BigData-09-Greenplum概述及架构
0.写在前面: 0.1. 此笔记是参考《Greenplum企业应用实战》、《PostgreSQL8.2.3 中文文档》和《Getting Started with Greenplum for Big Data Analytics》整理; 0.2. 《Greenplum企业应用实战》购买地址:【京东商城】 【 当当网】 0.3.参考网页(持续更新)
0.写在前面:
0.1. 此笔记是参考《Greenplum企业应用实战》、《PostgreSQL8.2.3 中文文档》和《Getting Started with Greenplum for Big Data Analytics》整理;
0.2. 《Greenplum企业应用实战》购买地址:【京东商城】 【 当当网】
0.3.参考网页(持续更新)
1) Shared Disk VS Shared Nothing分布式架构1. Greenplum概述及架构
1.1. 什么是Greenplum
1) 为全球大型企业用户提供新型企业级数据仓库(EDW)、企业级数据云(EDC)和商务智能(BI)提供解决方案和咨询服务,专注于OLAP系统数据引擎开发;
2) 海量并行处理(Massively Parallel Processing) DBMS:
Greenplum的架构采用了MPP(大规模并行处理),在 MPP 系统中,每个 SMP节点也可以运行自己的操作系统、数据库等。换言之,每个节点内的 CPU 不能访问另一个节点的内存。节点之间的信息交互是通过节点互联网络实现的,这个过程一般称为数据重分配(Data Redistribution) 。
SMP(SymmetricMulti-Processing),对称多处理结构的简称,是指在一个计算机上汇集了一组处理器(多CPU),各CPU之间共享内存子系统以及总线结构。在这种技术的支持下,一个服务器系统可以同时运行多个处理器,并共享内存和其他的主机资源。传统的ORACLE和DB2均是此种类型,ORACLE RAC 是半共享状态;
与传统的SMP架构明显不同,通常情况下,MPP系统因为要在不同处理单元之间传送信息,所以它的效率要比SMP要差一点,但是这也不是绝对的,因为 MPP系统不共享资源,因此对它而言,资源比SMP要多,当需要处理的事务达到一定规模时,MPP的效率要比SMP好。这就是看通信时间占用计算时间的比例而定,如果通信时间比较多,那MPP系统就不占优势了,相反,如果通信时间比较少,那MPP系统可以充分发挥资源的优势,达到高效率。
3) 基于PostgreSQL 8.2开源版本,具有相同的客户端功能,增加支持并行处理的技术,增加支持数据仓库和BI的特性;
4) 外部表(external tables)/并行加载(parallel loading):外部表是指数据库可以直接使用操作系统中的数据文件,在Greenplum 4.2版本中支持对外部表的读写操作;
5) 资源管理:基于PostgreSQL增加了并行度的处理;
6) 查询优化器增强(query optimizer enhancements):增加对分布式的支持,空间的回收和分析,不需要进行多方面的调优。
1.2. Greenplum 体系架构

图一
Greenplum是一种基于ProstgreSQL的分布式数据库,其采用Shared-Nothing架构、主机、操作系统、内存、存储都是自我控制的,不存在共享。
补充:SharedDisk与Shared Nothing介绍
图二
图三
比较事项 |
概述 |
优点 |
缺点 |
使用场景 |
Shared Disk |
如图二所示,所有节点共享一份数据 |
只要有一个节点就可以访问所有数据 |
内存融合限制水平扩展能力 |
Oracle RAC,24*7的高可用性核心业务 |
Shared Nothing |
如图三所示,数据和节点有一一对应关系 |
每个节点交互少,很容易扩展 |
如果需要访问所有数据,需要所有节点都可用 |
SQL Server、DB2、Hadoop以及Greenplum |
1.2.1.Master Host
1) 建立与客户端的会话连接和管理;
2) SQL的解析并形成分布式的执行计划;
3) 将生成好的执行计划分发到每个Segment上执行;
4) 收集Segment的执行结果;
5) 不存储业务数据,只存储数据字典;
6) 可以一主一备,分布在两台机器上,为了提高性能,最好单独占用一台机器。
1.2.2.Segment Host
1) 业务数据的存储和存取;
2) 执行由Master分发的SQL语句;
3) 对于Master来说,每个Segment都是对等的,负责对应数据的存储和计算;
4) 每一台机器上可以配置一到多个Segment,因此建议采用相同的机器配置。
1.2.3.Interconnect
1) 是GP数据库的网络层,在每个Segment中起到一个IPC作用;
2) 推荐使用千兆以太网交换机做Interconnect;
3) 支持UDP和TCP两种协议,推荐使用UDP协议,因为其高可靠性、高性能以及可扩展性;而TCP协议最高只能使用1000个Segment实例。
1.3.网络配置示例
图四
图四显示一个常见的网络配置示例,其中X4200是主节点,X4500(Segment host1)是主从节点,当主节点宕机后会主节点服务切换到此节点上,X4500(Segment host2)是从节点。
每个网络接口对应不同的网口,隔离到独立网络,保证不会竞争其他端口的网络带宽,提高网络的可靠性;串口连接到交换机是管理员管理的窗口。
1.4.Greenplum 高可用性体系架构

图五
图五中显示高可用性体系的示例图,其中按照从左到右且从上到下依次是主从节点,主节点,客户端,私有局域网以及从节点集群,实现功能和图一基本一致。
1.5.Master/Standby 镜像保护
图六
图六说明:Standby 节点用于当 Master 节点损坏时提供 Master服务,Standby 实时与Master 节点的Catalog 和事务日志保持同步,确保系统的变更信息不会丢失,提升系统的健壮性。
1.6.数据冗余-Segment 镜像保护

图七
图七说明:
1) 当GP配置了镜像节点之后,主节点不可用时会自动切换到镜像节点,集群仍然保持可用状态。当主节点恢复并启动之后,主节点会自动恢复期间的变更;
2) 只要Master不能连接上Segment实例时,就会在系统表中将此实例标识为不可用,并用镜像节点来代替,一般需要和主节点位于不同的服务器上,当Primary Segment失败时,Mirror Segment将自动提供服务,Primary Segment恢复正常后,使用gprecoverseg –F 同步数据
1.7.Segment 主机硬件配置示例
图八
1.8.网络冗余
图九
图九说明:
1) 数据之间存在冗余,网络也存在冗余;
2) 公共网络连接到主节点,主节点通过一台或者多台交换机连接到子节点。

Hot AI Tools

Undresser.AI Undress
AI-powered app for creating realistic nude photos

AI Clothes Remover
Online AI tool for removing clothes from photos.

Undress AI Tool
Undress images for free

Clothoff.io
AI clothes remover

AI Hentai Generator
Generate AI Hentai for free.

Hot Article

Hot Tools

Notepad++7.3.1
Easy-to-use and free code editor

SublimeText3 Chinese version
Chinese version, very easy to use

Zend Studio 13.0.1
Powerful PHP integrated development environment

Dreamweaver CS6
Visual web development tools

SublimeText3 Mac version
God-level code editing software (SublimeText3)

Hot Topics


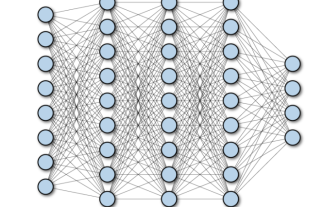
The concept of deep learning originates from the research of artificial neural networks. A multi-layer perceptron containing multiple hidden layers is a deep learning structure. Deep learning combines low-level features to form more abstract high-level representations to represent categories or characteristics of data. It is able to discover distributed feature representations of data. Deep learning is a type of machine learning, and machine learning is the only way to achieve artificial intelligence. So, what are the differences between various deep learning system architectures? 1. Fully Connected Network (FCN) A fully connected network (FCN) consists of a series of fully connected layers, with every neuron in each layer connected to every neuron in another layer. Its main advantage is that it is "structure agnostic", i.e. no special assumptions about the input are required. Although this structural agnostic makes the complete
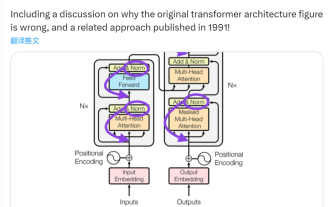
Some time ago, a tweet pointing out the inconsistency between the Transformer architecture diagram and the code in the Google Brain team's paper "AttentionIsAllYouNeed" triggered a lot of discussion. Some people think that Sebastian's discovery was an unintentional mistake, but it is also surprising. After all, considering the popularity of the Transformer paper, this inconsistency should have been mentioned a thousand times. Sebastian Raschka said in response to netizen comments that the "most original" code was indeed consistent with the architecture diagram, but the code version submitted in 2017 was modified, but the architecture diagram was not updated at the same time. This is also the root cause of "inconsistent" discussions.
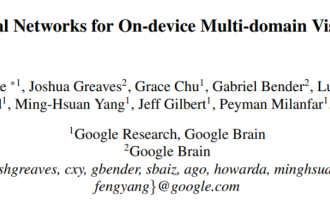
Deep learning models for vision tasks (such as image classification) are usually trained end-to-end with data from a single visual domain (such as natural images or computer-generated images). Generally, an application that completes vision tasks for multiple domains needs to build multiple models for each separate domain and train them independently. Data is not shared between different domains. During inference, each model will handle a specific domain. input data. Even if they are oriented to different fields, some features of the early layers between these models are similar, so joint training of these models is more efficient. This reduces latency and power consumption, and reduces the memory cost of storing each model parameter. This approach is called multi-domain learning (MDL). In addition, MDL models can also outperform single
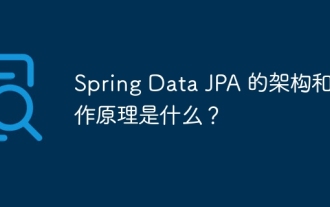
SpringDataJPA is based on the JPA architecture and interacts with the database through mapping, ORM and transaction management. Its repository provides CRUD operations, and derived queries simplify database access. Additionally, it uses lazy loading to only retrieve data when necessary, thus improving performance.
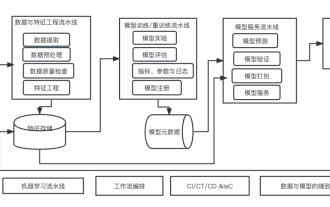
This is an era of AI empowerment, and machine learning is an important technical means to achieve AI. So, is there a universal machine learning system architecture? Within the cognitive scope of experienced programmers, Anything is nothing, especially for system architecture. However, it is possible to build a scalable and reliable machine learning system architecture if applicable to most machine learning driven systems or use cases. From a machine learning life cycle perspective, this so-called universal architecture covers key machine learning stages, from developing machine learning models, to deploying training systems and service systems to production environments. We can try to describe such a machine learning system architecture from the dimensions of 10 elements. 1.
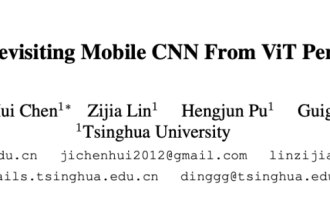
Paper address: https://arxiv.org/abs/2307.09283 Code address: https://github.com/THU-MIG/RepViTRepViT performs well in the mobile ViT architecture and shows significant advantages. Next, we explore the contributions of this study. It is mentioned in the article that lightweight ViTs generally perform better than lightweight CNNs on visual tasks, mainly due to their multi-head self-attention module (MSHA) that allows the model to learn global representations. However, the architectural differences between lightweight ViTs and lightweight CNNs have not been fully studied. In this study, the authors integrated lightweight ViTs into the effective
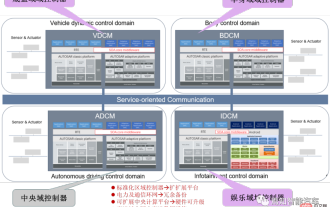
For the next generation of centralized electronic and electrical architecture, the use of central+zonal central computing unit and regional controller layout has become a must-have option for various OEMs or tier1 players. Regarding the architecture of the central computing unit, there are three ways: separation SOC, hardware isolation, software virtualization. The centralized central computing unit will integrate the core business functions of the three major domains of autonomous driving, smart cockpit and vehicle control. The standardized regional controller has three main responsibilities: power distribution, data services, and regional gateway. Therefore, the central computing unit will integrate a high-throughput Ethernet switch. As the degree of integration of the entire vehicle becomes higher and higher, more and more ECU functions will be slowly absorbed into the regional controller. And platformization
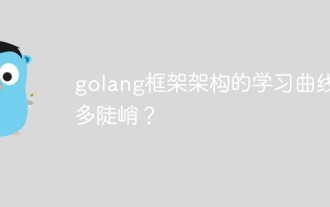
The learning curve of the Go framework architecture depends on familiarity with the Go language and back-end development and the complexity of the chosen framework: a good understanding of the basics of the Go language. It helps to have backend development experience. Frameworks that differ in complexity lead to differences in learning curves.
