


How to evaluate the performance and scalability of different open source alternatives?
When evaluating the performance and scalability of different open source alternatives: Performance evaluation includes benchmarking, stress testing, and profiling. Scalability assessment includes horizontal scalability, vertical scalability, and cloud scalability. For high performance and scalability applications, FastAPI and Sanic are better alternatives to Flask.
How to evaluate the performance and scalability of different open source alternatives
When evaluating open source software (OSS) alternatives, performance and scalability are crucial considerations. This article guides you through the assessment process and provides practical examples to help you understand how to apply these principles.
Performance Evaluation
- Benchmarking: Use industry standard benchmarks to measure the processing power of different alternatives, such as the TechEmpower Web Framework Benchmark .
- Stress testing: Simulate high load situations to evaluate how alternatives perform under extreme conditions. Consider metrics such as concurrent users, request size, and response time.
- Profiling: Use tools such as Profiler or gprof to identify performance bottlenecks and optimization opportunities.
Scalability Assessment
- Horizontal Scalability: Measures how well alternatives handle the load when adding more servers Ability. Evaluate each server's throughput and response time.
- Vertical Scalability: Measures the ability of an alternative to handle load as you increase the resources (such as CPU cores or memory) of a single server.
- Cloud Scalability: Evaluate whether alternatives leverage cloud computing services to scale, such as elastic load balancing and auto-scaling.
Practical Example
Consider using the following alternatives to replace the Flask Web Framework:
- Django
- FastAPI
- Sanic
Performance Benchmark
TechEmpower Score | |
---|---|
541 | |
321 | |
879 | |
1028 |
10,000 concurrent users | |
---|---|
Response time: 150ms | |
Response time: 250ms | |
Response time: 50ms | |
Response time: 20ms |
8 CPU cores | |
---|---|
Throughput: 5k RPS | |
Throughput: 7k RPS | |
Throughput: 12k RPS | |
Throughput: 15k RPS |
The above is the detailed content of How to evaluate the performance and scalability of different open source alternatives?. For more information, please follow other related articles on the PHP Chinese website!

Hot AI Tools

Undresser.AI Undress
AI-powered app for creating realistic nude photos

AI Clothes Remover
Online AI tool for removing clothes from photos.

Undress AI Tool
Undress images for free

Clothoff.io
AI clothes remover

AI Hentai Generator
Generate AI Hentai for free.

Hot Article

Hot Tools

Notepad++7.3.1
Easy-to-use and free code editor

SublimeText3 Chinese version
Chinese version, very easy to use

Zend Studio 13.0.1
Powerful PHP integrated development environment

Dreamweaver CS6
Visual web development tools

SublimeText3 Mac version
God-level code editing software (SublimeText3)

Hot Topics


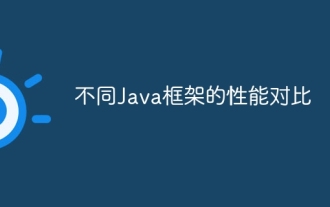
Performance comparison of different Java frameworks: REST API request processing: Vert.x is the best, with a request rate of 2 times SpringBoot and 3 times Dropwizard. Database query: SpringBoot's HibernateORM is better than Vert.x and Dropwizard's ORM. Caching operations: Vert.x's Hazelcast client is superior to SpringBoot and Dropwizard's caching mechanisms. Suitable framework: Choose according to application requirements. Vert.x is suitable for high-performance web services, SpringBoot is suitable for data-intensive applications, and Dropwizard is suitable for microservice architecture.
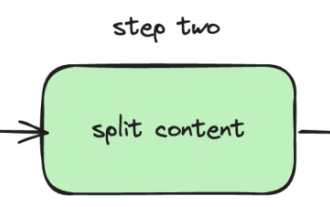
Ollama is a super practical tool that allows you to easily run open source models such as Llama2, Mistral, and Gemma locally. In this article, I will introduce how to use Ollama to vectorize text. If you have not installed Ollama locally, you can read this article. In this article we will use the nomic-embed-text[2] model. It is a text encoder that outperforms OpenAI text-embedding-ada-002 and text-embedding-3-small on short context and long context tasks. Start the nomic-embed-text service when you have successfully installed o
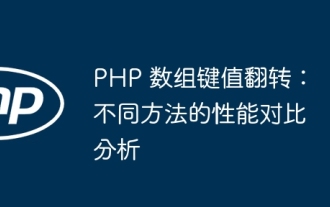
The performance comparison of PHP array key value flipping methods shows that the array_flip() function performs better than the for loop in large arrays (more than 1 million elements) and takes less time. The for loop method of manually flipping key values takes a relatively long time.
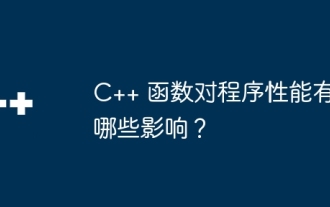
The impact of functions on C++ program performance includes function call overhead, local variable and object allocation overhead: Function call overhead: including stack frame allocation, parameter transfer and control transfer, which has a significant impact on small functions. Local variable and object allocation overhead: A large number of local variable or object creation and destruction can cause stack overflow and performance degradation.
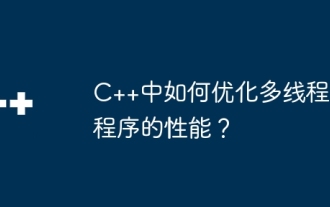
Effective techniques for optimizing C++ multi-threaded performance include limiting the number of threads to avoid resource contention. Use lightweight mutex locks to reduce contention. Optimize the scope of the lock and minimize the waiting time. Use lock-free data structures to improve concurrency. Avoid busy waiting and notify threads of resource availability through events.
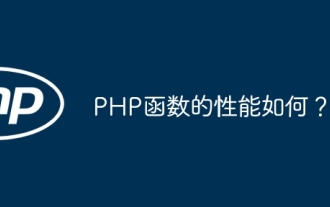
The performance of different PHP functions is crucial to application efficiency. Functions with better performance include echo and print, while functions such as str_replace, array_merge, and file_get_contents have slower performance. For example, the str_replace function is used to replace strings and has moderate performance, while the sprintf function is used to format strings. Performance analysis shows that it only takes 0.05 milliseconds to execute one example, proving that the function performs well. Therefore, using functions wisely can lead to faster and more efficient applications.
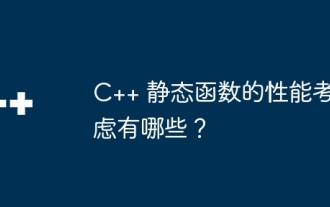
Static function performance considerations are as follows: Code size: Static functions are usually smaller because they do not contain member variables. Memory occupation: does not belong to any specific object and does not occupy object memory. Calling overhead: lower, no need to call through object pointer or reference. Multi-thread-safe: Generally thread-safe because there is no dependence on class instances.
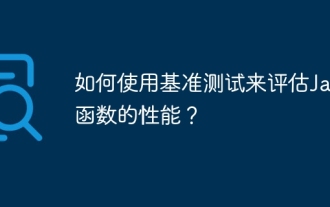
A way to benchmark the performance of Java functions is to use the Java Microbenchmark Suite (JMH). Specific steps include: Adding JMH dependencies to the project. Create a new Java class and annotate it with @State to represent the benchmark method. Write the benchmark method in the class and annotate it with @Benchmark. Run the benchmark using the JMH command line tool.
