理解Python垃圾回收机制
一.垃圾回收机制
Python中的垃圾回收是以引用计数为主,分代收集为辅。引用计数的缺陷是循环引用的问题。
在Python中,如果一个对象的引用数为0,Python虚拟机就会回收这个对象的内存。
#encoding=utf-8 __author__ = 'kevinlu1010@qq.com' class ClassA(): def __init__(self): print 'object born,id:%s'%str(hex(id(self))) def __del__(self): print 'object del,id:%s'%str(hex(id(self))) def f1(): while True: c1=ClassA() del c1
执行f1()会循环输出这样的结果,而且进程占用的内存基本不会变动
object born,id:0x237cf58 object del,id:0x237cf58
c1=ClassA()会创建一个对象,放在0x237cf58内存中,c1变量指向这个内存,这时候这个内存的引用计数是1
del c1后,c1变量不再指向0x237cf58内存,所以这块内存的引用计数减一,等于0,所以就销毁了这个对象,然后释放内存。
1、导致引用计数+1的情况
- 对象被创建,例如a=23
- 对象被引用,例如b=a
- 对象被作为参数,传入到一个函数中,例如func(a)
- 对象作为一个元素,存储在容器中,例如list1=[a,a]
2、导致引用计数-1的情况
- 对象的别名被显式销毁,例如del a
- 对象的别名被赋予新的对象,例如a=24
- 一个对象离开它的作用域,例如f函数执行完毕时,func函数中的局部变量(全局变量不会)
- 对象所在的容器被销毁,或从容器中删除对象
demo
def func(c,d): print 'in func function', sys.getrefcount(c) - 1 print 'init', sys.getrefcount(11) - 1 a = 11 print 'after a=11', sys.getrefcount(11) - 1 b = a print 'after b=1', sys.getrefcount(11) - 1 func(11) print 'after func(a)', sys.getrefcount(11) - 1 list1 = [a, 12, 14] print 'after list1=[a,12,14]', sys.getrefcount(11) - 1 a=12 print 'after a=12', sys.getrefcount(11) - 1 del a print 'after del a', sys.getrefcount(11) - 1 del b print 'after del b', sys.getrefcount(11) - 1 # list1.pop(0) # print 'after pop list1',sys.getrefcount(11)-1 del list1 print 'after del list1', sys.getrefcount(11) - 1
输出
init 24 after a=11 25 after b=1 26 in func function 28 after func(a) 26 after list1=[a,12,14] 27 after a=12 26 after del a 26 after del b 25 after del list1 24
问题:为什么调用函数会令引用计数+2
3、查看一个对象的引用计数
sys.getrefcount(a)可以查看a对象的引用计数,但是比正常计数大1,因为调用函数的时候传入a,这会让a的引用计数+1
二.循环引用导致内存泄露
def f2(): while True: c1=ClassA() c2=ClassA() c1.t=c2 c2.t=c1 del c1 del c2
执行f2(),进程占用的内存会不断增大。
object born,id:0x237cf30 object born,id:0x237cf58
创建了c1,c2后,0x237cf30(c1对应的内存,记为内存1),0x237cf58(c2对应的内存,记为内存2)这两块内存的引用计数都是1,执行c1.t=c2和c2.t=c1后,这两块内存的引用计数变成2.
在del c1后,内存1的对象的引用计数变为1,由于不是为0,所以内存1的对象不会被销毁,所以内存2的对象的引用数依然是2,在del c2后,同理,内存1的对象,内存2的对象的引用数都是1。
虽然它们两个的对象都是可以被销毁的,但是由于循环引用,导致垃圾回收器都不会回收它们,所以就会导致内存泄露。
三.垃圾回收
deff3(): # print gc.collect() c1=ClassA() c2=ClassA() c1.t=c2 c2.t=c1 del c1 del c2 print gc.garbage print gc.collect() #显式执行垃圾回收 print gc.garbage time.sleep(10) if __name__ == '__main__': gc.set_debug(gc.DEBUG_LEAK) #设置gc模块的日志 f3()
输出:
gc: uncollectable <ClassA instance at 0230E918> gc: uncollectable <ClassA instance at 0230E940> gc: uncollectable <dict 0230B810> gc: uncollectable <dict 02301ED0> object born,id:0x230e918 object born,id:0x230e940 4
- 垃圾回收后的对象会放在gc.garbage列表里面
- gc.collect()会返回不可达的对象数目,4等于两个对象以及它们对应的dict
- 有三种情况会触发垃圾回收:
1.调用gc.collect(),
2.当gc模块的计数器达到阀值的时候。
3.程序退出的时候
四.gc模块常用功能解析
gc模块提供一个接口给开发者设置垃圾回收的选项。上面说到,采用引用计数的方法管理内存的一个缺陷是循环引用,而gc模块的一个主要功能就是解决循环引用的问题。
常用函数:
1、gc.set_debug(flags)
设置gc的debug日志,一般设置为gc.DEBUG_LEAK
2、gc.collect([generation])
显式进行垃圾回收,可以输入参数,0代表只检查第一代的对象,1代表检查一,二代的对象,2代表检查一,二,三代的对象,如果不传参数,执行一个full collection,也就是等于传2。
返回不可达(unreachable objects)对象的数目
3、gc.set_threshold(threshold0[, threshold1[, threshold2])
设置自动执行垃圾回收的频率。
4、gc.get_count()
获取当前自动执行垃圾回收的计数器,返回一个长度为3的列表
5、gc模块的自动垃圾回收机制
必须要import gc模块,并且is_enable()=True才会启动自动垃圾回收。
这个机制的主要作用就是发现并处理不可达的垃圾对象。
垃圾回收=垃圾检查+垃圾回收
在Python中,采用分代收集的方法。把对象分为三代,一开始,对象在创建的时候,放在一代中,如果在一次一代的垃圾检查中,改对象存活下来,就会被放到二代中,同理在一次二代的垃圾检查中,该对象存活下来,就会被放到三代中。
gc模块里面会有一个长度为3的列表的计数器,可以通过gc.get_count()获取。
例如(488,3,0),其中488是指距离上一次一代垃圾检查,Python分配内存的数目减去释放内存的数目,注意是内存分配,而不是引用计数的增加。例如:
print gc.get_count() # (590, 8, 0) a = ClassA() print gc.get_count() # (591, 8, 0) del a print gc.get_count() # (590, 8, 0)
3是指距离上一次二代垃圾检查,一代垃圾检查的次数,同理,0是指距离上一次三代垃圾检查,二代垃圾检查的次数。
gc模快有一个自动垃圾回收的阀值,即通过gc.get_threshold函数获取到的长度为3的元组,例如(700,10,10)
每一次计数器的增加,gc模块就会检查增加后的计数是否达到阀值的数目,如果是,就会执行对应的代数的垃圾检查,然后重置计数器
例如,假设阀值是(700,10,10):
- 当计数器从(699,3,0)增加到(700,3,0),gc模块就会执行gc.collect(0),即检查一代对象的垃圾,并重置计数器为(0,4,0)
- 当计数器从(699,9,0)增加到(700,9,0),gc模块就会执行gc.collect(1),即检查一、二代对象的垃圾,并重置计数器为(0,0,1)
- 当计数器从(699,9,9)增加到(700,9,9),gc模块就会执行gc.collect(2),即检查一、二、三代对象的垃圾,并重置计数器为(0,0,0)
其他
如果循环引用中,两个对象都定义了__del__方法,gc模块不会销毁这些不可达对象,因为gc模块不知道应该先调用哪个对象的__del__方法,所以为了安全起见,gc模块会把对象放到gc.garbage中,但是不会销毁对象。
五.应用
- 项目中避免循环引用
- 引入gc模块,启动gc模块的自动清理循环引用的对象机制
- 由于分代收集,所以把需要长期使用的变量集中管理,并尽快移到二代以后,减少GC检查时的消耗
- gc模块唯一处理不了的是循环引用的类都有__del__方法,所以项目中要避免定义__del__方法,如果一定要使用该方法,同时导致了循环引用,需要代码显式调用gc.garbage里面的对象的__del__来打破僵局
以上就是本文的全部内容。希望对大家的学习有所帮助。

Hot AI Tools

Undresser.AI Undress
AI-powered app for creating realistic nude photos

AI Clothes Remover
Online AI tool for removing clothes from photos.

Undress AI Tool
Undress images for free

Clothoff.io
AI clothes remover

AI Hentai Generator
Generate AI Hentai for free.

Hot Article

Hot Tools

Notepad++7.3.1
Easy-to-use and free code editor

SublimeText3 Chinese version
Chinese version, very easy to use

Zend Studio 13.0.1
Powerful PHP integrated development environment

Dreamweaver CS6
Visual web development tools

SublimeText3 Mac version
God-level code editing software (SublimeText3)

Hot Topics
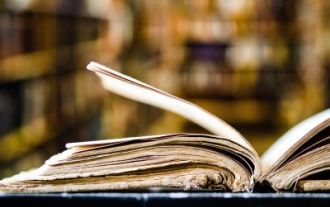
This tutorial demonstrates how to use Python to process the statistical concept of Zipf's law and demonstrates the efficiency of Python's reading and sorting large text files when processing the law. You may be wondering what the term Zipf distribution means. To understand this term, we first need to define Zipf's law. Don't worry, I'll try to simplify the instructions. Zipf's Law Zipf's law simply means: in a large natural language corpus, the most frequently occurring words appear about twice as frequently as the second frequent words, three times as the third frequent words, four times as the fourth frequent words, and so on. Let's look at an example. If you look at the Brown corpus in American English, you will notice that the most frequent word is "th
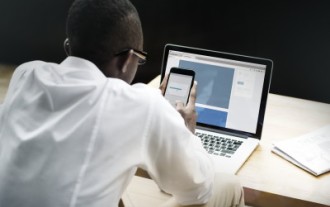
Python provides a variety of ways to download files from the Internet, which can be downloaded over HTTP using the urllib package or the requests library. This tutorial will explain how to use these libraries to download files from URLs from Python. requests library requests is one of the most popular libraries in Python. It allows sending HTTP/1.1 requests without manually adding query strings to URLs or form encoding of POST data. The requests library can perform many functions, including: Add form data Add multi-part file Access Python response data Make a request head
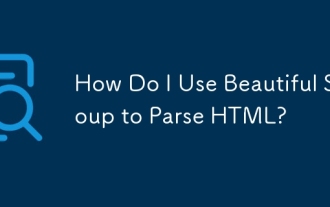
This article explains how to use Beautiful Soup, a Python library, to parse HTML. It details common methods like find(), find_all(), select(), and get_text() for data extraction, handling of diverse HTML structures and errors, and alternatives (Sel
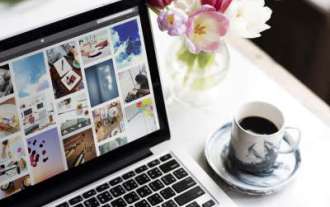
Dealing with noisy images is a common problem, especially with mobile phone or low-resolution camera photos. This tutorial explores image filtering techniques in Python using OpenCV to tackle this issue. Image Filtering: A Powerful Tool Image filter
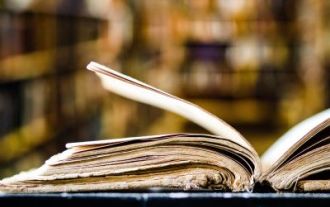
PDF files are popular for their cross-platform compatibility, with content and layout consistent across operating systems, reading devices and software. However, unlike Python processing plain text files, PDF files are binary files with more complex structures and contain elements such as fonts, colors, and images. Fortunately, it is not difficult to process PDF files with Python's external modules. This article will use the PyPDF2 module to demonstrate how to open a PDF file, print a page, and extract text. For the creation and editing of PDF files, please refer to another tutorial from me. Preparation The core lies in using external module PyPDF2. First, install it using pip: pip is P
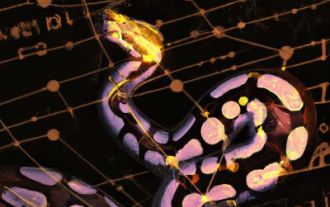
This tutorial demonstrates how to leverage Redis caching to boost the performance of Python applications, specifically within a Django framework. We'll cover Redis installation, Django configuration, and performance comparisons to highlight the bene
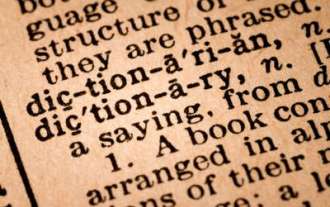
Natural language processing (NLP) is the automatic or semi-automatic processing of human language. NLP is closely related to linguistics and has links to research in cognitive science, psychology, physiology, and mathematics. In the computer science
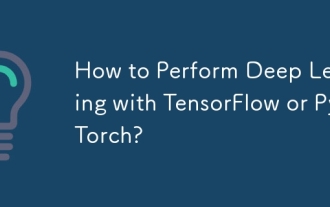
This article compares TensorFlow and PyTorch for deep learning. It details the steps involved: data preparation, model building, training, evaluation, and deployment. Key differences between the frameworks, particularly regarding computational grap
