


GraphRAG enhanced for knowledge graph retrieval (implemented based on Neo4j code)
图检索增强生成(Graph RAG)正逐渐流行起来,成为传统向量搜索方法的有力补充。这种方法利用图数据库的结构化特性,将数据以节点和关系的形式组织起来,从而增强检索信息的深度和上下文关联性。图在表示和存储多样化且相互关联的信息方面具有天然优势,能够轻松捕捉不同数据类型间的复杂关系和属性。而向量数据库则处理这类结构化信息时则显得力不从心,它们更专注于处理高维向量表示的非结构化数据。在 RAG 应用中,结合结构化化的图数据和非结构化的文本向量搜索,可以让我们同时享受两者的优势,这也是本文将要探讨的内容。
构建知识图谱通常是利用图数据表示的强大功能中最困难的一步。它需要收集和整理数据,这需要对领域知识和图建模有深刻的理解。为了简化这一过程,可以参考已有的项目或者利用LLM来创建知识图谱,进而可以把重点放在检索召回上,以提高LLM的生成阶段。下面来进行相关代码的实践。
1.知识图谱构建
为了存储知识图谱数据,首先需要搭建一个 Neo4j 实例。最简单的方法是在 Neo4j Aura 上启动一个免费实例,它提供了 Neo4j 数据库的云版本。当然,也可以通过 Docker 本地启动一个,然后将图谱数据导入到 Neo4j 数据库中。
步骤I:Neo4j环境搭建
下面是本地启动docker的运行示例:
docker run -d \--restart always \--publish=7474:7474 --publish=7687:7687 \--env NEO4J_AUTH=neo4j/000000 \--volume=/yourdockerVolume/neo4j:/data \neo4j:5.19.0
步骤II:图谱数据导入
演示中,我们可以使用伊丽莎白一世的维基百科页面。利用 LangChain 加载器从维基百科获取并分割文档,后存入Neo4j数据库。为了试验中文上的效果,我们导入这个Github上的这个项目(QASystemOnMedicalKG)中的医学知识图谱,包含近35000个节点,30万组三元组,大致得到如下结果:
图片
或者利用LangChainLangChain 加载器从维基百科获取并分割文档,大致如下面步骤所示:
# 读取维基百科文章raw_documents = WikipediaLoader(query="Elizabeth I").load()# 定义分块策略text_splitter = TokenTextSplitter(chunk_size=512, chunk_overlap=24)documents = text_splitter.split_documents(raw_documents[:3])llm=ChatOpenAI(temperature=0, model_name="gpt-4-0125-preview")llm_transformer = LLMGraphTransformer(llm=llm)# 提取图数据graph_documents = llm_transformer.convert_to_graph_documents(documents)# 存储到 neo4jgraph.add_graph_documents(graph_documents, baseEntityLabel=True, include_source=True)
2.知识图谱检索
在对知识图谱检索之前,需要对实体和相关属性进行向量嵌入并存储到Neo4j数据库中:
- 实体信息向量嵌入:将实体名称和实体的描述信息拼接后,利用向量表征模型进行向量嵌入(如下述示例代码中的add_embeddings方法所示)。
- 图谱结构化检索:图谱的结构化检索分为四个步骤:步骤一,从图谱中检索与查询相关的实体;步骤二,从全局索引中检索得到实体的标签;步骤三,根据实体标签在相应的节点中查询邻居节点路径;步骤四,对关系进行筛选,保持多样性(整个检索过程如下述示例代码中的structured_retriever方法所示)。
class GraphRag(object):def __init__(self):"""Any embedding function implementing `langchain.embeddings.base.Embeddings` interface."""self._database = 'neo4j'self.label = 'Med'self._driver = neo4j.GraphDatabase.driver(uri=os.environ["NEO4J_URI"],auth=(os.environ["NEO4J_USERNAME"],os.environ["NEO4J_PASSWORD"]))self.embeddings_zh = HuggingFaceEmbeddings(model_name=os.environ["EMBEDDING_MODEL"])self.vectstore = Neo4jVector(embedding=self.embeddings_zh, username=os.environ["NEO4J_USERNAME"], password=os.environ["NEO4J_PASSWORD"], url=os.environ["NEO4J_URI"], node_label=self.label, index_name="vector" )def query(self, query: str, params: dict = {}) -> List[Dict[str, Any]]:"""Query Neo4j database."""from neo4j.exceptions import CypherSyntaxErrorwith self._driver.session(database=self._database) as session:try:data = session.run(query, params)return [r.data() for r in data]except CypherSyntaxError as e:raise ValueError(f"Generated Cypher Statement is not valid\n{e}")def add_embeddings(self):"""Add embeddings to Neo4j database."""# 查询图中所有节点,并且根据节点的描述和名字生成embedding,添加到该节点上query = """MATCH (n) WHERE not (n:{}) RETURN ID(n) AS id, labels(n) as labels, n""".format(self.label)print("qurey node...")data = self.query(query)ids, texts, embeddings, metas = [], [], [], []for row in tqdm(data,desc="parsing node"):ids.append(row['id'])text = row['n'].get('name','') + row['n'].get('desc','')texts.append(text)metas.append({"label": row['labels'], "context": text})self.embeddings_zh.multi_process = Falseprint("node embeddings...")embeddings = self.embeddings_zh.embed_documents(texts)print("adding node embeddings...")ids_ret = self.vectstore.add_embeddings(ids=ids,texts=texts,embeddings=embeddings,metadatas=metas)return ids_ret# Fulltext index querydef structured_retriever(self, query, limit=3, simlarity=0.9) -> str:"""Collects the neighborhood of entities mentioned in the question"""# step1 从图谱中检索与查询相关的实体。docs_with_score = self.vectstore.similarity_search_with_score(query, k=topk)entities = [item[0].page_content for item in data if item[1] > simlarity] # scoreself.vectstore.query("CREATE FULLTEXT INDEX entity IF NOT EXISTS FOR (e:Med) ON EACH [e.context]")result = ""for entity in entities:qry = entity# step2 从全局索引中查出entity label,query1 =f"""CALL db.index.fulltext.queryNodes('entity', '{qry}') YIELD node, score return node.label as label,node.context as context, node.id as id, score LIMIT {limit}"""data1 = self.vectstore.query(query1)# step3 根据label在相应的节点中查询邻居节点路径for item in data1:node_type = item['label']node_type = item['label'] if type(node_type) == str else node_type[0]node_id = item['id']query2 = f"""match (node:{node_type})-[r]-(neighbor) where ID(node) = {node_id} RETURN type(r) as rel, node.name+' - '+type(r)+' - '+neighbor.name as output limit 50"""data2 = self.vectstore.query(query2)# step4 为了保持多样性,对关系进行筛选rel_dict = defaultdict(list)if len(data2) > 3*limit:for item1 in data2:rel_dict[item1['rel']].append(item1['output'])if rel_dict:rel_dict = {k:random.sample(v, 3) if len(v)>3 else v for k,v in rel_dict.items()}result += "\n".join(['\n'.join(el) for el in rel_dict.values()]) +'\n'else:result += "\n".join([el['output'] for el in data2]) +'\n'return result
3.结合LLM生成
最后利用大语言模型(LLM)根据从知识图谱中检索出来的结构化信息,生成最终的回复。下面的代码中我们以通义千问开源的大语言模型为例:
步骤I:加载LLM模型
from langchain import HuggingFacePipelinefrom transformers import pipeline, AutoTokenizer, AutoModelForCausalLMdef custom_model(model_name, branch_name=None, cache_dir=None, temperature=0, top_p=1, max_new_tokens=512, stream=False):tokenizer = AutoTokenizer.from_pretrained(model_name, revision=branch_name, cache_dir=cache_dir)model = AutoModelForCausalLM.from_pretrained(model_name,device_map='auto',torch_dtype=torch.float16,revision=branch_name,cache_dir=cache_dir)pipe = pipeline("text-generation",model = model,tokenizer = tokenizer,torch_dtype = torch.bfloat16,device_map = 'auto',max_new_tokens = max_new_tokens,do_sample = True)llm = HuggingFacePipeline(pipeline = pipe,model_kwargs = {"temperature":temperature, "top_p":top_p,"tokenizer":tokenizer, "model":model})return llmtongyi_model = "Qwen1.5-7B-Chat"llm_model = custom_model(model_name=tongyi_model)tokenizer = llm_model.model_kwargs['tokenizer']model = llm_model.model_kwargs['model']
步骤II:输入检索数据生成回复
final_data = self.get_retrieval_data(query)prompt = ("请结合以下信息,简洁和专业的来回答用户的问题,若信息与问题关联紧密,请尽量参考已知信息。\n""已知相关信息:\n{context} 请回答以下问题:{question}".format(cnotallow=final_data, questinotallow=query))messages = [{"role": "system", "content": "你是**开发的智能助手。"},{"role": "user", "content": prompt}]text = tokenizer.apply_chat_template(messages, tokenize=False, add_generation_prompt=True)model_inputs = tokenizer([text], return_tensors="pt").to(self.device)generated_ids = model.generate(model_inputs.input_ids,max_new_tokens=512)generated_ids = [output_ids[len(input_ids):] for input_ids, output_ids in zip(model_inputs.input_ids, generated_ids)]response = tokenizer.batch_decode(generated_ids, skip_special_tokens=True)[0]print(response)
4 结语
对一个查询问题分别进行了测试, 与没有RAG仅利用LLM生成回复的的情况进行对比,在有GraphRAG 的情况下,LLM模型回答的信息量更大、准确会更高。
The above is the detailed content of GraphRAG enhanced for knowledge graph retrieval (implemented based on Neo4j code). For more information, please follow other related articles on the PHP Chinese website!

Hot AI Tools

Undresser.AI Undress
AI-powered app for creating realistic nude photos

AI Clothes Remover
Online AI tool for removing clothes from photos.

Undress AI Tool
Undress images for free

Clothoff.io
AI clothes remover

Video Face Swap
Swap faces in any video effortlessly with our completely free AI face swap tool!

Hot Article

Hot Tools

Notepad++7.3.1
Easy-to-use and free code editor

SublimeText3 Chinese version
Chinese version, very easy to use

Zend Studio 13.0.1
Powerful PHP integrated development environment

Dreamweaver CS6
Visual web development tools

SublimeText3 Mac version
God-level code editing software (SublimeText3)

Hot Topics










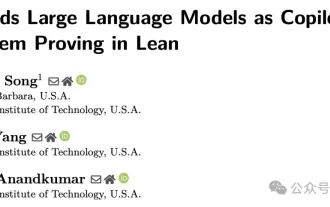
LeanCopilot, this formal mathematics tool that has been praised by many mathematicians such as Terence Tao, has evolved again? Just now, Caltech professor Anima Anandkumar announced that the team released an expanded version of the LeanCopilot paper and updated the code base. Image paper address: https://arxiv.org/pdf/2404.12534.pdf The latest experiments show that this Copilot tool can automate more than 80% of the mathematical proof steps! This record is 2.3 times better than the previous baseline aesop. And, as before, it's open source under the MIT license. In the picture, he is Song Peiyang, a Chinese boy. He is
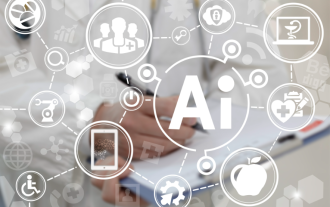
Translator | Bugatti Review | Chonglou This article describes how to use the GroqLPU inference engine to generate ultra-fast responses in JanAI and VSCode. Everyone is working on building better large language models (LLMs), such as Groq focusing on the infrastructure side of AI. Rapid response from these large models is key to ensuring that these large models respond more quickly. This tutorial will introduce the GroqLPU parsing engine and how to access it locally on your laptop using the API and JanAI. This article will also integrate it into VSCode to help us generate code, refactor code, enter documentation and generate test units. This article will create our own artificial intelligence programming assistant for free. Introduction to GroqLPU inference engine Groq
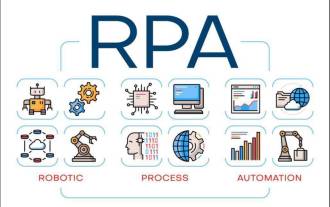
Image source@visualchinesewen|Wang Jiwei From "human + RPA" to "human + generative AI + RPA", how does LLM affect RPA human-computer interaction? From another perspective, how does LLM affect RPA from the perspective of human-computer interaction? RPA, which affects human-computer interaction in program development and process automation, will now also be changed by LLM? How does LLM affect human-computer interaction? How does generative AI change RPA human-computer interaction? Learn more about it in one article: The era of large models is coming, and generative AI based on LLM is rapidly transforming RPA human-computer interaction; generative AI redefines human-computer interaction, and LLM is affecting the changes in RPA software architecture. If you ask what contribution RPA has to program development and automation, one of the answers is that it has changed human-computer interaction (HCI, h
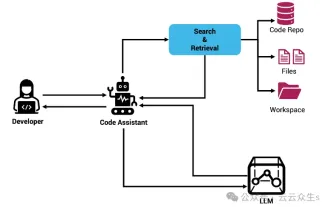
Improve developer productivity, efficiency, and accuracy by incorporating retrieval-enhanced generation and semantic memory into AI coding assistants. Translated from EnhancingAICodingAssistantswithContextUsingRAGandSEM-RAG, author JanakiramMSV. While basic AI programming assistants are naturally helpful, they often fail to provide the most relevant and correct code suggestions because they rely on a general understanding of the software language and the most common patterns of writing software. The code generated by these coding assistants is suitable for solving the problems they are responsible for solving, but often does not conform to the coding standards, conventions and styles of the individual teams. This often results in suggestions that need to be modified or refined in order for the code to be accepted into the application
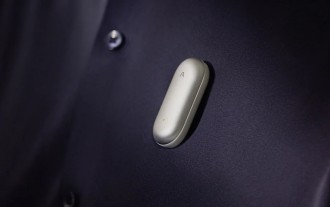
Plaud, the company behind the Plaud Note AI Voice Recorder (available on Amazon for $159), has announced a new product. Dubbed the NotePin, the device is described as an AI memory capsule, and like the Humane AI Pin, this is wearable. The NotePin is

To learn more about AIGC, please visit: 51CTOAI.x Community https://www.51cto.com/aigc/Translator|Jingyan Reviewer|Chonglou is different from the traditional question bank that can be seen everywhere on the Internet. These questions It requires thinking outside the box. Large Language Models (LLMs) are increasingly important in the fields of data science, generative artificial intelligence (GenAI), and artificial intelligence. These complex algorithms enhance human skills and drive efficiency and innovation in many industries, becoming the key for companies to remain competitive. LLM has a wide range of applications. It can be used in fields such as natural language processing, text generation, speech recognition and recommendation systems. By learning from large amounts of data, LLM is able to generate text
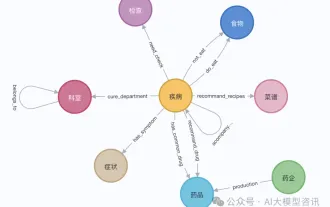
Graph Retrieval Enhanced Generation (GraphRAG) is gradually becoming popular and has become a powerful complement to traditional vector search methods. This method takes advantage of the structural characteristics of graph databases to organize data in the form of nodes and relationships, thereby enhancing the depth and contextual relevance of retrieved information. Graphs have natural advantages in representing and storing diverse and interrelated information, and can easily capture complex relationships and properties between different data types. Vector databases are unable to handle this type of structured information, and they focus more on processing unstructured data represented by high-dimensional vectors. In RAG applications, combining structured graph data and unstructured text vector search allows us to enjoy the advantages of both at the same time, which is what this article will discuss. structure
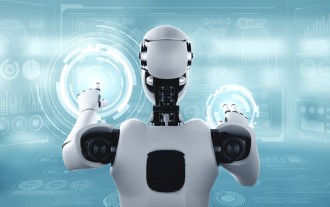
As the performance of open source large-scale language models continues to improve, performance in writing and analyzing code, recommendations, text summarization, and question-answering (QA) pairs has all improved. But when it comes to QA, LLM often falls short on issues related to untrained data, and many internal documents are kept within the company to ensure compliance, trade secrets, or privacy. When these documents are queried, LLM can hallucinate and produce irrelevant, fabricated, or inconsistent content. One possible technique to handle this challenge is Retrieval Augmented Generation (RAG). It involves the process of enhancing responses by referencing authoritative knowledge bases beyond the training data source to improve the quality and accuracy of the generation. The RAG system includes a retrieval system for retrieving relevant document fragments from the corpus
