优化案例:缺少整体规划导致DB性能问题
最近几天对客户的一个核心数据库进行了优化,将资源消耗较高的SQL优化完成之后,物理读和逻辑读总量得到了降低。客户反馈优化后性能有提升,但仍然在某些工作日的业务高峰时段存在性能问题。 我们通过将性能不佳的业务高峰时段(即问题时段)与性能正常的业务
最近几天对客户的一个核心数据库进行了优化,将资源消耗较高的SQL优化完成之后,物理读和逻辑读总量得到了降低。客户反馈优化后性能有提升,但仍然在某些工作日的业务高峰时段存在性能问题。我们通过将性能不佳的业务高峰时段(即问题时段)与性能正常的业务高峰时段(即基线时段)的性能数据进行了对比,发现了一些问题:
基线时段为2014-1-15日上午8:00-上午9:00,此时段TPS(每秒事务量)为:46T/s,该时段的总DB Time为:626.2 (mins)
问题时段为2014-1-20日上午8:00-上午9:00,此时段TPS为:47T/s(仅比基线时段多1T/s,可认为两者业务量相当),该时段的总DB Time为2361.4 (mins)
同样均为1小时的取样时间段,问题段的总DB Time是基线的近4倍,而通过对比两者的性能视图,发现问题时段的单次IO延迟非常高,如下:
Event Waits Time(s) Avg wait (ms) % DB time Wait Class
DB CPU 2,082 55.42
db file sequential read 62,140 774 12 20.61 User I/O
direct path read 177,440 575 3 15.31 User I/O
log file sync 17,486 145 8 3.86 Commit
gc cr block 2-way 98,519 30 0 0.80 Cluster
基线时段单次序列读延时为12ms,单次直接读延时为3ms,单次redolog写延时为8ms,
Event Waits Time(s) Avg wait (ms) % DB time Wait Class
direct path read 180,200 4,643 26 32.77 User I/O
db file sequential read 55,483 2,286 41 16.13 User I/O
DB CPU 1,917 13.53
gc buffer busy acquire 5,513 1,474 267 10.40 Cluster
log file sync 17,541 1,298 74 9.16 Commit
而问题时段单次序列读延时为41ms,单次直接读延时为26ms,单次redolog写延时为74ms
(Oracle文档中建议的单次IO正常延时应为0-20ms,否则需升级硬件),
即相比基线时段,在业务量不变的情况下,问题时段的IO效率下降非常明显,怀疑是存储层面的同一个RAID组中有其他业务系统有可能恰好在问题时段有大量的IO操作,
导致我们正在优化的系统的IO延迟较大。跟客户的存储人员确认发现确实如此,存储人员并没有结合数据库对存储做出合理规划,仅仅从容量管理上对自己工作的方便性出发,划分并分配LUN。由此导致性能问题,我想这种问题在很多企业都是存在的,跨部门之间的沟通不畅导致没有从整体上的规划出现,最终出现问题由DB买单。
因此建议客户进行存储改善:
1.将这种关键系统在存储层面与其他系统隔离,避免互相影响IO;
2.有预算的情况下升级存储。

Hot AI Tools

Undresser.AI Undress
AI-powered app for creating realistic nude photos

AI Clothes Remover
Online AI tool for removing clothes from photos.

Undress AI Tool
Undress images for free

Clothoff.io
AI clothes remover

Video Face Swap
Swap faces in any video effortlessly with our completely free AI face swap tool!

Hot Article

Hot Tools

Notepad++7.3.1
Easy-to-use and free code editor

SublimeText3 Chinese version
Chinese version, very easy to use

Zend Studio 13.0.1
Powerful PHP integrated development environment

Dreamweaver CS6
Visual web development tools

SublimeText3 Mac version
God-level code editing software (SublimeText3)

Hot Topics
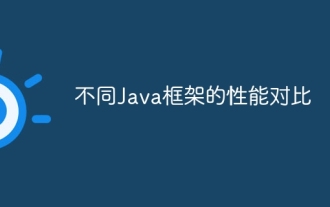
Performance comparison of different Java frameworks: REST API request processing: Vert.x is the best, with a request rate of 2 times SpringBoot and 3 times Dropwizard. Database query: SpringBoot's HibernateORM is better than Vert.x and Dropwizard's ORM. Caching operations: Vert.x's Hazelcast client is superior to SpringBoot and Dropwizard's caching mechanisms. Suitable framework: Choose according to application requirements. Vert.x is suitable for high-performance web services, SpringBoot is suitable for data-intensive applications, and Dropwizard is suitable for microservice architecture.
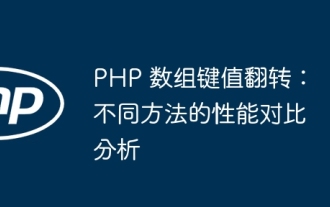
The performance comparison of PHP array key value flipping methods shows that the array_flip() function performs better than the for loop in large arrays (more than 1 million elements) and takes less time. The for loop method of manually flipping key values takes a relatively long time.
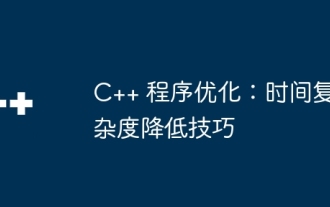
Time complexity measures the execution time of an algorithm relative to the size of the input. Tips for reducing the time complexity of C++ programs include: choosing appropriate containers (such as vector, list) to optimize data storage and management. Utilize efficient algorithms such as quick sort to reduce computation time. Eliminate multiple operations to reduce double counting. Use conditional branches to avoid unnecessary calculations. Optimize linear search by using faster algorithms such as binary search.
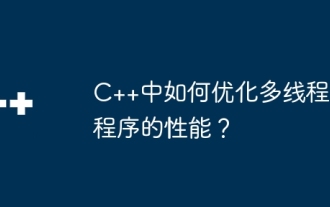
Effective techniques for optimizing C++ multi-threaded performance include limiting the number of threads to avoid resource contention. Use lightweight mutex locks to reduce contention. Optimize the scope of the lock and minimize the waiting time. Use lock-free data structures to improve concurrency. Avoid busy waiting and notify threads of resource availability through events.
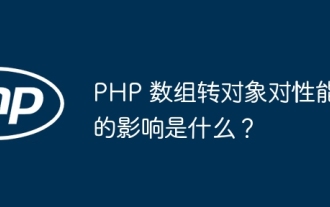
In PHP, the conversion of arrays to objects will have an impact on performance, mainly affected by factors such as array size, complexity, object class, etc. To optimize performance, consider using custom iterators, avoiding unnecessary conversions, batch converting arrays, and other techniques.
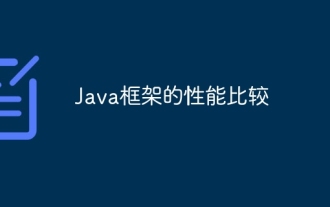
According to benchmarks, for small, high-performance applications, Quarkus (fast startup, low memory) or Micronaut (TechEmpower excellent) are ideal choices. SpringBoot is suitable for large, full-stack applications, but has slightly slower startup times and memory usage.
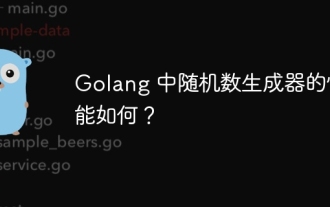
The best way to generate random numbers in Go depends on the level of security required by your application. Low security: Use the math/rand package to generate pseudo-random numbers, suitable for most applications. High security: Use the crypto/rand package to generate cryptographically secure random bytes, suitable for applications that require stronger randomness.
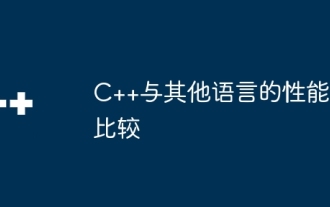
When developing high-performance applications, C++ outperforms other languages, especially in micro-benchmarks. In macro benchmarks, the convenience and optimization mechanisms of other languages such as Java and C# may perform better. In practical cases, C++ performs well in image processing, numerical calculations and game development, and its direct control of memory management and hardware access brings obvious performance advantages.
