The AIxiv column is a column where this site publishes academic and technical content. In the past few years, the AIxiv column of this site has received more than 2,000 reports, covering top laboratories from major universities and companies around the world, effectively promoting academic exchanges and dissemination. If you have excellent work that you want to share, please feel free to contribute or contact us for reporting. Submission email: liyazhou@jiqizhixin.com; zhaoyunfeng@jiqizhixin.com
##Richard Sutton made this evaluation in "The Bitter Lesson": "The most important lesson that can be drawn from 70 years of artificial intelligence research is that those general methods that exploit computing are ultimately the most effective, and the advantages are huge."
Self play is such a method that uses search and learning at the same time to fully utilize and expand the scale of computing.
At the beginning of this year, Professor Gu Quanquan’s team at the University of California, Los Angeles (UCLA) proposed a
Self-Play Fine-Tuning, SPIN ), without using additional fine-tuning data, the ability of LLM can be greatly improved by relying on self-game alone. Recently, Professor Gu Quanquan’s team and Professor Yiming Yang’s team at Carnegie Mellon University (CMU) collaborated to develop a method called “
Self-Game Preference Optimization (Self-Play Preference Optimization, SPPO) " alignment technology, this new method aims to optimize the behavior of large language models through a self-game framework to better match human preferences. Fight each other from left to right and show off your magical powers again! ##Paper title: Self-Play Preference Optimization for Language Model AlignmentPaper link: https://arxiv.org/pdf/2405.00675.pdf
##Technical background and challengesLarge language models (LLMs) are becoming an important driving force in the field of artificial intelligence, performing well in various tasks with their excellent text generation and understanding capabilities. Although the capabilities of LLM are impressive, making the output behavior of these models more consistent with the needs of practical applications often requires fine-tuning through an alignment process.
#The key to this process is to adjust the model to better reflect human preferences and behavioral norms. Common methods include reinforcement learning based on human feedback (RLHF) or direct preference optimization (Direct Preference Optimization, DPO).
Reinforcement learning based on human feedback (RLHF) relies on explicitly maintaining a reward model to adjust and refine large language models. In other words, for example, InstructGPT first trains a reward function that obeys the Bradley-Terry model based on human preference data, and then uses reinforcement learning algorithms like Proximal Policy Optimization (PPO) to optimize large language models. Last year, researchers proposed Direct Preference Optimization (DPO).
Unlike RLHF, which maintains an explicit reward model, the DPO algorithm implicitly obeys the Bradley-Terry model, but can be directly used for large language model optimization. Existing work has attempted to further fine-tune large models by using DPO over multiple iterations (Figure 1).
## Figure 1. The iterative optimization method based on the Bradley-Terry model lacks theoretical understanding and guarantee
Parametric models such as Bradley-Terry provide a numerical score for each choice. While these models provide reasonable approximations of human preferences, they fail to fully capture the complexity of human behavior.
These models often assume that the preference relationship between different options is monotonic and transitive, while empirical evidence often shows the inconsistency and nonlinearity of human decision-making, such as Tversky's research observed that human decision-making can be influenced by multiple factors and exhibit inconsistencies.
Theoretical basis and method of SPPO
2. The two language models of imagination are often played. In these contexts, the author proposes a new self-game framework SPPO, which not only has the ability to solve the problem of two players It is provably guaranteed for two-player constant-sum games and can be extended to efficiently fine-tune large language models on a large scale. Specifically, the article strictly defines the RLHF problem as a two-player normal-sum game (Figure 2). The goal of this work is to identify Nash equilibrium strategies that, on average, always provide a more preferred response than any other strategy. In order to approximately identify the Nash equilibrium strategy, the author adopts the classic online adaptive algorithm with multiplicative weights as a high-level framework algorithm to solve the two-player game. Within each step of this framework, the algorithm can approximate multiplicative weight updates through a self-game mechanism, where in each round, the large language model is working on the previous The wheel itself is fine-tuned, optimized through synthetic data generated by the model and annotations of preferred models. Specifically, the large language model will generate several responses for each prompt in each round; based on the annotations of the preference model, the algorithm can estimate the winning rate of each response ;The algorithm can further fine-tune the parameters of the large language model so that responses with a high winning rate have a higher probability of appearing (Figure 3). # Figure 3. The goal of the self-game algorithm is to fine-tune itself to outperform the previous round of language model Experimental design and resultsIn the experiment, the research team used A Mistral-7B is used as the baseline model and 60,000 prompts from the UltraFeedback dataset are used for unsupervised training. They found that through self-playing, the model was able to significantly improve its performance on multiple evaluation platforms, such as AlpacaEval 2.0 and MT-Bench. These platforms are widely used to evaluate the quality and relevance of model-generated text.
Through the SPPO method, the model is not only improved in
the fluency and accuracy of generated text, but more importantly is: "It performs better in conforming to human values and preferences." 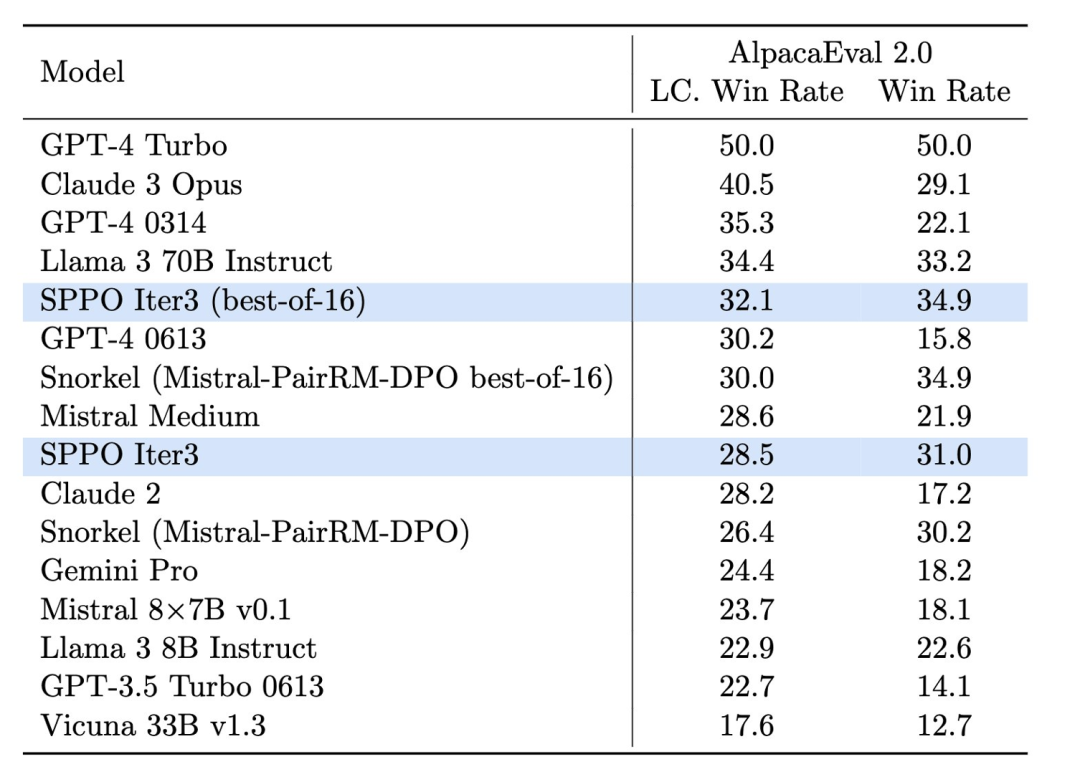
## 图 4. The effect of the Sppo model on Alpacaeval 2.0 is significantly improved, which is higher than other benchmark methods such as Iterative DPO. In the test of AlpacaEval 2.0 (Figure 4), the length control winning rate of the SPPO-optimized model increased from 17.11% of the baseline model to 28.53%, showing a significant improvement in its understanding of human preferences. The model optimized by three rounds of SPPO is significantly better than the multi-round iteration of DPO, IPO and self-rewarding language model (Self-Rewarding LM) on AlpacaEval2.0. In addition, the model’s performance on MT-Bench also exceeded that of traditional models tuned through human feedback. This demonstrates the effectiveness of SPPO in automatically adapting model behavior to complex tasks. Conclusion and future prospectsSelf-playing preference optimization (SPPO) is the big language The model provides a new optimization path, which not only improves the quality of model generation, but more importantly, improves the alignment of the model with human preferences. With the continuous development and optimization of technology, it is expected that SPPO and its derivative technologies will play a greater role in the sustainable development and social application of artificial intelligence, building a Paving the way for more intelligent and responsible AI systems. The above is the detailed content of Human preference is the ruler! SPPO alignment technology allows large language models to compete with each other and compete with themselves. For more information, please follow other related articles on the PHP Chinese website!