially in distant regions, may introduce inaccuracies. While 3D to 2D methods usually use 3D queries to sample 2D features and learn the attention weights of the correspondence between 3D and 2D features through a Transformer, which increases the computational and deployment time.
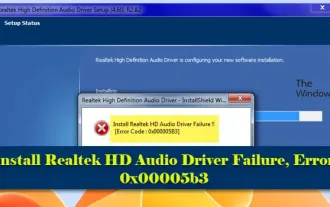
If you encounter RealtekHD audio driver failure error code 0x00005b3 on Windows 11/10 PC, please refer to the following steps to resolve the issue. We'll guide you through troubleshooting and resolving the error. Error code 0x00005b3 may be caused by audio driver installation issues. It may be that the current driver is corrupted or partially uninstalled, affecting the installation of the new driver. This issue can also be caused by insufficient disk space or an audio driver that is incompatible with your Windows version. Installation of RealtekHD audio driver failed! ! [Error Code: 0x00005B3] If there is a problem with the Realtek Audio Driver Installation Wizard, continue reading
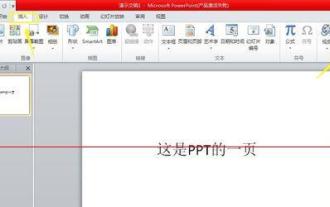
1. Open PPT, click [Insert] on the menu bar, and then click [Audio]. 2. In the file selection box that opens, select the first audio file you want to insert. 3. After the insertion is successful, a speaker icon will be displayed in the PPT to represent the file just inserted. You can play and listen to it and adjust the volume to control its volume during the PPT screening process. 4. Follow the same method and insert the second audio file. At this time, two speaker icons will be displayed in the PPT, representing two audio files respectively. 5. Click to select the first audio file icon, and then click the [Play] menu. 6. In Start in the toolbar, select [Play Across Slides] to set this audio to play in every slide without human intervention. 7. Follow the steps in step 6
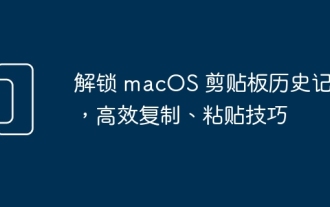
On Mac, it's common to need to copy and paste content between different documents. The macOS clipboard only retains the last copied item, which limits our work efficiency. Fortunately, there are some third-party applications that can help us view and manage our clipboard history easily. How to View Clipboard Contents in Finder There is a built-in clipboard viewer in Finder, allowing you to view the contents of the current clipboard at any time to avoid pasting errors. The operation is very simple: open the Finder, click the Edit menu, and then select Show Clipboard. Although the function of viewing the contents of the clipboard in the Finder is small, there are a few points to note: the clipboard viewer in the Finder can only display the contents and cannot edit them. If you copied
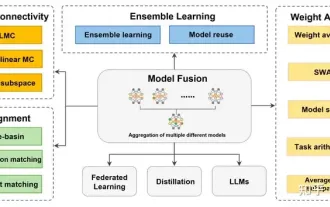
In September 23, the paper "DeepModelFusion:ASurvey" was published by the National University of Defense Technology, JD.com and Beijing Institute of Technology. Deep model fusion/merging is an emerging technology that combines the parameters or predictions of multiple deep learning models into a single model. It combines the capabilities of different models to compensate for the biases and errors of individual models for better performance. Deep model fusion on large-scale deep learning models (such as LLM and basic models) faces some challenges, including high computational cost, high-dimensional parameter space, interference between different heterogeneous models, etc. This article divides existing deep model fusion methods into four categories: (1) "Pattern connection", which connects solutions in the weight space through a loss-reducing path to obtain a better initial model fusion
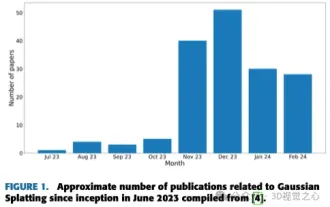
Written above & The author’s personal understanding is that image-based 3D reconstruction is a challenging task that involves inferring the 3D shape of an object or scene from a set of input images. Learning-based methods have attracted attention for their ability to directly estimate 3D shapes. This review paper focuses on state-of-the-art 3D reconstruction techniques, including generating novel, unseen views. An overview of recent developments in Gaussian splash methods is provided, including input types, model structures, output representations, and training strategies. Unresolved challenges and future directions are also discussed. Given the rapid progress in this field and the numerous opportunities to enhance 3D reconstruction methods, a thorough examination of the algorithm seems crucial. Therefore, this study provides a comprehensive overview of recent advances in Gaussian scattering. (Swipe your thumb up
