By taking advantage of C++, we can build machine learning models to process large data sets: Optimize memory management: use smart pointers (such as unique_ptr, shared_ptr) Use memory pools Parallel processing: multi-threading ( Using std::thread library) OpenMP parallel programming standard CUDA Utilizing GPU parallel processing capabilities Data compression: using binary file formats (such as HDF5, Parquet) using sparse data structures (such as sparse arrays, hash tables)
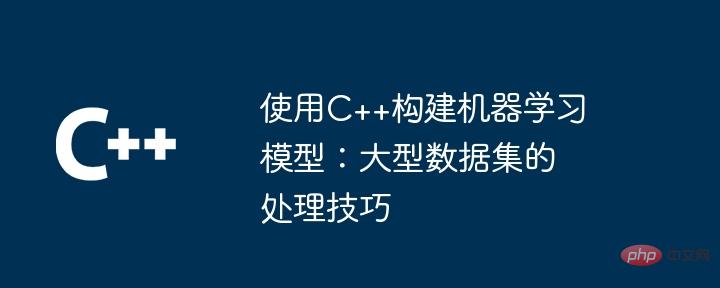
Building Machine Learning Models in C++: Tips for Handling Large Data Sets
In today’s data-driven era, handling large data sets is crucial for machine learning. C++ is known for its efficiency and flexibility, making it ideal for building machine learning models.
Optimize memory management
-
Use smart pointers: Smart pointers automatically manage memory and release the memory when the object is no longer used. For example, unique_ptr is suitable for a single object, and shared_ptr is suitable for objects that require shared ownership.
-
Use memory pool: The memory pool pre-allocates a piece of memory and allows objects that require memory to choose space from it. This can avoid frequent allocation and deconfiguration and improve performance.
Parallel processing
-
Multi-threading: C++ supports the creation and management of multi-threads using the std::thread library, This can parallelize computationally intensive tasks.
-
OpenMP: OpenMP is a parallel programming standard that allows easy creation of parallel regions using the #pragma directive.
-
CUDA: CUDA allows leveraging the parallel processing capabilities of GPUs and is suitable for tasks such as image processing and deep learning.
Data compression
-
Use binary file formats: such as HDF5 or Apache Parquet, compared to plain text files, Data set size can be significantly reduced.
-
Use sparse data structures: For sparse data sets with a large number of zero values, you can use sparse arrays or hash tables to store the data efficiently.
Practical Case: Large-Scale Image Classification
Using C++ and OpenCV, we can build a machine learning model to classify a large number of images. Here is an example:
#include <opencv2/opencv.hpp>
#include <vector>
using namespace cv;
using namespace std;
int main() {
// 加载图像数据
vector<Mat> images;
vector<int> labels;
load_data(images, labels);
// 训练分类器
Ptr<ml::SVM> svm = ml::SVM::create();
svm->train(images, ml::ROW_SAMPLE, labels);
// 使用分类器进行预测
Mat test_image = imread("test_image.jpg");
int predicted_label = svm->predict(test_image);
// 输出预测结果
cout << "Predicted label: " << predicted_label << endl;
return 0;
}
Copy after login
The above is the detailed content of Building Machine Learning Models in C++: Tips for Handling Large Data Sets. For more information, please follow other related articles on the PHP Chinese website!