实例演示使用并行和非并行选项的性能差异
比较direct方式使用并行和非并行选项的性能差异。 并行测试 创建测试表 create table sqlldr_paral nologging PARTITION BY HASH (sfzh) (partition p1 tablespace users , partition p2tablespace zdry_tbs2 ) AS SELECT *FROM sfxx2 where 1=2 ; sqlldr_pa
比较direct方式使用并行和非并行选项的性能差异。
并行测试
创建测试表
create table sqlldr_paral
nologging
PARTITION BY HASH (sfzh)
(partition p1 tablespace users , partition p2tablespace zdry_tbs2 )
AS SELECT *FROM sfxx2 where 1=2 ;
sqlldr_paral.ctl
LOAD DATA
INFILE 'd:\flatfile\flatfile.dat'
APPEND
INTO TABLE sqlldr_paral
fields terminated by X'23'
TRAILING NULLCOLS
(zj,
…字段太多省略
cjdwdm)
sqlldr_paral.par
USERID=zdrygk/zdrygk
CONTROL='d:\flatfile\sqlldr_paral.ctl'
DATA='d:\flatfile\flatfile.dat'
LOG='d:\flatfile\sqlldr_paral.log'
DIRECT=true
parallel=true
ERRORS=10000
执行
D:\flatfile>sqlldrparfile=d:\flatfile\sqlldr_paral.par
SQL*Loader: Release 11.2.0.1.0 - Production onMon Jan 7 23:21:42 2013
Copyright (c) 1982, 2009, Oracle and/or itsaffiliates. All rights reserved.
Load completed - logical record count 3022375.
sqlldr_paral.log
Control File: d:\flatfile\sqlldr_paral.ctl
Data File: d:\flatfile\flatfile.dat
BadFile: d:\flatfile\flatfile.bad
DiscardFile: none specified
(Allowall discards)
Number to load: ALL
Number to skip: 0
Errors allowed: 10000
Continuation: none specified
Path used: Direct- with parallel option. -----这里指定了并行选项
Table SQLLDR_PARAL, loaded from every logicalrecord.
Insert option in effect for this table: APPEND
TRAILING NULLCOLS option in effect
Table SQLLDR_PARAL:
3017264Rows successfully loaded.
5111Rows not loaded due to data errors.
0 Rows not loaded because all WHEN clauseswere failed.
0 Rowsnot loaded because all fields were null.
Dateconversion cache disabled due to overflow (default size: 1000)
Partition P1: 1509091 Rows loaded.
Partition P2: 1508173 Rows loaded.
Bind array size not used in direct path.
Column array rows : 5000
Stream buffer bytes: 256000
Read buffer bytes: 1048576
Total logical records skipped: 0
Total logical records read: 3022375
Total logical records rejected: 5111
Total logical records discarded: 0
Total stream buffers loaded by SQL*Loader mainthread: 1301
Total stream buffers loaded by SQL*Loader loadthread: 3901
Run began on Mon Jan 07 23:21:42 2013
Run ended on Mon Jan 07 23:22:18 2013
Elapsed time was: 00:00:35.91
CPU time was: 00:00:25.79
非并行测试
创建测试表
create table sqlldr_nopal
nologging
PARTITION BY HASH (sfzh)
(partition p1 tablespace users , partition p2tablespace zdry_tbs2 )
AS SELECT *FROM sfxx2 where 1=2 ;
sqlldr_nopar.ctl
LOAD DATA
INFILE 'd:\flatfile\flatfile.dat'
APPEND
INTO TABLEsqlldr_nopar
fields terminated by X'23'
TRAILING NULLCOLS
(zj,
…字段太多省略
cjdwdm)
sqlldr_nopar.par
这里删除了并行选项
USERID=zdrygk/zdrygk
CONTROL='d:\flatfile\sqlldr_nopar.ctl'
DATA='d:\flatfile\flatfile.dat'
LOG='d:\flatfile\sqlldr_nopar.log'
DIRECT=true
ERRORS=10000
执行
D:\flatfile>sqlldrparfile=d:\flatfile\sqlldr_nopar.par
SQL*Loader: Release 11.2.0.1.0 - Production onMon Jan 7 23:20:54 2013
Copyright (c) 1982, 2009, Oracle and/or itsaffiliates. All rights reserved.
Load completed - logical record count 3022375.
sqlldr_nopar.log
Control File: d:\flatfile\sqlldr_nopar.ctl
Data File: d:\flatfile\flatfile.dat
BadFile: d:\flatfile\flatfile.bad
DiscardFile: none specified
(Allowall discards)
Number to load: ALL
Number to skip: 0
Errors allowed: 10000
Continuation: none specified
Path used: Direct ----这里只是指定了直接路径加载
Table SQLLDR_NOPAR, loaded from every logicalrecord.
Insert option in effect for this table: APPEND
TRAILING NULLCOLS option in effect
Table SQLLDR_NOPAR:
3017264Rows successfully loaded.
5111Rows not loaded due to data errors.
0 Rows not loaded because all WHEN clauseswere failed.
0 Rowsnot loaded because all fields were null.
Dateconversion cache disabled due to overflow (default size: 1000)
Partition P1: 1509091 Rows loaded.
Partition P2: 1508173 Rows loaded.
Bind array size not used in direct path.
Column array rows : 5000
Stream buffer bytes: 256000
Read buffer bytes: 1048576
Total logical records skipped: 0
Total logical records read: 3022375
Total logical records rejected: 5111
Total logical records discarded: 0
Total stream buffers loaded by SQL*Loader mainthread: 1301
Total stream buffers loaded by SQL*Loader loadthread: 3901
Run began on Mon Jan 07 23:20:54 2013
Run ended on Mon Jan 07 23:21:28 2013
Elapsed time was: 00:00:33.81
CPU time was: 00:00:25.32
分析
Load Mode |
Elapsed time(ss.99) |
Direct |
33.81 |
Direct + parallel |
35.91 |
笔者做了多次试验,除了第一次消耗时间较长(因为表空间自动拓展),后面的实验中两种模式的用时大致相同。自己猜测在使用Direct 直接路径加载的情况下,多线程并不能提高效率。
在Util中找到关于sql*loader command line的描述
PARALLEL(parallel load)
Default: false
PARALLELspecifies whether direct loads canoperate in multiple concurrent sessions
to load data into the same table.
parallel这个参数用来设定使用direct loads的时候是否使用并发的session去加载数据到相同的表中。
于是测试了多文件,多session加载数据的情况。
D:\flatfile>sqluldr2 user=zdrygk/zdrygk@orclquery=sfxx2 degree=8 file=d:\flatfile\ldrfiles%t.dat field=0x23 size=300MB
0rows exported at 2013-01-08 00:07:26, size 0 MB.
output file d:\flatfile\ldrfiles1357574846.dat closed at 707972 rows,size 304 MB.
output file d:\flatfile\ldrfiles1357574861.dat closed at 697498 rows,size 604 MB.
output file d:\flatfile\ldrfiles1357574876.dat closed at 701379 rows,size 904 MB.
output file d:\flatfile\ldrfiles1357574891.dat closed at 692556 rows,size 1204 MB.
222868rows exported at 2013-01-08 00:08:31, size 1300 MB.
output file d:\flatfile\ldrfiles1357574906.dat closed at 222868 rows,size 1300 MB.
一共5个文件。
多文件并行测试
如果指定了parallel选项,sqlldr运行的时候会对表加4级锁,允许多个sqlldr session同时对同一个表执行加载作用。在我们执行了truncate 操作后对表进行并行加载操作。
parallelpar1文件
USERID=zdrygk/zdrygk
CONTROL='D:\flatfile\multifile\paral\sqlldr_paral_1.ctl'
DATA='D:\flatfile\multifile\ldrfiles1357574846.dat'
LOG='D:\flatfile\multifile\paral\sqlldr_paral_1.log'
DIRECT=true
parallel=true
ERRORS=10000
parallel par2、3、4、5的内容大致相同。
在windows下只能打开多个窗口,通过复制粘贴的方法保证并行了。
执行
sqlldrparfile=D:\flatfile\multifile\paral\sqlldr_paral_1.par
sqlldrparfile=D:\flatfile\multifile\paral\sqlldr_paral_2.par
sqlldrparfile=D:\flatfile\multifile\paral\sqlldr_paral_3.par
sqlldr parfile=D:\flatfile\multifile\paral\sqlldr_paral_4.par
sqlldrparfile=D:\flatfile\multifile\paral\sqlldr_paral_5.par
最后得到了5个log文件关键内容:
log1
Run began on Tue Jan 08 01:51:11 2013
Run ended on Tue Jan 08 01:51:21 2013
Elapsed time was: 00:00:09.42
CPU time was: 00:00:06.43
…
中间的几个log文件省略
log5
Run began on Tue Jan 08 01:51:34 2013
Run ended on Tue Jan 08 01:51:38 2013
Elapsed time was: 00:00:03.27
CPU time was: 00:00:02.03
因为最后一个sqlldr session 是最后一个执行结束的,所以需要计算并行sqlldr的执行消耗时间,只需要使用log5中的结束时间减去log1中的开始时间即可。
01:51:38-01:51:11=22秒
因为不是使用程序执行这几个sqlldr程序,复制、粘贴、执行之间会有空隙。真实用时应该比22秒这个数字要小的多。
多文件非并行测试
因为如果没有指定parallel选项,sqlldr运行的时候会对表加6级锁,其他sqlldr session会无法工作,所以只能串行的执行sqlldr操作。在我们执行了truncate操作之后对表进行串行加载操作。
noparallelpar1文件
USERID=zdrygk/zdrygk
CONTROL='D:\flatfile\multifile\nopar\sqlldr_nopar_1.ctl'
DATA='D:\flatfile\multifile\ldrfiles1357574846.dat'
LOG='D:\flatfile\multifile\nopar\sqlldr_nopar_1.log'
DIRECT=true
ERRORS=10000
noparallel par2、3、4、5的内容大致相同。
执行
sqlldrparfile=D:\flatfile\multifile\nopar\sqlldr_nopar_1.par
sqlldr parfile=D:\flatfile\multifile\nopar\sqlldr_nopar_2.par
sqlldrparfile=D:\flatfile\multifile\nopar\sqlldr_nopar_3.par
sqlldrparfile=D:\flatfile\multifile\nopar\sqlldr_nopar_4.par
sqlldrparfile=D:\flatfile\multifile\nopar\sqlldr_nopar_5.par
最后得到了5个log文件关键内容:
log1
Run began on Tue Jan 08 01:47:35 2013
Run ended on Tue Jan 08 01:47:48 2013
Elapsed time was: 00:00:12.61
CPU time was: 00:00:06.92
…
中间的几个log文件省略
log5
Run began on Tue Jan 08 01:48:26 2013
Run ended on Tue Jan 08 01:48:30 2013
Elapsed time was: 00:00:03.68
CPU time was: 00:00:02.01
最后执行时间相加
12.61+ 12.54+ 12.09+ 11.40+ 03.68=52.32秒
总结
Load Mode |
Elapsed time(ss.99) |
Direct |
52.32 |
Direct + parallel |
22 |
sqlldr是cpu密集型的操作,并且多线程,只有在使用多个数据文件,并且开启多个sqlldr session的时候才会有效果。并且效果非常明显。在消耗更多cpu和内存的同时能够给予非常高的效率,在真实情况下与单文件加载相比效率相差有1倍以上。

Hot AI Tools

Undresser.AI Undress
AI-powered app for creating realistic nude photos

AI Clothes Remover
Online AI tool for removing clothes from photos.

Undress AI Tool
Undress images for free

Clothoff.io
AI clothes remover

AI Hentai Generator
Generate AI Hentai for free.

Hot Article

Hot Tools

Notepad++7.3.1
Easy-to-use and free code editor

SublimeText3 Chinese version
Chinese version, very easy to use

Zend Studio 13.0.1
Powerful PHP integrated development environment

Dreamweaver CS6
Visual web development tools

SublimeText3 Mac version
God-level code editing software (SublimeText3)

Hot Topics
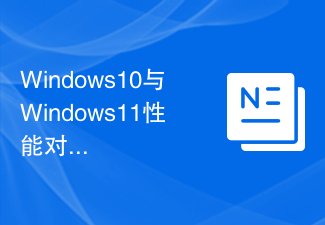
Windows 10 vs. Windows 11 performance comparison: Which one is better? With the continuous development and advancement of technology, operating systems are constantly updated and upgraded. As one of the world's largest operating system developers, Microsoft's Windows series of operating systems have always attracted much attention from users. In 2021, Microsoft released the Windows 11 operating system, which triggered widespread discussion and attention. So, what is the difference in performance between Windows 10 and Windows 11? Which
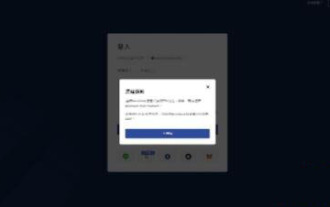
MetaMask (also called Little Fox Wallet in Chinese) is a free and well-received encryption wallet software. Currently, BTCC supports binding to the MetaMask wallet. After binding, you can use the MetaMask wallet to quickly log in, store value, buy coins, etc., and you can also get 20 USDT trial bonus for the first time binding. In the BTCCMetaMask wallet tutorial, we will introduce in detail how to register and use MetaMask, and how to bind and use the Little Fox wallet in BTCC. What is MetaMask wallet? With over 30 million users, MetaMask Little Fox Wallet is one of the most popular cryptocurrency wallets today. It is free to use and can be installed on the network as an extension
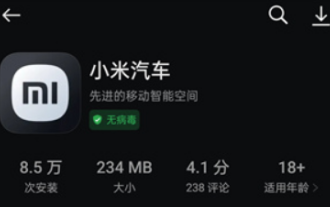
Xiaomi car software provides remote car control functions, allowing users to remotely control the vehicle through mobile phones or computers, such as opening and closing the vehicle's doors and windows, starting the engine, controlling the vehicle's air conditioner and audio, etc. The following is the use and content of this software, let's learn about it together . Comprehensive list of Xiaomi Auto app functions and usage methods 1. The Xiaomi Auto app was launched on the Apple AppStore on March 25, and can now be downloaded from the app store on Android phones; Car purchase: Learn about the core highlights and technical parameters of Xiaomi Auto, and make an appointment for a test drive. Configure and order your Xiaomi car, and support online processing of car pickup to-do items. 3. Community: Understand Xiaomi Auto brand information, exchange car experience, and share wonderful car life; 4. Car control: The mobile phone is the remote control, remote control, real-time security, easy
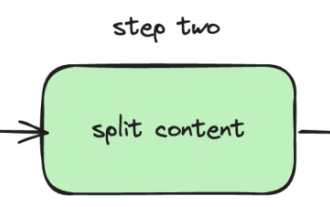
Ollama is a super practical tool that allows you to easily run open source models such as Llama2, Mistral, and Gemma locally. In this article, I will introduce how to use Ollama to vectorize text. If you have not installed Ollama locally, you can read this article. In this article we will use the nomic-embed-text[2] model. It is a text encoder that outperforms OpenAI text-embedding-ada-002 and text-embedding-3-small on short context and long context tasks. Start the nomic-embed-text service when you have successfully installed o
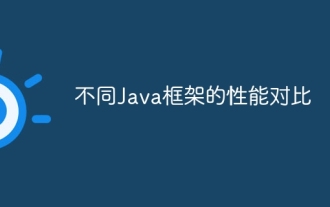
Performance comparison of different Java frameworks: REST API request processing: Vert.x is the best, with a request rate of 2 times SpringBoot and 3 times Dropwizard. Database query: SpringBoot's HibernateORM is better than Vert.x and Dropwizard's ORM. Caching operations: Vert.x's Hazelcast client is superior to SpringBoot and Dropwizard's caching mechanisms. Suitable framework: Choose according to application requirements. Vert.x is suitable for high-performance web services, SpringBoot is suitable for data-intensive applications, and Dropwizard is suitable for microservice architecture.
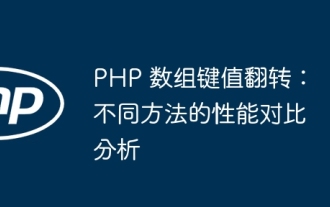
The performance comparison of PHP array key value flipping methods shows that the array_flip() function performs better than the for loop in large arrays (more than 1 million elements) and takes less time. The for loop method of manually flipping key values takes a relatively long time.
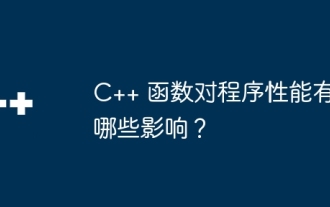
The impact of functions on C++ program performance includes function call overhead, local variable and object allocation overhead: Function call overhead: including stack frame allocation, parameter transfer and control transfer, which has a significant impact on small functions. Local variable and object allocation overhead: A large number of local variable or object creation and destruction can cause stack overflow and performance degradation.
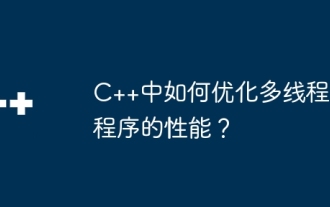
Effective techniques for optimizing C++ multi-threaded performance include limiting the number of threads to avoid resource contention. Use lightweight mutex locks to reduce contention. Optimize the scope of the lock and minimize the waiting time. Use lock-free data structures to improve concurrency. Avoid busy waiting and notify threads of resource availability through events.
