


1.5 times beyond the diffraction limit, imaging conditions are 10 times lower, Tsinghua University and the Chinese Academy of Sciences use AI methods to improve microscope resolution
Editor | Radishpi
Computational super-resolution methods, including traditional analysis algorithms and deep learning models, have greatly improved optical microscopy. Among them, supervised deep neural networks have shown excellent performance, but due to the high dynamics of living cells, a large amount of high-quality training data is required, and obtaining these data is very laborious and impractical.
In the latest study, researchers from Tsinghua University and the Chinese Academy of Sciences developed zero-shot deconvolution networks (ZS-DeconvNet) that can instantly increase the resolution of microscope images by more than 1.5 times more than the diffraction limit while fluorescence is 10 times lower than ordinary super-resolution imaging conditions, performed in an unsupervised manner without the need for ground experiments or additional data collection.
The researchers also demonstrated the versatile applicability of ZS-DeconvNet to multiple imaging modes, including total internal reflection fluorescence microscopy, three-dimensional wide-field microscopy, confocal microscopy, two-photon microscopy, and lattice light microscopy. Slice microscopy and multi-modal structured illumination microscopy; it enables multi-color, long-term, super-resolution 2D/3D imaging of multi-cell embryonic organisms from mitotic single cells to mice and Caenorhabditis elegans.
The research is titled "Zero-shot learning enables instant denoising and super-resolution in optical fluorescence microscopy" and was published in "Nature Communications" on May 16, 2024 》.
Optical fluorescence microscopy is crucial for biological research. The advancement of super-resolution technology has improved imaging details, but along with the improvement of spatial resolution comes the trade-off of other imaging parameters. Computational super-resolution methods have become a research hotspot due to their ability to improve image quality online, enhance the capabilities of existing equipment, and expand the scope of applications.
These methods are divided into two categories: deconvolution and other technologies based on analytical models and super-resolution (SR) networks based on deep learning. The former is limited by parameter tuning and poor adaptability to complex imaging environments. The latter can learn complex image transformations through big data, but faces the challenge of difficulty in obtaining and high dependence on the quality of training data. This limits the popularity of deep learning super-resolution technology in daily applications in biological research.
Here, a research team from Tsinghua University and the Chinese Academy of Sciences proposed a zero-shot deconvolution deep neural network framework ZS-DeconvNet, which is capable of unsupervised training of the DLSR network using only a low-resolution and Planar or volumetric image stacks with low signal-to-noise ratio, enabling zero-shot implementation.
Therefore, compared with state-of-the-art DLSR methods, ZS-DeconvNet can adapt to different biological imaging environments, where biological processes are too dynamic and sensitive to light to obtain real SR images , or the image acquisition process is affected by unknown and non-ideal factors.
The researchers said that even when trained on a single low signal-to-noise ratio input image, ZS-DeconvNet can improve resolution beyond the diffraction limit by more than 1.5 times with high fidelity and quantification, and No image-specific parameter adjustments are required.
ZS-DeconvNet is suitable for a variety of imaging modalities, from scanning microscopy to widefield detection microscopy, and has demonstrated its capabilities in a variety of samples and microscopy setups.
Illustration: Generalizing ZS-DeconvNet to multiple imaging modalities. (Source: paper)
Researchers demonstrate that properly trained ZS-DeconvNet can infer high-resolution images on millisecond timescales, enabling light-sensitive processes of multiple organelle interactions, migration, and mitosis High-throughput long-term SR 2D/3D imaging of cytoskeletal and organelle dynamics in C. elegans and subcellular structures and dynamics in developing C. elegans and mouse embryos.
Illustration: Zero-sample denoising and resolution enhancement in multimodal SIM data. (Source: paper)
In addition, in order to make ZS-DeconvNet widely used by the biological research community, the team established a Fiji plug-in toolbox and a tutorial homepage of the ZS-DeconvNet method. Users do not need deep learning knowledge Also easy to use.
Despite its broad applicability and robustness, ZS-DeconvNet users need to be aware of potential phantom generation and its limitations, such as misidentification of low-fluorescence signals, performance degradation when applied to images of different imaging modes, and problems caused by improper PSF matching , and the resolution improvement under unsupervised learning is not as obvious as supervised learning.
In the future, by combining more advanced network architectures, expanding to other optical super-resolution technologies, adopting domain adaptation or generalization technologies, and processing spatially varying PSFs, the functions and application scope of ZS-DeconvNet will be further expanded. .
Paper link:https://www.nature.com/articles/s41467-024-48575-9
The above is the detailed content of 1.5 times beyond the diffraction limit, imaging conditions are 10 times lower, Tsinghua University and the Chinese Academy of Sciences use AI methods to improve microscope resolution. For more information, please follow other related articles on the PHP Chinese website!

Hot AI Tools

Undresser.AI Undress
AI-powered app for creating realistic nude photos

AI Clothes Remover
Online AI tool for removing clothes from photos.

Undress AI Tool
Undress images for free

Clothoff.io
AI clothes remover

Video Face Swap
Swap faces in any video effortlessly with our completely free AI face swap tool!

Hot Article

Hot Tools

Notepad++7.3.1
Easy-to-use and free code editor

SublimeText3 Chinese version
Chinese version, very easy to use

Zend Studio 13.0.1
Powerful PHP integrated development environment

Dreamweaver CS6
Visual web development tools

SublimeText3 Mac version
God-level code editing software (SublimeText3)

Hot Topics
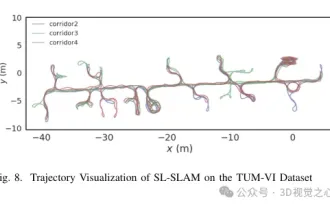
Written previously, today we discuss how deep learning technology can improve the performance of vision-based SLAM (simultaneous localization and mapping) in complex environments. By combining deep feature extraction and depth matching methods, here we introduce a versatile hybrid visual SLAM system designed to improve adaptation in challenging scenarios such as low-light conditions, dynamic lighting, weakly textured areas, and severe jitter. sex. Our system supports multiple modes, including extended monocular, stereo, monocular-inertial, and stereo-inertial configurations. In addition, it also analyzes how to combine visual SLAM with deep learning methods to inspire other research. Through extensive experiments on public datasets and self-sampled data, we demonstrate the superiority of SL-SLAM in terms of positioning accuracy and tracking robustness.
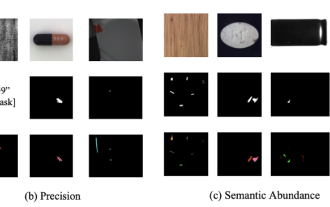
In modern manufacturing, accurate defect detection is not only the key to ensuring product quality, but also the core of improving production efficiency. However, existing defect detection datasets often lack the accuracy and semantic richness required for practical applications, resulting in models unable to identify specific defect categories or locations. In order to solve this problem, a top research team composed of Hong Kong University of Science and Technology Guangzhou and Simou Technology innovatively developed the "DefectSpectrum" data set, which provides detailed and semantically rich large-scale annotation of industrial defects. As shown in Table 1, compared with other industrial data sets, the "DefectSpectrum" data set provides the most defect annotations (5438 defect samples) and the most detailed defect classification (125 defect categories
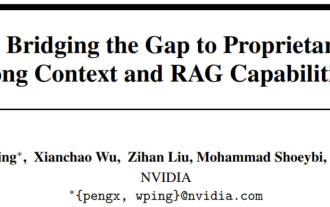
The open LLM community is an era when a hundred flowers bloom and compete. You can see Llama-3-70B-Instruct, QWen2-72B-Instruct, Nemotron-4-340B-Instruct, Mixtral-8x22BInstruct-v0.1 and many other excellent performers. Model. However, compared with proprietary large models represented by GPT-4-Turbo, open models still have significant gaps in many fields. In addition to general models, some open models that specialize in key areas have been developed, such as DeepSeek-Coder-V2 for programming and mathematics, and InternVL for visual-language tasks.
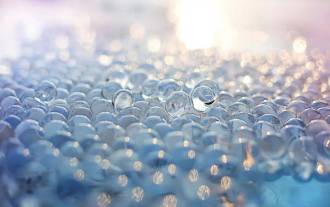
Editor |KX To this day, the structural detail and precision determined by crystallography, from simple metals to large membrane proteins, are unmatched by any other method. However, the biggest challenge, the so-called phase problem, remains retrieving phase information from experimentally determined amplitudes. Researchers at the University of Copenhagen in Denmark have developed a deep learning method called PhAI to solve crystal phase problems. A deep learning neural network trained using millions of artificial crystal structures and their corresponding synthetic diffraction data can generate accurate electron density maps. The study shows that this deep learning-based ab initio structural solution method can solve the phase problem at a resolution of only 2 Angstroms, which is equivalent to only 10% to 20% of the data available at atomic resolution, while traditional ab initio Calculation
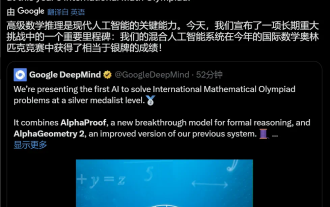
For AI, Mathematical Olympiad is no longer a problem. On Thursday, Google DeepMind's artificial intelligence completed a feat: using AI to solve the real question of this year's International Mathematical Olympiad IMO, and it was just one step away from winning the gold medal. The IMO competition that just ended last week had six questions involving algebra, combinatorics, geometry and number theory. The hybrid AI system proposed by Google got four questions right and scored 28 points, reaching the silver medal level. Earlier this month, UCLA tenured professor Terence Tao had just promoted the AI Mathematical Olympiad (AIMO Progress Award) with a million-dollar prize. Unexpectedly, the level of AI problem solving had improved to this level before July. Do the questions simultaneously on IMO. The most difficult thing to do correctly is IMO, which has the longest history, the largest scale, and the most negative
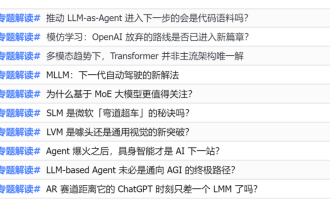
In 2023, almost every field of AI is evolving at an unprecedented speed. At the same time, AI is constantly pushing the technological boundaries of key tracks such as embodied intelligence and autonomous driving. Under the multi-modal trend, will the situation of Transformer as the mainstream architecture of AI large models be shaken? Why has exploring large models based on MoE (Mixed of Experts) architecture become a new trend in the industry? Can Large Vision Models (LVM) become a new breakthrough in general vision? ...From the 2023 PRO member newsletter of this site released in the past six months, we have selected 10 special interpretations that provide in-depth analysis of technological trends and industrial changes in the above fields to help you achieve your goals in the new year. be prepared. This interpretation comes from Week50 2023
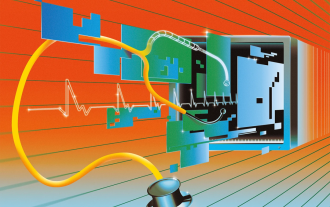
Editor | ScienceAI Based on limited clinical data, hundreds of medical algorithms have been approved. Scientists are debating who should test the tools and how best to do so. Devin Singh witnessed a pediatric patient in the emergency room suffer cardiac arrest while waiting for treatment for a long time, which prompted him to explore the application of AI to shorten wait times. Using triage data from SickKids emergency rooms, Singh and colleagues built a series of AI models that provide potential diagnoses and recommend tests. One study showed that these models can speed up doctor visits by 22.3%, speeding up the processing of results by nearly 3 hours per patient requiring a medical test. However, the success of artificial intelligence algorithms in research only verifies this

Editor | Radish Skin Since the release of the powerful AlphaFold2 in 2021, scientists have been using protein structure prediction models to map various protein structures within cells, discover drugs, and draw a "cosmic map" of every known protein interaction. . Just now, Google DeepMind released the AlphaFold3 model, which can perform joint structure predictions for complexes including proteins, nucleic acids, small molecules, ions and modified residues. The accuracy of AlphaFold3 has been significantly improved compared to many dedicated tools in the past (protein-ligand interaction, protein-nucleic acid interaction, antibody-antigen prediction). This shows that within a single unified deep learning framework, it is possible to achieve
