cvKMeans2均值聚类分析+代码解析+灰度彩色图像聚类
1 K-均聚类算法的基本思想 K-均聚类算法 是著名的划分聚类分割方法。划分方法的基本思想是:给定一个有N个元组或者纪录的数据集,分裂法将构造K个分组,每一个分组就代表一个聚类,KN。而且这K个分组满足下列条件:(1) 每一个分组至少包含一个数据纪录;(
1 K-均值聚类算法的基本思想
K-均值聚类算法是著名的划分聚类分割方法。划分方法的基本思想是:给定一个有N个元组或者纪录的数据集,分裂法将构造K个分组,每一个分组就代表一个聚类,K K-means算法的工作原理:算法首先随机从数据集中选取 K个点作为初始聚类中心,然后计算各个样本到聚类中心的距离,把样本归到离它最近的那个聚类中心所在的类。计算新形成的每一个聚类的数据对象的平均值来得到新的聚类中心,如果相邻两次的聚类中心没有任何变化,说明样本调整结束,聚类准则函数 已经收敛。本算法的一个特点是在每次迭代中都要考察每个样本的分类是否正确。若不正确,就要调整,在全部样本调整完后,再修改聚类中心,进入下一次迭代。这个过程将不断重复直到满足某个终止条件,终止条件可以是以下任何一个: (1)没有对象被重新分配给不同的聚类。 (2)聚类中心再发生变化。 (3)误差平方和局部最小。 K-means聚类算法的一般步骤: (1)从 n个数据对象任意选择 k 个对象作为初始聚类中心; (2)循环(3)到(4)直到每个聚类不再发生变化为止; (3)根据每个聚类对象的均值(中心对象),计算每个对象与这些中心对象的距离;并根据最小距离重新对相应对象进行划分; (4)重新计算每个(有变化)聚类的均值(中心对象),直到聚类中心不再变化。这种划分使得下式最小 K-均值聚类法的缺点: (1)在 K-means 算法中 K 是事先给定的,这个 K 值的选定是非常难以估计的。 (2)在 K-means 算法中,首先需要根据初始聚类中心来确定一个初始划分,然后对初始划分进行优化。 (3) K-means算法需要不断地进行样本分类调整不断地计算调整后的新的聚类中心因此当数据量非常大时算法的时间开销是非常大的。 (4)K-means算法对一些离散点和初始k值敏感,不同的距离初始值对同样的数据样本可能得到不同的结果。
2 OpenCV中K均值函数分析:
CV_IMPL int
cvKMeans2( const CvArr* _samples, intcluster_count, CvArr* _labels,
CvTermCriteria termcrit, int attempts, CvRNG*,
intflags, CvArr* _centers, double* _compactness )
_samples:输入样本的浮点矩阵,每个样本一行,如对彩色图像进行聚类,每个通道一行,CV_32FC3
cluster_count:所给定的聚类数目
_labels:输出整数向量:每个样本对应的类别标识,其范围为0- (cluster_count-1),必须满足以下条件:
cv::Mat data = cv::cvarrToMat(_samples),labels = cv::cvarrToMat(_labels);
CV_Assert(labels.isContinuous() && labels.type() == CV_32S &&
(labels.cols == 1 || labels.rows == 1)&&
labels.cols + labels.rows - 1 ==data.rows );
termcrit:指定聚类的最大迭代次数和/或精度(两次迭代引起的聚类中心的移动距离),其执行 k-means 算法搜索 cluster_count 个类别的中心并对样本进行分类,输出 labels(i) 为样本i的类别标识。其中CvTermCriteria为OpenCV中的迭代算法的终止准则,其结构如下:
#define CV_TERMCRIT_ITER 1
#define CV_TERMCRIT_NUMBER CV_TERMCRIT_ITER
#define CV_TERMCRIT_EPS 2
typedef struct CvTermCriteria
{
int type; int max_iter; double epsilon;
} CvTermCriteria;
max_iter:最大迭代次数。 epsilon:结果的精确性 。
attempts:
flags: 与labels和centers相关
_centers: 输出聚类中心,可以不用设置输出聚类中心,但如果想输出聚类中心必须满足以下条件:
CV_Assert(!centers.empty() );
CV_Assert( centers.rows == cluster_count );
CV_Assert( centers.cols ==data.cols );
CV_Assert( centers.depth() == data.depth() );
聚类中心的获得方式:(以三类为例)
double cent0 = centers->data.fl[0];
double cent1 = centers->data.fl[1];
double cent2 = centers->data.fl[2];
CV_IMPL int
cvKMeans2( const CvArr* _samples,int cluster_count,CvArr* _labels,
CvTermCriteriatermcrit, intattempts, CvRNG*,
int flags, CvArr* _centers, double* _compactness )
{
cv::Mat data = cv::cvarrToMat(_samples), labels= cv::cvarrToMat(_labels), centers;
if( _centers )
{
centers= cv::cvarrToMat(_centers);
// 将centers和data转换为行向量
centers= centers.reshape(1);
data= data.reshape(1);
// centers必须满足的条件
CV_Assert(!centers.empty());
CV_Assert(centers.rows== cluster_count );
CV_Assert(centers.cols== data.cols);
CV_Assert(centers.depth()== data.depth());
}
// labels必须满足的条件
CV_Assert(labels.isContinuous()&& labels.type()== CV_32S &&
(labels.cols == 1 || labels.rows == 1) &&
labels.cols + labels.rows - 1 == data.rows );
// 调用kmeans实现聚类,如果定义了输出聚类中心矩阵,那么输出centers
double compactness = cv::kmeans(data, cluster_count, labels,termcrit, attempts,
flags, _centers? cv::_OutputArray(centers) : cv::_OutputArray() );
if( _compactness )
*_compactness= compactness;
return 1;
}
double cv::kmeans( InputArray_data, int K,
InputOutputArray_bestLabels,
TermCriteriacriteria, intattempts,
intflags, OutputArray_centers )
{
const int SPP_TRIALS =3;
Mat data = _data.getMat();
// 判断data是否为行向量
bool isrow = data.rows == 1 && data.channels() > 1;
int N = !isrow ? data.rows : data.cols;
int dims = (!isrow? data.cols: 1)*data.channels();
int type = data.depth();
attempts= std::max(attempts, 1);
CV_Assert(data.dimstype == CV_32F && K> 0 );
CV_Assert(N >= K);
_bestLabels.create(N, 1, CV_32S, -1, true);
Mat _labels, best_labels = _bestLabels.getMat();
// 使用已初始化的labels
if( flags & CV_KMEANS_USE_INITIAL_LABELS)
{
CV_Assert((best_labels.cols== 1 || best_labels.rows== 1) &&
best_labels.cols*best_labels.rows == N&&
best_labels.type() == CV_32S&&
best_labels.isContinuous());
best_labels.copyTo(_labels);
}
else
{
if( !((best_labels.cols== 1 || best_labels.rows== 1) &&
best_labels.cols*best_labels.rows == N&&
best_labels.type() == CV_32S&&
best_labels.isContinuous()))
best_labels.create(N, 1, CV_32S);
_labels.create(best_labels.size(), best_labels.type());
}
int* labels = _labels.ptrint>();
Mat centers(K, dims, type), old_centers(K, dims, type), temp(1, dims, type);
vectorint> counters(K);
vectorVec2f> _box(dims);
Vec2f* box = &_box[0];
double best_compactness = DBL_MAX,compactness = 0;
RNG&rng = theRNG();
int a, iter, i, j, k;
// 对终止条件进行修改
if( criteria.type& TermCriteria::EPS)
criteria.epsilon = std::max(criteria.epsilon, 0.);
else
criteria.epsilon = FLT_EPSILON;
criteria.epsilon *= criteria.epsilon;
if( criteria.type& TermCriteria::COUNT)
criteria.maxCount = std::min(std::max(criteria.maxCount, 2), 100);
else
criteria.maxCount = 100;
// 聚类数目为1类的时候
if( K == 1 )
{
attempts= 1;
criteria.maxCount = 2;
}
const float* sample = data.ptrfloat>(0);
for( j = 0; j dims; j++ )
box[j] = Vec2f(sample[j], sample[j]);
for( i = 1; i N; i++ )
{
sample= data.ptrfloat>(i);
for( j = 0; j dims; j++ )
{
floatv = sample[j];
box[j][0] = std::min(box[j][0], v);
box[j][1] = std::max(box[j][1], v);
}
}
for( a = 0; a attempts; a++ )
{
double max_center_shift = DBL_MAX;
for( iter = 0;; )
{
swap(centers, old_centers);
/*enum
{
KMEANS_RANDOM_CENTERS=0, // Chooses random centers for k-Meansinitialization
KMEANS_PP_CENTERS=2, // Usesk-Means++ algorithm for initialization
KMEANS_USE_INITIAL_LABELS=1 // Uses the user-provided labels for K-Meansinitialization
};*/
if(iter == 0 && (a > 0 || !(flags& KMEANS_USE_INITIAL_LABELS)) )
{
if(flags & KMEANS_PP_CENTERS)
generateCentersPP(data, centers, K, rng, SPP_TRIALS);
else
{
for(k = 0; kK; k++)
generateRandomCenter(_box, centers.ptrfloat>(k), rng);
}
}
else
{
if(iter == 0 && a == 0 && (flags& KMEANS_USE_INITIAL_LABELS) )
{
for(i = 0; iN; i++)
CV_Assert( (unsigned)labels[i] unsigned)K);
}
//compute centers
centers= Scalar(0);
for(k = 0; kK; k++)
counters[k] = 0;
for(i = 0; iN; i++)
{
sample= data.ptrfloat>(i);
k= labels[i];
float*center = centers.ptrfloat>(k);
j=0;
#ifCV_ENABLE_UNROLLED
for(;j dims- 4; j += 4 )
{
float t0 = center[j] + sample[j];
float t1 = center[j+1] + sample[j+1];
center[j] = t0;
center[j+1] = t1;
t0 = center[j+2] + sample[j+2];
t1 = center[j+3] + sample[j+3];
center[j+2] = t0;
center[j+3] = t1;
}
#endif
for(; j dims;j++ )
center[j] += sample[j];
counters[k]++;
}
if(iter > 0 )
max_center_shift= 0;
for(k = 0; kK; k++)
{
if(counters[k]!= 0 )
continue;
// if somecluster appeared to be empty then:
// 1. find the biggest cluster
// 2. find the farthest from the center pointin the biggest cluster
// 3. exclude the farthest point from thebiggest cluster and form a new 1-point cluster.
intmax_k = 0;
for(int k1 = 1; k1 K; k1++ )
{
if( counters[max_k] counters[k1] )
max_k = k1;
}
doublemax_dist = 0;
intfarthest_i = -1;
float*new_center = centers.ptrfloat>(k);
float*old_center = centers.ptrfloat>(max_k);
float*_old_center = temp.ptrfloat>();// normalized
floatscale = 1.f/counters[max_k];
for(j = 0; jdims; j++)
_old_center[j] = old_center[j]*scale;
for(i = 0; iN; i++)
{
if(labels[i]!= max_k )
continue;
sample = data.ptrfloat>(i);
double dist = normL2Sqr_(sample,_old_center, dims);
if( max_dist dist )
{
max_dist = dist;
farthest_i = i;
}
}
counters[max_k]--;
counters[k]++;
labels[farthest_i] = k;
sample= data.ptrfloat>(farthest_i);
for(j = 0; jdims; j++)
{
old_center[j] -= sample[j];
new_center[j] += sample[j];
}
}
for(k = 0; kK; k++)
{
float*center = centers.ptrfloat>(k);
CV_Assert(counters[k]!= 0 );
floatscale = 1.f/counters[k];
for(j = 0; jdims; j++)
center[j] *= scale;
if(iter > 0 )
{
double dist = 0;
const float* old_center = old_centers.ptrfloat>(k);
for( j = 0; j dims; j++ )
{
double t = center[j] - old_center[j];
dist += t*t;
}
max_center_shift= std::max(max_center_shift, dist);
}
}
}
if(++iter == MAX(criteria.maxCount,2) || max_center_shift criteria.epsilon)
break;
// assignlabels
Matdists(1, N,CV_64F);
double*dist = dists.ptrdouble>(0);
parallel_for_(Range(0, N),
KMeansDistanceComputer(dist,labels, data,centers));
compactness= 0;
for(i = 0; iN; i++)
{
compactness+= dist[i];
}
}
if( compactness best_compactness)
{
best_compactness= compactness;
if(_centers.needed())
centers.copyTo(_centers);
_labels.copyTo(best_labels);
}
}
return best_compactness;
}
3 采用cvKMeans2对灰度图像进行聚类分析
//灰度图像聚类分析
BOOL GrayImageSegmentByKMeans2(const IplImage* pImg, IplImage*pResult, intsortFlag)
{
assert(pImg != NULL&& pImg->nChannels== 1);
//创建样本矩阵,CV_32FC1代表位浮点通道(灰度图像)
CvMat*samples = cvCreateMat((pImg->width)* (pImg->height),1, CV_32FC1);
//创建类别标记矩阵,CV_32SF1代表位整型通道
CvMat*clusters = cvCreateMat((pImg->width)* (pImg->height),1, CV_32SC1);
//创建类别中心矩阵
CvMat*centers = cvCreateMat(nClusters, 1, CV_32FC1);
// 将原始图像转换到样本矩阵
{
intk = 0;
CvScalars;
for(int i = 0; i pImg->width; i++)
{
for(int j=0;j pImg->height; j++)
{
s.val[0] = (float)cvGet2D(pImg, j, i).val[0];
cvSet2D(samples,k++, 0, s);
}
}
}
//开始聚类,迭代次,终止误差.0
cvKMeans2(samples, nClusters,clusters, cvTermCriteria(CV_TERMCRIT_ITER + CV_TERMCRIT_EPS,100, 1.0), 1, 0, 0, centers);
// 无需排序直接输出时
if (sortFlag == 0)
{
intk = 0;
intval = 0;
floatstep = 255 / ((float)nClusters - 1);
CvScalars;
for(int i = 0; i pImg->width; i++)
{
for(int j = 0;j pImg->height; j++)
{
val = (int)clusters->data.i[k++];
s.val[0] = 255- val * step;//这个是将不同类别取不同的像素值,
cvSet2D(pResult,j, i, s); //将每个像素点赋值
}
}
returnTRUE;
}
4 利用OpenCV对彩色图像进行颜色聚类:
BOOL ColorImageSegmentByKMeans2(const IplImage* img, IplImage* pResult, int sortFlag)
{
assert(img != NULL&& pResult != NULL);
assert(img->nChannels== 3 && pResult->nChannels == 1);
int i,j;
CvMat*samples=cvCreateMat((img->width)*(img->height),1,CV_32FC3);//创建样本矩阵,CV_32FC3代表位浮点通道(彩色图像)
CvMat*clusters=cvCreateMat((img->width)*(img->height),1,CV_32SC1);//创建类别标记矩阵,CV_32SF1代表位整型通道
int k=0;
for (i=0;iimg->width;i++)
{
for(j=0;jimg->height;j++)
{
CvScalars;
//获取图像各个像素点的三通道值(RGB)
s.val[0]=(float)cvGet2D(img,j,i).val[0];
s.val[1]=(float)cvGet2D(img,j,i).val[1];
s.val[2]=(float)cvGet2D(img,j,i).val[2];
cvSet2D(samples,k++,0,s);//将像素点三通道的值按顺序排入样本矩阵
}
}
cvKMeans2(samples,nClusters,clusters,cvTermCriteria(CV_TERMCRIT_ITER,100,1.0));//开始聚类,迭代次,终止误差.0
k=0;
int val=0;
float step=255/(nClusters-1);
for (i=0;iimg->width;i++)
{
for(j=0;jimg->height;j++)
{
val=(int)clusters->data.i[k++];
CvScalars;
s.val[0]=255-val*step;//这个是将不同类别取不同的像素值,
cvSet2D(pResult,j,i,s); //将每个像素点赋值
}
}
cvReleaseMat(&samples);
cvReleaseMat(&clusters);
return TRUE;
}

Hot AI Tools

Undresser.AI Undress
AI-powered app for creating realistic nude photos

AI Clothes Remover
Online AI tool for removing clothes from photos.

Undress AI Tool
Undress images for free

Clothoff.io
AI clothes remover

AI Hentai Generator
Generate AI Hentai for free.

Hot Article

Hot Tools

Notepad++7.3.1
Easy-to-use and free code editor

SublimeText3 Chinese version
Chinese version, very easy to use

Zend Studio 13.0.1
Powerful PHP integrated development environment

Dreamweaver CS6
Visual web development tools

SublimeText3 Mac version
God-level code editing software (SublimeText3)

Hot Topics
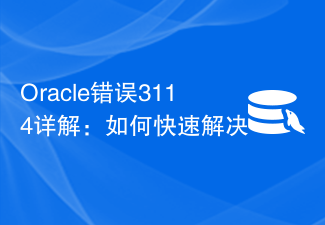
Detailed explanation of Oracle error 3114: How to solve it quickly, specific code examples are needed. During the development and management of Oracle database, we often encounter various errors, among which error 3114 is a relatively common problem. Error 3114 usually indicates a problem with the database connection, which may be caused by network failure, database service stop, or incorrect connection string settings. This article will explain in detail the cause of error 3114 and how to quickly solve this problem, and attach the specific code
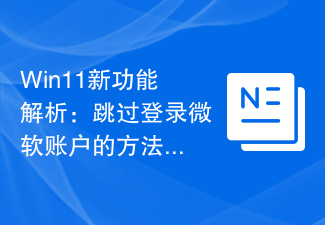
Analysis of new features of Win11: How to skip logging in to a Microsoft account. With the release of Windows 11, many users have found that it brings more convenience and new features. However, some users may not like having their system tied to a Microsoft account and wish to skip this step. This article will introduce some methods to help users skip logging in to a Microsoft account in Windows 11 and achieve a more private and autonomous experience. First, let’s understand why some users are reluctant to log in to their Microsoft account. On the one hand, some users worry that they
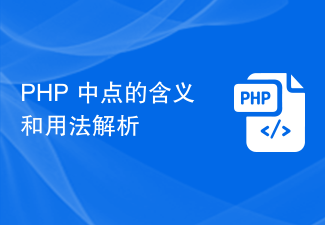
[Analysis of the meaning and usage of midpoint in PHP] In PHP, midpoint (.) is a commonly used operator used to connect two strings or properties or methods of objects. In this article, we’ll take a deep dive into the meaning and usage of midpoints in PHP, illustrating them with concrete code examples. 1. Connect string midpoint operator. The most common usage in PHP is to connect two strings. By placing . between two strings, you can splice them together to form a new string. $string1=&qu

Working with files in the Linux operating system requires the use of various commands and techniques that enable developers to efficiently create and execute files, code, programs, scripts, and other things. In the Linux environment, files with the extension ".a" have great importance as static libraries. These libraries play an important role in software development, allowing developers to efficiently manage and share common functionality across multiple programs. For effective software development in a Linux environment, it is crucial to understand how to create and run ".a" files. This article will introduce how to comprehensively install and configure the Linux ".a" file. Let's explore the definition, purpose, structure, and methods of creating and executing the Linux ".a" file. What is L
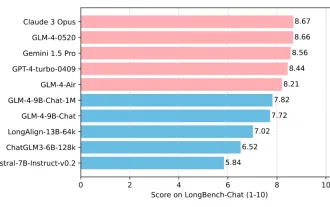
Since the launch of ChatGLM-6B on March 14, 2023, the GLM series models have received widespread attention and recognition. Especially after ChatGLM3-6B was open sourced, developers are full of expectations for the fourth-generation model launched by Zhipu AI. This expectation has finally been fully satisfied with the release of GLM-4-9B. The birth of GLM-4-9B In order to give small models (10B and below) more powerful capabilities, the GLM technical team launched this new fourth-generation GLM series open source model: GLM-4-9B after nearly half a year of exploration. This model greatly compresses the model size while ensuring accuracy, and has faster inference speed and higher efficiency. The GLM technical team’s exploration has not
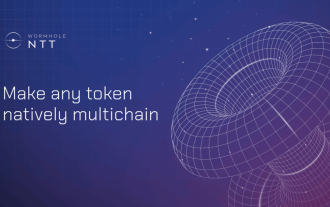
Wormhole is a leader in blockchain interoperability, focused on creating resilient, future-proof decentralized systems that prioritize ownership, control, and permissionless innovation. The foundation of this vision is a commitment to technical expertise, ethical principles, and community alignment to redefine the interoperability landscape with simplicity, clarity, and a broad suite of multi-chain solutions. With the rise of zero-knowledge proofs, scaling solutions, and feature-rich token standards, blockchains are becoming more powerful and interoperability is becoming increasingly important. In this innovative application environment, novel governance systems and practical capabilities bring unprecedented opportunities to assets across the network. Protocol builders are now grappling with how to operate in this emerging multi-chain
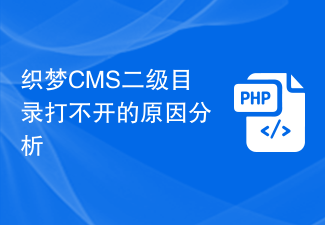
Title: Analysis of the reasons and solutions for why the secondary directory of DreamWeaver CMS cannot be opened. Dreamweaver CMS (DedeCMS) is a powerful open source content management system that is widely used in the construction of various websites. However, sometimes during the process of building a website, you may encounter a situation where the secondary directory cannot be opened, which brings trouble to the normal operation of the website. In this article, we will analyze the possible reasons why the secondary directory cannot be opened and provide specific code examples to solve this problem. 1. Possible cause analysis: Pseudo-static rule configuration problem: during use
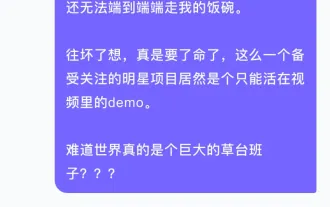
The big model subverts everything, and finally got to the head of this editor. It is also an Agent that was created in just one sentence. Like this, give him an article, and in less than 1 second, fresh title suggestions will come out. Compared to me, this efficiency can only be said to be as fast as lightning and as slow as a sloth... What's even more incredible is that creating this Agent really only takes a few minutes. Prompt belongs to Aunt Jiang: And if you also want to experience this subversive feeling, now, based on the new Wenxin intelligent agent platform launched by Baidu, everyone can create their own intelligent assistant for free. You can use search engines, smart hardware platforms, speech recognition, maps, cars and other Baidu mobile ecological channels to let more people use your creativity! Robin Li himself
