


A new milestone in controllable nuclear fusion, AI achieves fully automatic optimization of dual tokamak 3D field for the first time, published in Nature sub-issue
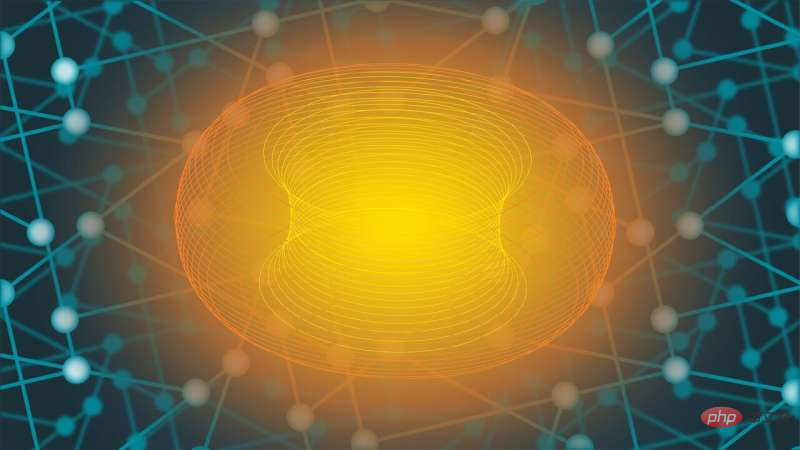
Now, at the Princeton Plasma Physics Laboratory (PPPL), scientists are using artificial intelligence to solve a pressing challenge facing humanity: generating clean, reliable energy through fusion plasma.
Unlike traditional computer code, machine learning is more than just a list of instructions. It can analyze data, infer relationships between features, and learn and adapt from new knowledge.
PPPL+ researchers believe this ability to learn and adapt could improve their control of fusion reactions in a number of ways. This includes perfecting the design of the vessel surrounding the superheated plasma, optimizing heating methods, and maintaining stable control of the reaction over increasingly longer periods of time.
Recently, PPPL’s AI research has achieved significant results. PPPL researchers explain how they use machine learning to avoid magnetic disturbances and stabilize fusion plasmas. This achievement is of great significance for achieving sustainable fusion energy. By analyzing and training large amounts of data, the researchers successfully developed a machine learning model that can accurately
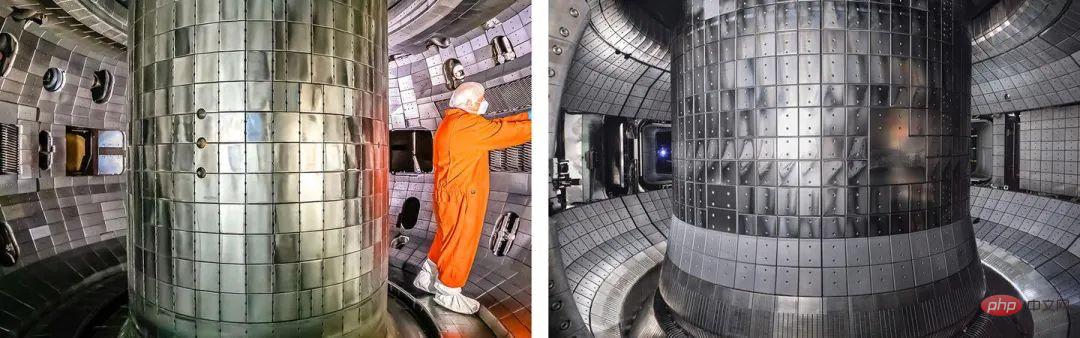
The related research is titled "
Highest fusion performance without harmful edge energy bursts in tokamak" and was published in "#. ##Nature Communications》On.
Paper link: https://www.nature.com/articles/s41467-024-48415-w
In order to be economically competitive in the fusion energy market, it must achieve sufficient ion density (n), temperature (T) while maintaining fusion ) and the high fusion triple product (nτT) of the energy confinement time (τ).
Ions require a sufficient quality factor (G∝ατT) to achieve high fusion denaturation, and this increases with the ion confinement mass (H89: normalized energy confinement time). In order for the tokamak design to become a viable option for fusion reactors, reliable methods must be developed to periodically suppress edge burst events without affecting G. Scientists have used various methods to mitigate fringe outbreaks. One effective approach is to utilize resonant magnetic perturbation (RMP) of external 3D field coils, which has proven to be one of the most promising methods for edge burst suppression.Illustration: 3D field coil structure in a tokamak. (Source: Paper)
First implementation on two tokamak
This research conducted an innovative and integrated 3D field on two tokamak, KSTAR and DIII-D for the first time Optimization, by combining machine learning (ML), adaptive and multi-machine capabilities, to automatically access and achieve almost completely edgeless burst states while improving plasma fusion performance from the initial burst suppression state, which is the edgeless future reactor A major milestone in the outbreak's run.
This is achieved by exploiting the lag between edgeless burst onset and loss in real time to enhance plasma confinement, while expanding ML's capabilities in capturing physics and optimizing nuclear fusion technology.Illustration: Performance comparison of ELM-free discharge in DIII-D and KSTAR tokamak. (Source: paper)
Highly enhanced plasma confinement, reaching the highest fusion G in the Edge Localized Mode-free (ELM-free) scenario of the two machines, with G increased by up to 90%;
First fully automated 3D field optimization using ML-based 3D field simulator;
Simultaneous establishment of burst suppression from the beginning of plasma operation , achieving almost complete edge-free burst operation close to ITER-related levels. This achievement represents a crucial step for future devices such as the International Thermonuclear Experimental Reactor (ITER), where reliance on empirical RMP optimization is no longer a feasible or acceptable approach.
"There are instabilities in the plasma that can cause serious damage to the fusion device. We cannot use these substances in commercial fusion vessels. Our work advances the field and And shows that artificial intelligence can play an important role in managing fusion reactions to avoid instability while allowing the plasma to generate as much fusion energy as possible," said corresponding author Egemen Kolemen, associate professor in the Department of Mechanical and Aerospace Engineering at PPPL.
Fully Automated ML-Based 3D Field Optimization
In this experiment, a series of discharges are used to find optimized 3D waveforms for safe ELM suppression.
In this context, the study introduces ML techniques to develop novel paths for automated 3D coil optimization and demonstrates the concept for the first time.
Researchers developed a surrogate model of the GPEC code (ML-3D) to leverage physics-based models in real time. The model uses ML algorithms to accelerate computation time to the ms level and is integrated into the adaptive RMP optimizer in KSTAR.
ML-3D consists of a fully connected multilayer perceptron (MLP) driven by nine inputs. To train the model, 8490 KSTAR balanced GPEC simulations were utilized.
The algorithm utilizes the ELM status monitor (Dα) signal to adjust the IRMP in real time, which can maintain sufficient edge 3D fields to access and maintain ELM suppression. At the same time, the 3D field optimizer uses the output of ML-3D to adjust the current distribution on the 3D coil, thus ensuring a safe 3D field to avoid interruptions.
In KSTAR experiments, the ML-integrated adaptive RMP optimizer triggered in 4.5 seconds and achieved safe ELM suppression in 6.2 seconds.
Research also demonstrates 3D-ML as a viable solution for automating ELM-free access. ML-3D is based on physical models and does not require experimental data, making it directly scalable to ITER and future fusion reactors. This strong applicability to future devices highlights the advantages of ML's integrated 3D field optimization approach. Furthermore, better field optimization and higher fusion performance are expected to be achieved in future devices with higher 3D coil current limitations.
Research successfully optimized controlled ELM-free states in KSTAR and DIII-D devices with highly enhanced fusion performance, covering low-n RMP related to future reactors to nRMP related to ITER = 3 RMP, and achieved the highest level in various ELM-free scenarios in two machines.
In addition, the innovative integration of ML algorithms with RMP control enables fully automatic 3D field optimization and ELM-free operation for the first time, and with the support of adaptive optimization processes, performance is significantly enhanced . This adaptive approach demonstrates compatibility between RMP ELM suppression and high limits.
Additionally, it provides a robust strategy to achieve stable ELM suppression in long pulse scenarios (lasting more than 45 seconds) by minimizing the loss of limiting and uninductive current fractions.
Notably, significant performance (G) improvement was observed in DIII-D with nRMP = 3 RMP, showing an improvement of more than 90% from the initial standard ELM suppression state. This enhancement is attributed not only to the adaptive RMP control but also to the self-consistent evolution of the plasma rotation. This response enables ELM suppression at very low RMP amplitudes, thereby enhancing the base. This feature is a good example of a system transitioning to an optimal state through a self-organized response to adaptive modulation.
In addition, the adaptive scheme is combined with the early RMP-ramp method to achieve ITER-related ELM-free scenarios with almost completely ELM-free operation. These results confirm that integrated adaptive RMP control is a very promising approach to optimize ELM suppression states, with the potential to address one of the most difficult challenges in achieving practical and economically viable fusion energy.
Reference content: https://phys.org/news/2024-05-ai-intensive-aspects-plasma-physics.html
【 Recommended reading]
The above is the detailed content of A new milestone in controllable nuclear fusion, AI achieves fully automatic optimization of dual tokamak 3D field for the first time, published in Nature sub-issue. For more information, please follow other related articles on the PHP Chinese website!

Hot AI Tools

Undresser.AI Undress
AI-powered app for creating realistic nude photos

AI Clothes Remover
Online AI tool for removing clothes from photos.

Undress AI Tool
Undress images for free

Clothoff.io
AI clothes remover

AI Hentai Generator
Generate AI Hentai for free.

Hot Article

Hot Tools

Notepad++7.3.1
Easy-to-use and free code editor

SublimeText3 Chinese version
Chinese version, very easy to use

Zend Studio 13.0.1
Powerful PHP integrated development environment

Dreamweaver CS6
Visual web development tools

SublimeText3 Mac version
God-level code editing software (SublimeText3)

Hot Topics
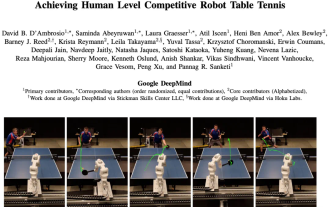
But maybe he can’t defeat the old man in the park? The Paris Olympic Games are in full swing, and table tennis has attracted much attention. At the same time, robots have also made new breakthroughs in playing table tennis. Just now, DeepMind proposed the first learning robot agent that can reach the level of human amateur players in competitive table tennis. Paper address: https://arxiv.org/pdf/2408.03906 How good is the DeepMind robot at playing table tennis? Probably on par with human amateur players: both forehand and backhand: the opponent uses a variety of playing styles, and the robot can also withstand: receiving serves with different spins: However, the intensity of the game does not seem to be as intense as the old man in the park. For robots, table tennis
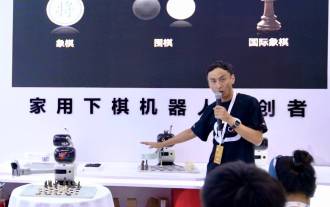
On August 21, the 2024 World Robot Conference was grandly held in Beijing. SenseTime's home robot brand "Yuanluobot SenseRobot" has unveiled its entire family of products, and recently released the Yuanluobot AI chess-playing robot - Chess Professional Edition (hereinafter referred to as "Yuanluobot SenseRobot"), becoming the world's first A chess robot for the home. As the third chess-playing robot product of Yuanluobo, the new Guoxiang robot has undergone a large number of special technical upgrades and innovations in AI and engineering machinery. For the first time, it has realized the ability to pick up three-dimensional chess pieces through mechanical claws on a home robot, and perform human-machine Functions such as chess playing, everyone playing chess, notation review, etc.
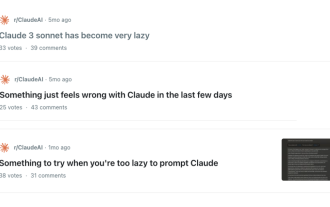
The start of school is about to begin, and it’s not just the students who are about to start the new semester who should take care of themselves, but also the large AI models. Some time ago, Reddit was filled with netizens complaining that Claude was getting lazy. "Its level has dropped a lot, it often pauses, and even the output becomes very short. In the first week of release, it could translate a full 4-page document at once, but now it can't even output half a page!" https:// www.reddit.com/r/ClaudeAI/comments/1by8rw8/something_just_feels_wrong_with_claude_in_the/ in a post titled "Totally disappointed with Claude", full of
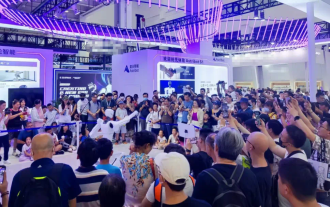
At the World Robot Conference being held in Beijing, the display of humanoid robots has become the absolute focus of the scene. At the Stardust Intelligent booth, the AI robot assistant S1 performed three major performances of dulcimer, martial arts, and calligraphy in one exhibition area, capable of both literary and martial arts. , attracted a large number of professional audiences and media. The elegant playing on the elastic strings allows the S1 to demonstrate fine operation and absolute control with speed, strength and precision. CCTV News conducted a special report on the imitation learning and intelligent control behind "Calligraphy". Company founder Lai Jie explained that behind the silky movements, the hardware side pursues the best force control and the most human-like body indicators (speed, load) etc.), but on the AI side, the real movement data of people is collected, allowing the robot to become stronger when it encounters a strong situation and learn to evolve quickly. And agile

Deep integration of vision and robot learning. When two robot hands work together smoothly to fold clothes, pour tea, and pack shoes, coupled with the 1X humanoid robot NEO that has been making headlines recently, you may have a feeling: we seem to be entering the age of robots. In fact, these silky movements are the product of advanced robotic technology + exquisite frame design + multi-modal large models. We know that useful robots often require complex and exquisite interactions with the environment, and the environment can be represented as constraints in the spatial and temporal domains. For example, if you want a robot to pour tea, the robot first needs to grasp the handle of the teapot and keep it upright without spilling the tea, then move it smoothly until the mouth of the pot is aligned with the mouth of the cup, and then tilt the teapot at a certain angle. . this
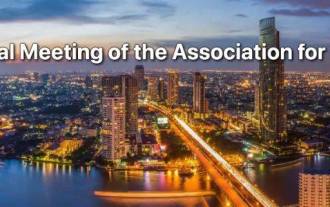
At this ACL conference, contributors have gained a lot. The six-day ACL2024 is being held in Bangkok, Thailand. ACL is the top international conference in the field of computational linguistics and natural language processing. It is organized by the International Association for Computational Linguistics and is held annually. ACL has always ranked first in academic influence in the field of NLP, and it is also a CCF-A recommended conference. This year's ACL conference is the 62nd and has received more than 400 cutting-edge works in the field of NLP. Yesterday afternoon, the conference announced the best paper and other awards. This time, there are 7 Best Paper Awards (two unpublished), 1 Best Theme Paper Award, and 35 Outstanding Paper Awards. The conference also awarded 3 Resource Paper Awards (ResourceAward) and Social Impact Award (
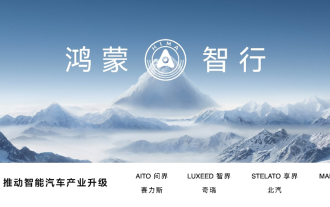
This afternoon, Hongmeng Zhixing officially welcomed new brands and new cars. On August 6, Huawei held the Hongmeng Smart Xingxing S9 and Huawei full-scenario new product launch conference, bringing the panoramic smart flagship sedan Xiangjie S9, the new M7Pro and Huawei novaFlip, MatePad Pro 12.2 inches, the new MatePad Air, Huawei Bisheng With many new all-scenario smart products including the laser printer X1 series, FreeBuds6i, WATCHFIT3 and smart screen S5Pro, from smart travel, smart office to smart wear, Huawei continues to build a full-scenario smart ecosystem to bring consumers a smart experience of the Internet of Everything. Hongmeng Zhixing: In-depth empowerment to promote the upgrading of the smart car industry Huawei joins hands with Chinese automotive industry partners to provide
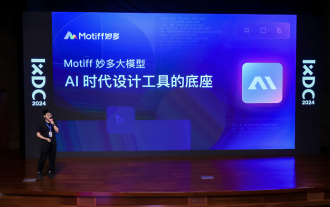
Artificial intelligence is developing faster than you might imagine. Since GPT-4 introduced multimodal technology into the public eye, multimodal large models have entered a stage of rapid development, gradually shifting from pure model research and development to exploration and application in vertical fields, and are deeply integrated with all walks of life. In the field of interface interaction, international technology giants such as Google and Apple have invested in the research and development of large multi-modal UI models, which is regarded as the only way forward for the mobile phone AI revolution. In this context, the first large-scale UI model in China was born. On August 17, at the IXDC2024 International Experience Design Conference, Motiff, a design tool in the AI era, launched its independently developed UI multi-modal model - Motiff Model. This is the world's first UI design tool
