使用优化器来提升Python程序的执行效率的教程
如果不首先想想这句Knuth的名言,就开始进行优化工作是不明智的。可是,你很快写出来加入一些特性的代码,可能会很丑陋,你需要注意了。这篇文章就是为这时候准备的。
那么接下来就是一些很有用的工具和模式来快速优化Python。它的主要目的很简单:尽快发现瓶颈,修复它们并且确认你修复了它们。
写一个测试
在你开始优化前,写一个高级测试来证明原来代码很慢。你可能需要采用一些最小值数据集来复现它足够慢。通常一两个显示运行时秒的程序就足够处理一些改进的地方了。
有一些基础测试来保证你的优化没有改变原有代码的行为也是很必要的。你也能够在很多次运行测试来优化代码的时候稍微修改这些测试的基准。
那么现在,我们来来看看优化工具把。
简单的计时器
计时器很简单,这是一个最灵活的记录执行时间的方法。你可以把它放到任何地方并且副作用很小。运行你自己的计时器非常简单,并且你可以将其定制,使它以你期望的方式工作。例如,你个简单的计时器如下:
import time def timefunc(f): def f_timer(*args, **kwargs): start = time.time() result = f(*args, **kwargs) end = time.time() print f.__name__, 'took', end - start, 'time' return result return f_timer def get_number(): for x in xrange(5000000): yield x @timefunc def expensive_function(): for x in get_number(): i = x ^ x ^ x return 'some result!' # prints "expensive_function took 0.72583088875 seconds" result = expensive_function()
当然,你可以用上下文管理来让它功能更加强大,添加一些检查点或者一些其他的功能:
import time class timewith(): def __init__(self, name=''): self.name = name self.start = time.time() @property def elapsed(self): return time.time() - self.start def checkpoint(self, name=''): print '{timer} {checkpoint} took {elapsed} seconds'.format( timer=self.name, checkpoint=name, elapsed=self.elapsed, ).strip() def __enter__(self): return self def __exit__(self, type, value, traceback): self.checkpoint('finished') pass def get_number(): for x in xrange(5000000): yield x def expensive_function(): for x in get_number(): i = x ^ x ^ x return 'some result!' # prints something like: # fancy thing done with something took 0.582462072372 seconds # fancy thing done with something else took 1.75355315208 seconds # fancy thing finished took 1.7535982132 seconds with timewith('fancy thing') as timer: expensive_function() timer.checkpoint('done with something') expensive_function() expensive_function() timer.checkpoint('done with something else') # or directly timer = timewith('fancy thing') expensive_function() timer.checkpoint('done with something')
计时器还需要你做一些挖掘。包装一些更高级的函数,并且确定瓶颈在哪,然后深入的函数里,能够不停的重现。当你发现一些不合适的代码,修复它,然后测试一遍以确认它被修复了。
一些小技巧:不要忘了好用的timeit模块!它对小块代码做基准测试而不是实际调查更加有用。
- Timer 优点:很容易理解和实现。也非常容易在修改后进行比较。对于很多语言都适用。
- Timer 缺点:有时候对于非常复杂的代码有点过于简单,你可能会花更多时间放置或移动引用代码而不是修复问题!
内建优化器
启用内建的优化器就像是用一门大炮。它非常强大,但是有点不太好用,使用和解释起来比较复杂。
你可以了解更多关于profile模块的东西,但是它的基础是非常简单的:你能够启用和禁用优化器,而且它能打印所有的函数调用和执行时间。它能给你编译和打印出输出。一个简单的装饰器如下:
import cProfile def do_cprofile(func): def profiled_func(*args, **kwargs): profile = cProfile.Profile() try: profile.enable() result = func(*args, **kwargs) profile.disable() return result finally: profile.print_stats() return profiled_func def get_number(): for x in xrange(5000000): yield x @do_cprofile def expensive_function(): for x in get_number(): i = x ^ x ^ x return 'some result!' # perform profiling result = expensive_function()
在上面代码的情况下,你应该看到有些东西在终端打印出来,打印的内容如下:
5000003 function calls in 1.626 seconds Ordered by: standard name ncalls tottime percall cumtime percall filename:lineno(function) 5000001 0.571 0.000 0.571 0.000 timers.py:92(get_number) 1 1.055 1.055 1.626 1.626 timers.py:96(expensive_function) 1 0.000 0.000 0.000 0.000 {method 'disable' of '_lsprof.Profiler' objects}
你可以看到,它给出了不同函数的调用次数,但它遗漏了一些关键的信息:是哪个函数让运行这么慢?
可是,这对于基础优化来说是个好的开始。有时候甚至能用更少的精力找到解决方案。我经常用它来在深入挖掘究竟是哪个函数慢或者调用次数过多之前来调试程序。
- 内建优点:没有额外的依赖并且非常快。对于快速的高等级检查非常有用。
- 内建缺点:信息相对有限,需要进一步的调试;报告有点不太直接,尤其是对于复杂的代码。
Line Profiler
如果内建的优化器是一门大炮,那么line profiler可以看作是一门离子加农炮。它非常的重量级和强大。
在这个例子里,我们会用非常棒的line_profiler库。为了容易使用,我们会再次用装饰器包装一下,这种简单的方法也可以防止把它放在生产代码里。
try: from line_profiler import LineProfiler def do_profile(follow=[]): def inner(func): def profiled_func(*args, **kwargs): try: profiler = LineProfiler() profiler.add_function(func) for f in follow: profiler.add_function(f) profiler.enable_by_count() return func(*args, **kwargs) finally: profiler.print_stats() return profiled_func return inner except ImportError: def do_profile(follow=[]): "Helpful if you accidentally leave in production!" def inner(func): def nothing(*args, **kwargs): return func(*args, **kwargs) return nothing return inner def get_number(): for x in xrange(5000000): yield x @do_profile(follow=[get_number]) def expensive_function(): for x in get_number(): i = x ^ x ^ x return 'some result!' result = expensive_function()
如果你运行上面的代码,你就可以看到一下的报告:
Timer unit: 1e-06 s File: test.py Function: get_number at line 43 Total time: 4.44195 s Line # Hits Time Per Hit % Time Line Contents ============================================================== 43 def get_number(): 44 5000001 2223313 0.4 50.1 for x in xrange(5000000): 45 5000000 2218638 0.4 49.9 yield x File: test.py Function: expensive_function at line 47 Total time: 16.828 s Line # Hits Time Per Hit % Time Line Contents ============================================================== 47 def expensive_function(): 48 5000001 14090530 2.8 83.7 for x in get_number(): 49 5000000 2737480 0.5 16.3 i = x ^ x ^ x 50 1 0 0.0 0.0 return 'some result!'
你可以看到,有一个非常详细的报告,能让你完全洞悉代码运行的情况。不想内建的cProfiler,它能计算话在语言核心特性的时间,比如循环和导入并且给出在不同的行花费的时间。
这些细节能让我们更容易理解函数内部。如果你在研究某个第三方库,你可以直接将其导入并加上装饰器来分析它。
一些小技巧:只装饰你的测试函数并将问题函数作为接下来的参数。
- Line Profiler 优点:有非常直接和详细的报告。能够追踪第三方库里的函数。
- Line Profiler 缺点:因为它会让代码比真正运行时慢很多,所以不要用它来做基准测试。这是额外的需求。
总结和最佳实践
你应该用更简单的工具来对测试用例进行根本的检查,并且用更慢但能显示更多细节的line_profiler来深入到函数内部。
九成情况下,你可能会发现在一个函数里循环调用或一个错误的数据结构消耗了90%的时间。一些调整工具是非常适合你的。
如果你仍然觉得这太慢,而是用一些你自己的秘密武器,如比较属性访问技术或调整平衡检查技术。你也可以用如下的方法:
1.忍受缓慢或者缓存它们
2.重新思考整个实现
3.更多使用优化的数据结构
4.写一个C扩展
注意了,优化代码是种罪恶的快感!用合适的方法来为你的Python代码加速很有意思,但是注意不要破坏了本身的逻辑。可读的代码比运行速度更重要。先把它缓存起来再进行优化其实更好。

Hot AI Tools

Undresser.AI Undress
AI-powered app for creating realistic nude photos

AI Clothes Remover
Online AI tool for removing clothes from photos.

Undress AI Tool
Undress images for free

Clothoff.io
AI clothes remover

AI Hentai Generator
Generate AI Hentai for free.

Hot Article

Hot Tools

Notepad++7.3.1
Easy-to-use and free code editor

SublimeText3 Chinese version
Chinese version, very easy to use

Zend Studio 13.0.1
Powerful PHP integrated development environment

Dreamweaver CS6
Visual web development tools

SublimeText3 Mac version
God-level code editing software (SublimeText3)

Hot Topics


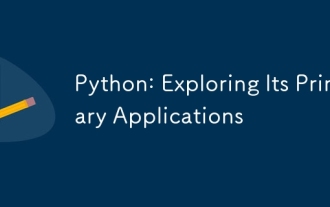
Python is widely used in the fields of web development, data science, machine learning, automation and scripting. 1) In web development, Django and Flask frameworks simplify the development process. 2) In the fields of data science and machine learning, NumPy, Pandas, Scikit-learn and TensorFlow libraries provide strong support. 3) In terms of automation and scripting, Python is suitable for tasks such as automated testing and system management.
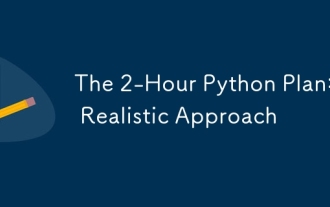
You can learn basic programming concepts and skills of Python within 2 hours. 1. Learn variables and data types, 2. Master control flow (conditional statements and loops), 3. Understand the definition and use of functions, 4. Quickly get started with Python programming through simple examples and code snippets.
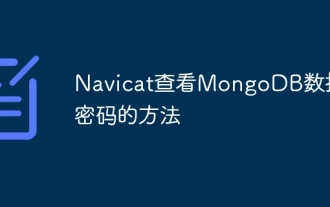
It is impossible to view MongoDB password directly through Navicat because it is stored as hash values. How to retrieve lost passwords: 1. Reset passwords; 2. Check configuration files (may contain hash values); 3. Check codes (may hardcode passwords).
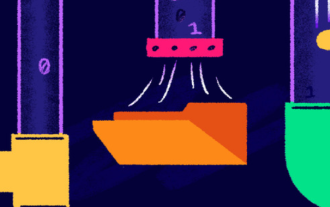
As a data professional, you need to process large amounts of data from various sources. This can pose challenges to data management and analysis. Fortunately, two AWS services can help: AWS Glue and Amazon Athena.
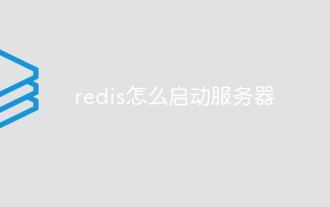
The steps to start a Redis server include: Install Redis according to the operating system. Start the Redis service via redis-server (Linux/macOS) or redis-server.exe (Windows). Use the redis-cli ping (Linux/macOS) or redis-cli.exe ping (Windows) command to check the service status. Use a Redis client, such as redis-cli, Python, or Node.js, to access the server.
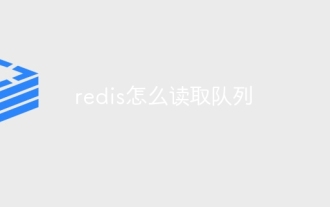
To read a queue from Redis, you need to get the queue name, read the elements using the LPOP command, and process the empty queue. The specific steps are as follows: Get the queue name: name it with the prefix of "queue:" such as "queue:my-queue". Use the LPOP command: Eject the element from the head of the queue and return its value, such as LPOP queue:my-queue. Processing empty queues: If the queue is empty, LPOP returns nil, and you can check whether the queue exists before reading the element.
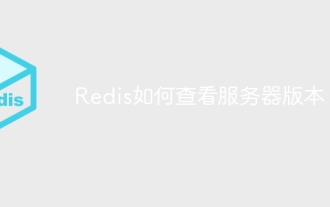
Question: How to view the Redis server version? Use the command line tool redis-cli --version to view the version of the connected server. Use the INFO server command to view the server's internal version and need to parse and return information. In a cluster environment, check the version consistency of each node and can be automatically checked using scripts. Use scripts to automate viewing versions, such as connecting with Python scripts and printing version information.
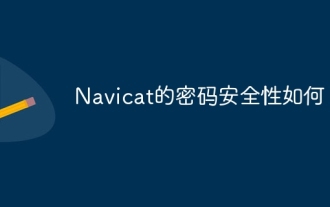
Navicat's password security relies on the combination of symmetric encryption, password strength and security measures. Specific measures include: using SSL connections (provided that the database server supports and correctly configures the certificate), regularly updating Navicat, using more secure methods (such as SSH tunnels), restricting access rights, and most importantly, never record passwords.
