NikitaIvanov谈GridGain的Hadoop内存片内加速技术
GridGain最近在2014年的Spark峰会上发布了Hadoop内存片内加速技术,可以为Hadoop应用带来内存片内计算的相关收益。 该技术包括两个单元:和Hadoop HDFS兼容的内存片内文件系统,以及为内存片内处理而优化的MapReduce实现。这两个单元对基于磁盘的HDFS和传统
GridGain最近在2014年的Spark峰会上发布了Hadoop内存片内加速技术,可以为Hadoop应用带来内存片内计算的相关收益。
该技术包括两个单元:和Hadoop HDFS兼容的内存片内文件系统,以及为内存片内处理而优化的MapReduce实现。这两个单元对基于磁盘的HDFS和传统的MapReduce进行了扩展,为大数据处理情况提供了更好的性能。
内存片内加速技术消除了在传统Hadoop架构模型中与作业追踪者(job tracker)、任务追踪者(task tracker)相关的系统开销,它可以和现有的MapReduce应用一起工作而无需改动任何原有的MapReduce、HDFS和YARN环境的代码。
下面是InfoQ对GridGain的CTO Nikita Ivanov关于Hadoop内存片内加速技术和架构细节的访谈。
InfoQ: Hadoop内存片内加速技术的关键特性在于GridGain的内存片内文件系统和内存片内MapReduce,你能描述一下这两个组件是如何协同工作的吗?
Nikita:GridGain的Hadoop内存片内加速技术是一种免费、开源和即插即用的解决方案,它提升了传统MapReduce工作(MapReduce jobs)的速度,你只需用10分钟进行下载和安装,就可以得到十几倍的性能提升,并且不需要对代码做任何改动。该产品是业界第一个基于双模、高性能内存片内文件系统,以及为内存片内处理而优化的MapReduce实现方案,这个文件系统和Hadoop的HDFS百分百的兼容。内存片内HDFS和内存片内MapReduce以易用的方式对基于磁盘的HDFS和传统的MapReduce进行了扩展,以带来显著的性能提升。
简要地说,GridGain的内存片内文件系统GGFS提供了一个高性能、分布式并与HDFS兼容的内存片内计算平台,并在此进行数据的存储,这样我们基于YARN的MapReduce实现就可以在数据存储这块利用GGFS做针对性的优化。这两个组件都是必需的,这样才能达到十几倍的性能提升(在一些边界情况下可以更高)。
InfoQ: 如何对这两种组合做一下比较,一种是内存片内HDFS和内存片内MapReduce的组合,另一种是基于磁盘的HDFS和传统的MapReduce的组合?
Nikita:GridGain的内存片内方案和传统的HDFS/MapReduce方案最大的不同在于:
在GridGain的内存片内计算平台里,数据是以分布式的方式存储在内存中。GridGain的MapReduce实现是从底层向上优化,以充分利用数据存储在内存中这一优势,同时改善了Hadoop之前架构中的一些缺陷。在GridGain的MapReduce实现中,执行路径是从客户端应用的工作提交者(job submitter)直接到数据节点,然后完成进程内(in-process)的数据处理,数据处理是基于数据节点中的内存片内数据分区,这样就绕过了传统实现中的作业跟踪者(job tracker)、任务跟踪者(task tracker)和名字节点(name nodes)这些单元,也避免了相关的延迟。
相比而言,传统的MapReduce实现中,数据是存储在低速的磁盘上,而MapReduce实现也是基于此而做优化的。
InfoQ:你能描述一下这个在Hadoop内存片内加速技术背后的双模、高性能的内存片内文件系统是如何工作的?它与传统的文件系统又有何不同呢?
Nikita:GridGain的内存片内文件系统GGFS支持两种模式,一种模式是作为独立的Hadoop簇的主文件系统,另一种模式是和HDFS进行串联,此时GGFS作为主文件系统HDFS的智能缓存层。
作为缓存层,GGFS可以提供直接读和直接写的逻辑,这些逻辑是高度可调节的,并且用户也可以自由地选择哪些文件和目录要被缓存以及如何缓存。这两种情况下,GGFS可以作为对传统HDFS的嵌入式替代方案,或者是一种扩展,而这都会立刻带来性能的提升。
InfoQ:如何比较GridGain的内存片内MapReduce方案和其它的一些实时流解决方案,比如Storm或者Apache Spark?
Nikita:最本质的差别在于GridGain的内存片内加速技术支持即插即用这一特性。不同于Storm或者Spark(顺便说一下,两者都是伟大的项目),它们需要对你原有的Hadoop MapReduce代码进行完全的推倒重来,而GridGain不需要修改一行代码,就能得到相同甚至更高的性能优势。
InfoQ:什么情况下需要使用Hadoop内存片内加速技术呢?
Nikita:实际上当你听到“实时分析”这个词时,也就听到了Hadoop内存片内加速技术的新用例。如你所知,在传统的Hadoop中并没有实时的东西。我们在新兴的HTAP (hybrid transactional and analytical processing)中正看到一些这样的用例,比如欺诈保护,游戏中分析,算法交易,投资组合分析和优化等等。
InfoQ:你能谈谈GridGain的Visor和基于图形界面的文件系统分析工具吗,以及他们如何帮助监视和管理Hadoop工作(Hadoop jobs)的?
Nikita:GridGain的Hadoop内存片内加速是和GridGain的Visor合在一起的,Visor是一种对GridGain产品进行管理和监视的方案。Visor提供了对Hadoop内存片内加速技术的直接支持,它为HDFS兼容的文件系统提供了精细的文件管理器和HDFS分析工具,通过它你可以看到并分析和HDFS相关的各种实时性能信息。
InfoQ:后面的产品路标是怎么样的呢?
Nikita:我们会持续投资(同我们的开源社区一起)来为Hadoop相关产品技术,包括Hive、Pig和Hbase,提供性能提升方案。
Taneja Group也有相关报道(Memory is the Hidden Secret to Success with Big Data, 下载全部报告需要先注册),讨论了GridGain如何把Hadoop内存片内加速技术和已有的Hadoop簇、传统基于磁盘的有缺陷的数据库系统以及面向批处理的MapReduce技术进行集成。
关于被访问者
Nikita Ivanov是GridGain系统公司的发起人和CTO,GridGain成立于2007年,投资者包括RTP Ventures和Almaz Capital。Nikita领导GridGain开发了领先的分布式内存片内数据处理技术-领先的Java内存片内计算平台,今天在全世界每10秒它就会启动运行一次。Nikita有超过20年的软件应用开发经验,创建了HPC和中间件平台,并在一些创业公司和知名企业都做出过贡献,包括Adaptec, Visa和BEA Systems。Nikita也是使用Java技术作为服务器端开发应用的先驱者,1996年他在为欧洲大型系统做集成工作时他就进行了相关实践。
查看参考原文:Nikita Ivanov on GridGain’s In-Memory Accelerator for Hadoop

Hot AI Tools

Undresser.AI Undress
AI-powered app for creating realistic nude photos

AI Clothes Remover
Online AI tool for removing clothes from photos.

Undress AI Tool
Undress images for free

Clothoff.io
AI clothes remover

AI Hentai Generator
Generate AI Hentai for free.

Hot Article

Hot Tools

Notepad++7.3.1
Easy-to-use and free code editor

SublimeText3 Chinese version
Chinese version, very easy to use

Zend Studio 13.0.1
Powerful PHP integrated development environment

Dreamweaver CS6
Visual web development tools

SublimeText3 Mac version
God-level code editing software (SublimeText3)

Hot Topics


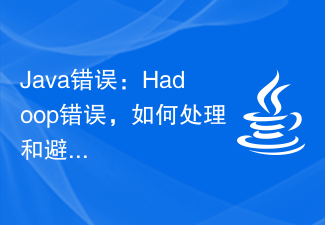
Java Errors: Hadoop Errors, How to Handle and Avoid When using Hadoop to process big data, you often encounter some Java exception errors, which may affect the execution of tasks and cause data processing to fail. This article will introduce some common Hadoop errors and provide ways to deal with and avoid them. Java.lang.OutOfMemoryErrorOutOfMemoryError is an error caused by insufficient memory of the Java virtual machine. When Hadoop is
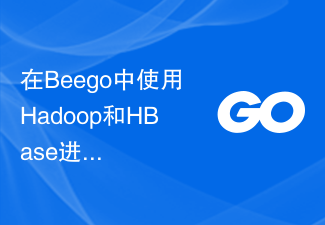
With the advent of the big data era, data processing and storage have become more and more important, and how to efficiently manage and analyze large amounts of data has become a challenge for enterprises. Hadoop and HBase, two projects of the Apache Foundation, provide a solution for big data storage and analysis. This article will introduce how to use Hadoop and HBase in Beego for big data storage and query. 1. Introduction to Hadoop and HBase Hadoop is an open source distributed storage and computing system that can
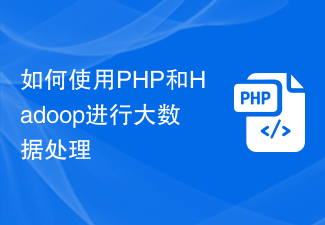
As the amount of data continues to increase, traditional data processing methods can no longer handle the challenges brought by the big data era. Hadoop is an open source distributed computing framework that solves the performance bottleneck problem caused by single-node servers in big data processing through distributed storage and processing of large amounts of data. PHP is a scripting language that is widely used in web development and has the advantages of rapid development and easy maintenance. This article will introduce how to use PHP and Hadoop for big data processing. What is HadoopHadoop is
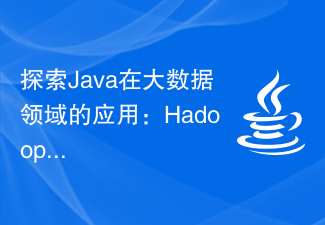
Java big data technology stack: Understand the application of Java in the field of big data, such as Hadoop, Spark, Kafka, etc. As the amount of data continues to increase, big data technology has become a hot topic in today's Internet era. In the field of big data, we often hear the names of Hadoop, Spark, Kafka and other technologies. These technologies play a vital role, and Java, as a widely used programming language, also plays a huge role in the field of big data. This article will focus on the application of Java in large
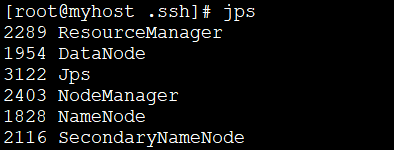
1: Install JDK1. Execute the following command to download the JDK1.8 installation package. wget--no-check-certificatehttps://repo.huaweicloud.com/java/jdk/8u151-b12/jdk-8u151-linux-x64.tar.gz2. Execute the following command to decompress the downloaded JDK1.8 installation package. tar-zxvfjdk-8u151-linux-x64.tar.gz3. Move and rename the JDK package. mvjdk1.8.0_151//usr/java84. Configure Java environment variables. echo'
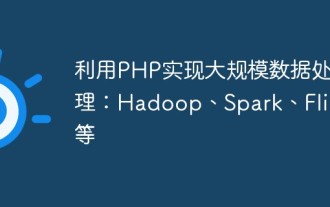
As the amount of data continues to increase, large-scale data processing has become a problem that enterprises must face and solve. Traditional relational databases can no longer meet this demand. For the storage and analysis of large-scale data, distributed computing platforms such as Hadoop, Spark, and Flink have become the best choices. In the selection process of data processing tools, PHP is becoming more and more popular among developers as a language that is easy to develop and maintain. In this article, we will explore how to leverage PHP for large-scale data processing and how
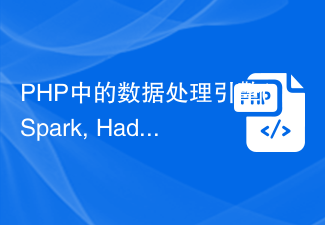
In the current Internet era, the processing of massive data is a problem that every enterprise and institution needs to face. As a widely used programming language, PHP also needs to keep up with the times in data processing. In order to process massive data more efficiently, PHP development has introduced some big data processing tools, such as Spark and Hadoop. Spark is an open source data processing engine that can be used for distributed processing of large data sets. The biggest feature of Spark is its fast data processing speed and efficient data storage.
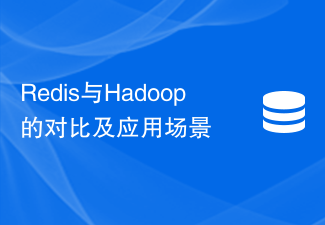
Redis and Hadoop are both commonly used distributed data storage and processing systems. However, there are obvious differences between the two in terms of design, performance, usage scenarios, etc. In this article, we will compare the differences between Redis and Hadoop in detail and explore their applicable scenarios. Redis Overview Redis is an open source memory-based data storage system that supports multiple data structures and efficient read and write operations. The main features of Redis include: Memory storage: Redis
