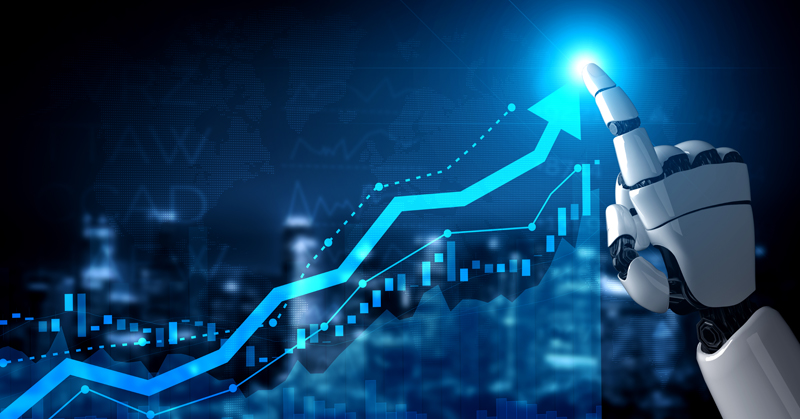
GenAI remains the top
investmentfocus for most enterprises, and
expectationsare high. According to the latest PwC survey, 61% of CEOs in the United States expect
AI to change the way their business generates value, but to achieve this goal, companies must turn AI hype into reality. The good news is, they're getting better at it. In fact, according to the results of Databricks’ recently released State of Data + AI Report, companies pushed 1,342% of their models from the experimental stage into the real world, and their ambitions for data and AI have not diminished, and the number of experimental models has also An increase of 134%. These are encouraging signs, but based on my conversations with CIOs and other technology leaders, the challenge now is maintaining this momentum. Companies face difficulties in agreeing on expectations
. Naturally, many executives want to see an immediate return on their investment
, and companies are not thinking about long-term strategies out of fear that competitors are making faster progress in AI. This approach is wrong. Companies that focus only on the “now” will find themselves jumping from one novelty to the next. Investment
in GenAI is a long process and CIOs must shift their company’s AI mindset from short-term wins to long-term business transformation. With that in mind, here are three things CIOs and other technology leaders should focus on as they address these challenges. Key Areas: 1. Improving Data Infrastructure Priorities and areas of focus for AI are evolving almost as rapidly as the technology itself. For example, six months ago, few CIOs were familiar with Retrieval Augmented Generation (RAG). Now, training commercial LLM on private company data to achieve better and more customized performance has become an important area of focus. According to our anonymized usage data, usage of vector databases, which are key to building RAG models, has grown 377% over the past year. Some companies, like Databricks, are even starting to use an “agents
and tools” approach to help AI systems solve more complex problems. This is a testament to the speed of GenAI's development and the rapid expansion of its capabilities, but data migration remains a challenge. For example, to build a successful RAG system, the data needed for training must be readily available and not trapped in silos across the enterprise. Both structured and unstructured data need to come together to ensure that the model can provide the customized answers that users expect
. But this is only a challenge today, businesses need a framework that can scale as needs and technology change. Leaders should ask themselves the following questions: What infrastructure will allow us to effectively manage, maintain and derive insights from data, no matter how the larger AI environment evolves? At what types or scales of models do we want to use the applications? How does our data environment need to improve or change to work well with these models? 2. Navigate the basic model For most enterprises, the general LLM is a good starting point. We also continue to see an increase in usage on the platform. But the more companies use these basic models, the more they discover their limitations. This is why many enterprises are now looking to techniques such as RAG and fine-tuning to improve the performance of their models and ultimately reduce their running costs. While companies are doing this with proprietary models, they are increasingly choosing open systems. In fact, just weeks after Llama3 was released, it accounted for 39% of all open source model usage, according to findings in our report. As these technologies become more prevalent, businesses need to learn when to apply which technology. For example, businesses may decide to build their own models from scratch when it might be more cost-effective to build their own models, instead just fine-tuning a base model, or they may be working with ever-updating sets of information. In this case, RAG is a better choice because the enterprise can quickly feed new data to the model. Importantly, models are only getting better. It’s crucial to keep a keen eye on future trends. 3. Prioritize accuracy in AI development and use AI is changing workflows and reshaping the way businesses think about data, but many companies are still in the testing and experimentation phase because, currently, many models don’t work as well as they need to. as accurate or reliable. Some companies are already seeing the consequences: an airline's chatbot offers a refund that doesn't comply with the company's official policy, a court ruling sides with a customer, or consider a car dealership's chatbot offering a customer a car for a dollar. If these lapses occur at scale or in high-risk scenarios, businesses will suffer, which is why keeping humans in the loop is critical, especially for applications that require high accuracy. Even as models improve, the quality of their outputs depends on the data that supports them. Strong data hygiene and oversight are key to ensuring enterprises achieve AI-driven success. Ensuring data is managed, accessible and well-structured will help businesses see more consistent and reliable output in the future.AI will increasingly be able to augment human capabilities in workplaces such as healthcare, manufacturing, enterprise technology, and more, which is why businesses need to focus on maintaining strong data infrastructure and practices, prioritizing AI applications that align with their broader business strategies, and cultivating An AI-ready workforce prepared for this era of change. The above is the detailed content of Areas CIOs should focus on to maintain GenAI's momentum. For more information, please follow other related articles on the PHP Chinese website!