


To improve the utilization of optical data sets, the Tianda team proposed an AI model to enhance spectral prediction effects
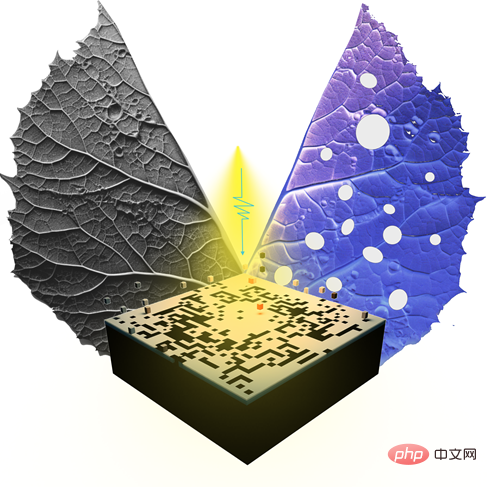
Editor | Dead Leaf Butterfly
Recently, the team of Associate Professor Wu Liang and Academician Yao Jianquan of the Institute of Laser and Optoelectronics of Tianjin University and the team of Professor Xiong Deyi of the Natural Language Processing Laboratory reported A scheme to enhance spectral predictions using deep learning models with multi-frequency supplementary inputs. This scheme can improve the accuracy of spectral prediction by using multi-frequency input data. In addition, this solution can also reduce noise interference in the spectrum prediction process, thereby improving the prediction effect.
This solution can improve the utilization of existing optical data sets and enhance the prediction effect of the spectral response corresponding to the metasurface structure without increasing the training cost.
The relevant research results were titled "Enhanced spectrum prediction using deep learning models with multi-frequency supplementary inputs" and were published in "APL Machine Learning" on May 16, 2024 》.
Paper link: https://doi.org/10.1063/5.0203931
Research background
In recent years, the rapid development of deep learning technology has brought unprecedented changes and innovations to various fields, and has become an effective tool for processing complex and huge data in multiple disciplines.
Methods based on neural networks can effectively detect relevant features and potential patterns of target data, but there are still certain challenges if the deep learning model directly learns these related data from different fields and different formats. To solve this problem, feature extraction techniques can be used. Feature extraction techniques can transform raw data into a representation suitable for a specific task. Different feature extraction methods can be used, such as FFT based on frequency domain analysis, WT based on wavelet transform, etc. By applying these technologies, different fields can be combined.
In recent years, research fields that combine deep learning technology have generally faced problems such as the small size and low quality of existing data sets, which has affected the model's ability to perform target tasks. learning result.
In the entire "AI for Science" research process, the most expensive part is mainly the construction of data sets. Therefore, how to use existing data sets more effectively is crucial.
Research by the Tianjin University team has proven that adding supplementary multi-frequency input information to the existing data set during the target spectrum prediction process can significantly improve the prediction accuracy of the network. This approach provides new ideas for using data sets for interdisciplinary research and applications in deep learning and other fields such as photonics, composite material design, and biomedicine.
Research Highlights
The innovative point of the research is to propose the idea of splitting spectral information in the full frequency range, which is manifested in combining the actual design requirements and dividing the full-frequency spectral information according to The learning tasks are split into the working frequency part and the non-working frequency part.
In order to demonstrate the universality of this solution, the target operating frequency band was refined into a low-frequency information (0-1 THz) part and a high-frequency information (1-2 THz) part to demonstrate the enhancement of model learning. Effect.
Compared with direct prediction of the working frequency range data, after supplementing other frequency information, the overall transmission spectrum data prediction error dropped by about 80%. Among them, the Transformer-based model after supplementing the low-frequency information , the prediction error is only about 40% of the direct prediction. The designed metasurface structure and model architecture are shown in Figure 1:
In order to more intuitively demonstrate the prediction effect of optimized amplitude and phase parameters at different operating frequencies, some metasurface structures are randomly selected for simulation demonstration in CST Studio Suite software, as shown in Figure 2:
Figure 2 Schematic diagram of the prediction effect of optimized high-frequency and low-frequency data. (a)-(f) Demonstrate the different prediction performance of the optimized network model in different frequency ranges by comparing real data (purple solid line) with predicted data (black dashed line). Green areas represent frequency information data used as supplementary input, while yellow areas represent areas used to validate optimized prediction performance. where a and b represent the prediction results of the high-frequency and low-frequency amplitudes of the x-polarization state. (c)-(d) Prediction results of high-frequency and low-frequency amplitudes of y-polarization state. (e)-(f) Prediction results of high-frequency and low-frequency phases.
Summary and Outlook
This research effectively improves the utilization efficiency of existing data sets by conducting targeted data set splitting for learning tasks of different optical problems. This further improves the learning effect of the deep learning model.
This optimization solution effectively alleviates the problem of small existing optical data sets (especially related data sets in the terahertz band), and also provides more research areas that combine deep learning technology but have expensive data, such as Composites design, medical image analysis, financial data prediction, and more provide a new perspective on optimizing data sets.
The above is the detailed content of To improve the utilization of optical data sets, the Tianda team proposed an AI model to enhance spectral prediction effects. For more information, please follow other related articles on the PHP Chinese website!

Hot AI Tools

Undresser.AI Undress
AI-powered app for creating realistic nude photos

AI Clothes Remover
Online AI tool for removing clothes from photos.

Undress AI Tool
Undress images for free

Clothoff.io
AI clothes remover

Video Face Swap
Swap faces in any video effortlessly with our completely free AI face swap tool!

Hot Article

Hot Tools

Notepad++7.3.1
Easy-to-use and free code editor

SublimeText3 Chinese version
Chinese version, very easy to use

Zend Studio 13.0.1
Powerful PHP integrated development environment

Dreamweaver CS6
Visual web development tools

SublimeText3 Mac version
God-level code editing software (SublimeText3)

Hot Topics










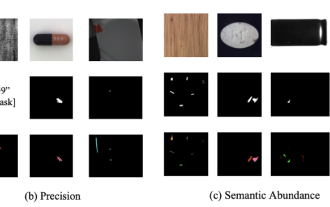
In modern manufacturing, accurate defect detection is not only the key to ensuring product quality, but also the core of improving production efficiency. However, existing defect detection datasets often lack the accuracy and semantic richness required for practical applications, resulting in models unable to identify specific defect categories or locations. In order to solve this problem, a top research team composed of Hong Kong University of Science and Technology Guangzhou and Simou Technology innovatively developed the "DefectSpectrum" data set, which provides detailed and semantically rich large-scale annotation of industrial defects. As shown in Table 1, compared with other industrial data sets, the "DefectSpectrum" data set provides the most defect annotations (5438 defect samples) and the most detailed defect classification (125 defect categories
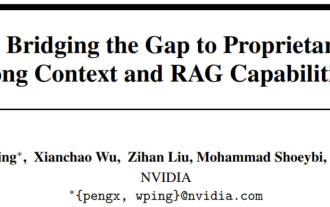
The open LLM community is an era when a hundred flowers bloom and compete. You can see Llama-3-70B-Instruct, QWen2-72B-Instruct, Nemotron-4-340B-Instruct, Mixtral-8x22BInstruct-v0.1 and many other excellent performers. Model. However, compared with proprietary large models represented by GPT-4-Turbo, open models still have significant gaps in many fields. In addition to general models, some open models that specialize in key areas have been developed, such as DeepSeek-Coder-V2 for programming and mathematics, and InternVL for visual-language tasks.
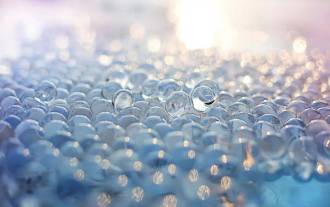
Editor |KX To this day, the structural detail and precision determined by crystallography, from simple metals to large membrane proteins, are unmatched by any other method. However, the biggest challenge, the so-called phase problem, remains retrieving phase information from experimentally determined amplitudes. Researchers at the University of Copenhagen in Denmark have developed a deep learning method called PhAI to solve crystal phase problems. A deep learning neural network trained using millions of artificial crystal structures and their corresponding synthetic diffraction data can generate accurate electron density maps. The study shows that this deep learning-based ab initio structural solution method can solve the phase problem at a resolution of only 2 Angstroms, which is equivalent to only 10% to 20% of the data available at atomic resolution, while traditional ab initio Calculation
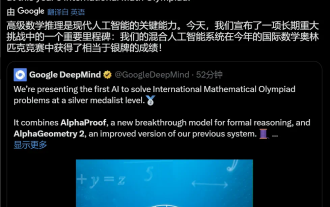
For AI, Mathematical Olympiad is no longer a problem. On Thursday, Google DeepMind's artificial intelligence completed a feat: using AI to solve the real question of this year's International Mathematical Olympiad IMO, and it was just one step away from winning the gold medal. The IMO competition that just ended last week had six questions involving algebra, combinatorics, geometry and number theory. The hybrid AI system proposed by Google got four questions right and scored 28 points, reaching the silver medal level. Earlier this month, UCLA tenured professor Terence Tao had just promoted the AI Mathematical Olympiad (AIMO Progress Award) with a million-dollar prize. Unexpectedly, the level of AI problem solving had improved to this level before July. Do the questions simultaneously on IMO. The most difficult thing to do correctly is IMO, which has the longest history, the largest scale, and the most negative
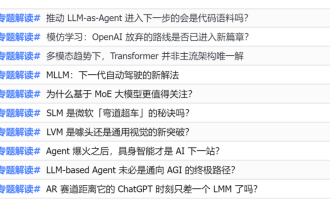
In 2023, almost every field of AI is evolving at an unprecedented speed. At the same time, AI is constantly pushing the technological boundaries of key tracks such as embodied intelligence and autonomous driving. Under the multi-modal trend, will the situation of Transformer as the mainstream architecture of AI large models be shaken? Why has exploring large models based on MoE (Mixed of Experts) architecture become a new trend in the industry? Can Large Vision Models (LVM) become a new breakthrough in general vision? ...From the 2023 PRO member newsletter of this site released in the past six months, we have selected 10 special interpretations that provide in-depth analysis of technological trends and industrial changes in the above fields to help you achieve your goals in the new year. be prepared. This interpretation comes from Week50 2023
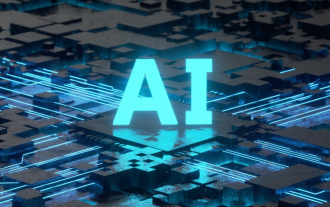
Editor |ScienceAI Question Answering (QA) data set plays a vital role in promoting natural language processing (NLP) research. High-quality QA data sets can not only be used to fine-tune models, but also effectively evaluate the capabilities of large language models (LLM), especially the ability to understand and reason about scientific knowledge. Although there are currently many scientific QA data sets covering medicine, chemistry, biology and other fields, these data sets still have some shortcomings. First, the data form is relatively simple, most of which are multiple-choice questions. They are easy to evaluate, but limit the model's answer selection range and cannot fully test the model's ability to answer scientific questions. In contrast, open-ended Q&A

Editor | KX Retrosynthesis is a critical task in drug discovery and organic synthesis, and AI is increasingly used to speed up the process. Existing AI methods have unsatisfactory performance and limited diversity. In practice, chemical reactions often cause local molecular changes, with considerable overlap between reactants and products. Inspired by this, Hou Tingjun's team at Zhejiang University proposed to redefine single-step retrosynthetic prediction as a molecular string editing task, iteratively refining the target molecular string to generate precursor compounds. And an editing-based retrosynthetic model EditRetro is proposed, which can achieve high-quality and diverse predictions. Extensive experiments show that the model achieves excellent performance on the standard benchmark data set USPTO-50 K, with a top-1 accuracy of 60.8%.
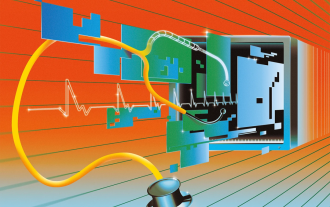
Editor | ScienceAI Based on limited clinical data, hundreds of medical algorithms have been approved. Scientists are debating who should test the tools and how best to do so. Devin Singh witnessed a pediatric patient in the emergency room suffer cardiac arrest while waiting for treatment for a long time, which prompted him to explore the application of AI to shorten wait times. Using triage data from SickKids emergency rooms, Singh and colleagues built a series of AI models that provide potential diagnoses and recommend tests. One study showed that these models can speed up doctor visits by 22.3%, speeding up the processing of results by nearly 3 hours per patient requiring a medical test. However, the success of artificial intelligence algorithms in research only verifies this
