Python装饰器入门学习教程(九步学习)
装饰器(decorator)是一种高级Python语法。装饰器可以对一个函数、方法或者类进行加工。在Python中,我们有多种方法对函数和类进行加工,比如在Python闭包中,我们见到函数对象作为某一个函数的返回结果。相对于其它方式,装饰器语法简单,代码可读性高。因此,装饰器在Python项目中有广泛的应用。
这是在Python学习小组上介绍的内容,现学现卖、多练习是好的学习方式。
第一步:最简单的函数,准备附加额外功能
# -*- coding:gbk -*- '''示例1: 最简单的函数,表示调用了两次''' def myfunc(): print("myfunc() called.") myfunc() myfunc()
第二步:使用装饰函数在函数执行前和执行后分别附加额外功能
# -*- coding:gbk -*- '''示例2: 替换函数(装饰) 装饰函数的参数是被装饰的函数对象,返回原函数对象 装饰的实质语句: myfunc = deco(myfunc)''' def deco(func): print("before myfunc() called.") func() print(" after myfunc() called.") return func def myfunc(): print(" myfunc() called.") myfunc = deco(myfunc) myfunc() myfunc()
第三步:使用语法糖@来装饰函数
# -*- coding:gbk -*- '''示例3: 使用语法糖@来装饰函数,相当于“myfunc = deco(myfunc)” 但发现新函数只在第一次被调用,且原函数多调用了一次''' def deco(func): print("before myfunc() called.") func() print(" after myfunc() called.") return func @deco def myfunc(): print(" myfunc() called.") myfunc() myfunc()
第四步:使用内嵌包装函数来确保每次新函数都被调用
# -*- coding:gbk -*- '''示例4: 使用内嵌包装函数来确保每次新函数都被调用, 内嵌包装函数的形参和返回值与原函数相同,装饰函数返回内嵌包装函数对象''' def deco(func): def _deco(): print("before myfunc() called.") func() print(" after myfunc() called.") # 不需要返回func,实际上应返回原函数的返回值 return _deco @deco def myfunc(): print(" myfunc() called.") return 'ok' myfunc() myfunc()
第五步:对带参数的函数进行装饰
# -*- coding:gbk -*- '''示例5: 对带参数的函数进行装饰, 内嵌包装函数的形参和返回值与原函数相同,装饰函数返回内嵌包装函数对象''' def deco(func): def _deco(a, b): print("before myfunc() called.") ret = func(a, b) print(" after myfunc() called. result: %s" % ret) return ret return _deco @deco def myfunc(a, b): print(" myfunc(%s,%s) called." % (a, b)) return a + b myfunc(1, 2) myfunc(3, 4)
第六步:对参数量不确定的函数进行装饰
# -*- coding:gbk -*- '''示例6: 对参数数量不确定的函数进行装饰, 参数用(*args, **kwargs),自动适应变参和命名参数''' def deco(func): def _deco(*args, **kwargs): print("before %s called." % func.__name__) ret = func(*args, **kwargs) print(" after %s called. result: %s" % (func.__name__, ret)) return ret return _deco @deco def myfunc(a, b): print(" myfunc(%s,%s) called." % (a, b)) return a+b @deco def myfunc2(a, b, c): print(" myfunc2(%s,%s,%s) called." % (a, b, c)) return a+b+c myfunc(1, 2) myfunc(3, 4) myfunc2(1, 2, 3) myfunc2(3, 4, 5)
第七步:让装饰器带参数
# -*- coding:gbk -*- '''示例7: 在示例4的基础上,让装饰器带参数, 和上一示例相比在外层多了一层包装。 装饰函数名实际上应更有意义些''' def deco(arg): def _deco(func): def __deco(): print("before %s called [%s]." % (func.__name__, arg)) func() print(" after %s called [%s]." % (func.__name__, arg)) return __deco return _deco @deco("mymodule") def myfunc(): print(" myfunc() called.") @deco("module2") def myfunc2(): print(" myfunc2() called.") myfunc() myfunc2()
第八步:让装饰器带 类 参数
# -*- coding:gbk -*- '''示例8: 装饰器带类参数''' class locker: def __init__(self): print("locker.__init__() should be not called.") @staticmethod def acquire(): print("locker.acquire() called.(这是静态方法)") @staticmethod def release(): print(" locker.release() called.(不需要对象实例)") def deco(cls): '''cls 必须实现acquire和release静态方法''' def _deco(func): def __deco(): print("before %s called [%s]." % (func.__name__, cls)) cls.acquire() try: return func() finally: cls.release() return __deco return _deco @deco(locker) def myfunc(): print(" myfunc() called.") myfunc() myfunc()
第九步:装饰器带类参数,并分拆公共类到其他py文件中,同时演示了对一个函数应用多个装饰器
# -*- coding:gbk -*- '''mylocker.py: 公共类 for 示例9.py''' class mylocker: def __init__(self): print("mylocker.__init__() called.") @staticmethod def acquire(): print("mylocker.acquire() called.") @staticmethod def unlock(): print(" mylocker.unlock() called.") class lockerex(mylocker): @staticmethod def acquire(): print("lockerex.acquire() called.") @staticmethod def unlock(): print(" lockerex.unlock() called.") def lockhelper(cls): '''cls 必须实现acquire和release静态方法''' def _deco(func): def __deco(*args, **kwargs): print("before %s called." % func.__name__) cls.acquire() try: return func(*args, **kwargs) finally: cls.unlock() return __deco return _deco # -*- coding:gbk -*-
'''示例9: 装饰器带类参数,并分拆公共类到其他py文件中
同时演示了对一个函数应用多个装饰器'''
from mylocker import * class example: @lockhelper(mylocker) def myfunc(self): print(" myfunc() called.") @lockhelper(mylocker) @lockhelper(lockerex) def myfunc2(self, a, b): print(" myfunc2() called.") return a + b if __name__=="__main__": a = example() a.myfunc() print(a.myfunc()) print(a.myfunc2(1, 2)) print(a.myfunc2(3, 4))
以上给大家分享了Python装饰器入门学习教程(九步学习),希望对大家有所帮助。

Hot AI Tools

Undresser.AI Undress
AI-powered app for creating realistic nude photos

AI Clothes Remover
Online AI tool for removing clothes from photos.

Undress AI Tool
Undress images for free

Clothoff.io
AI clothes remover

AI Hentai Generator
Generate AI Hentai for free.

Hot Article

Hot Tools

Notepad++7.3.1
Easy-to-use and free code editor

SublimeText3 Chinese version
Chinese version, very easy to use

Zend Studio 13.0.1
Powerful PHP integrated development environment

Dreamweaver CS6
Visual web development tools

SublimeText3 Mac version
God-level code editing software (SublimeText3)

Hot Topics
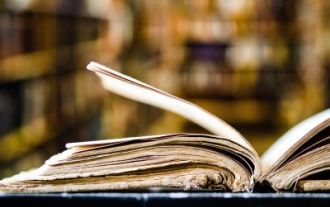
This tutorial demonstrates how to use Python to process the statistical concept of Zipf's law and demonstrates the efficiency of Python's reading and sorting large text files when processing the law. You may be wondering what the term Zipf distribution means. To understand this term, we first need to define Zipf's law. Don't worry, I'll try to simplify the instructions. Zipf's Law Zipf's law simply means: in a large natural language corpus, the most frequently occurring words appear about twice as frequently as the second frequent words, three times as the third frequent words, four times as the fourth frequent words, and so on. Let's look at an example. If you look at the Brown corpus in American English, you will notice that the most frequent word is "th
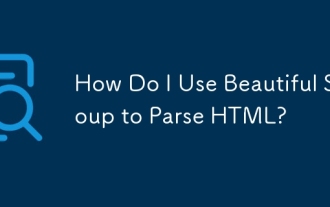
This article explains how to use Beautiful Soup, a Python library, to parse HTML. It details common methods like find(), find_all(), select(), and get_text() for data extraction, handling of diverse HTML structures and errors, and alternatives (Sel
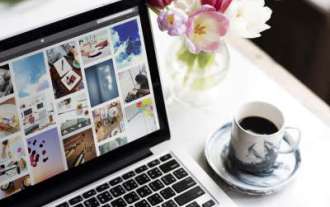
Dealing with noisy images is a common problem, especially with mobile phone or low-resolution camera photos. This tutorial explores image filtering techniques in Python using OpenCV to tackle this issue. Image Filtering: A Powerful Tool Image filter
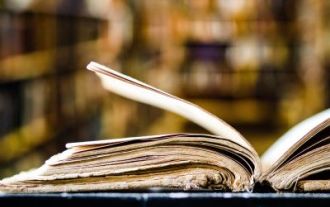
PDF files are popular for their cross-platform compatibility, with content and layout consistent across operating systems, reading devices and software. However, unlike Python processing plain text files, PDF files are binary files with more complex structures and contain elements such as fonts, colors, and images. Fortunately, it is not difficult to process PDF files with Python's external modules. This article will use the PyPDF2 module to demonstrate how to open a PDF file, print a page, and extract text. For the creation and editing of PDF files, please refer to another tutorial from me. Preparation The core lies in using external module PyPDF2. First, install it using pip: pip is P
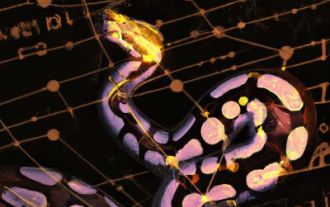
This tutorial demonstrates how to leverage Redis caching to boost the performance of Python applications, specifically within a Django framework. We'll cover Redis installation, Django configuration, and performance comparisons to highlight the bene
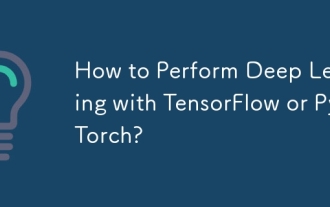
This article compares TensorFlow and PyTorch for deep learning. It details the steps involved: data preparation, model building, training, evaluation, and deployment. Key differences between the frameworks, particularly regarding computational grap
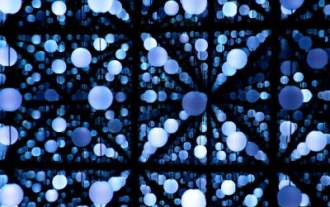
This tutorial demonstrates creating a custom pipeline data structure in Python 3, leveraging classes and operator overloading for enhanced functionality. The pipeline's flexibility lies in its ability to apply a series of functions to a data set, ge
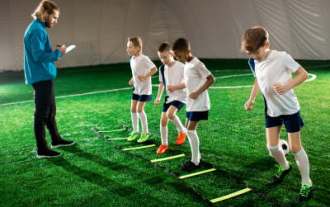
Python, a favorite for data science and processing, offers a rich ecosystem for high-performance computing. However, parallel programming in Python presents unique challenges. This tutorial explores these challenges, focusing on the Global Interprete
