


Eight times stronger than the original material, teams from Tsinghua University and Wuhan Institute of Technology used AI to screen high-entropy dielectric materials
component in capacitors. They are widely used in hybrid electric vehicles, portable electronic devices, and pulsed power systems due to their high power density and fast response characteristics. Additionally, dielectric materials are critical in modern electronic and power systems, supporting the miniaturization
.
structure and electrical property analysis. (Source: paper) Then perform probability sampling on the existing small sample data, retain two decimal places for the element content of positions A and B, and set the sum of each position to 1 to construct a possibility of close to 10^11 combinations.
Graphic: Energy storage performance and cycle stability. (Source: Paper) Experimental results show that the prepared high-entropy ceramic dielectric film is significantly better than traditional materials in terms of energy density and breakdown strength, especially the C-3 film under the electric field of 5104 kV/cm The energy density reaches 156 J/cm^3, which is eight times that of the original BMT (~18 J/cm^3) film. In addition, the study also explored the effects of different annealing temperatures on the performance of high-entropy films, and found that appropriate annealing temperatures can further improve the energy storage performance of the material. In summary, high-entropy films not only have excellent fatigue performance and temperature and frequency stability, but also show great potential for widespread applications in energy storage capacitors. Based on a machine learning-driven paradigm, the team leveraged very sparse experimental data to efficiently find the desired high-entropy composites with high energy storage properties. The method also allows researchers to significantly shorten the overall experimental cycle and opens up new avenues for designing material systems with complex components. Paper link: https://www.nature.com/articles/s41467-024-49170-8
The above is the detailed content of Eight times stronger than the original material, teams from Tsinghua University and Wuhan Institute of Technology used AI to screen high-entropy dielectric materials. For more information, please follow other related articles on the PHP Chinese website!

Hot AI Tools

Undresser.AI Undress
AI-powered app for creating realistic nude photos

AI Clothes Remover
Online AI tool for removing clothes from photos.

Undress AI Tool
Undress images for free

Clothoff.io
AI clothes remover

Video Face Swap
Swap faces in any video effortlessly with our completely free AI face swap tool!

Hot Article

Hot Tools

Notepad++7.3.1
Easy-to-use and free code editor

SublimeText3 Chinese version
Chinese version, very easy to use

Zend Studio 13.0.1
Powerful PHP integrated development environment

Dreamweaver CS6
Visual web development tools

SublimeText3 Mac version
God-level code editing software (SublimeText3)

Hot Topics










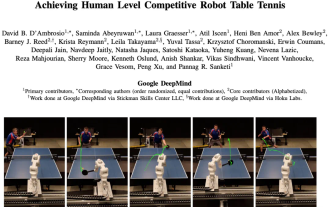
But maybe he can’t defeat the old man in the park? The Paris Olympic Games are in full swing, and table tennis has attracted much attention. At the same time, robots have also made new breakthroughs in playing table tennis. Just now, DeepMind proposed the first learning robot agent that can reach the level of human amateur players in competitive table tennis. Paper address: https://arxiv.org/pdf/2408.03906 How good is the DeepMind robot at playing table tennis? Probably on par with human amateur players: both forehand and backhand: the opponent uses a variety of playing styles, and the robot can also withstand: receiving serves with different spins: However, the intensity of the game does not seem to be as intense as the old man in the park. For robots, table tennis
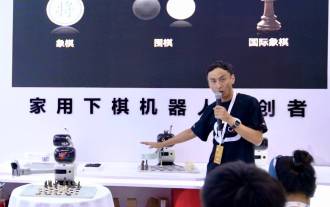
On August 21, the 2024 World Robot Conference was grandly held in Beijing. SenseTime's home robot brand "Yuanluobot SenseRobot" has unveiled its entire family of products, and recently released the Yuanluobot AI chess-playing robot - Chess Professional Edition (hereinafter referred to as "Yuanluobot SenseRobot"), becoming the world's first A chess robot for the home. As the third chess-playing robot product of Yuanluobo, the new Guoxiang robot has undergone a large number of special technical upgrades and innovations in AI and engineering machinery. For the first time, it has realized the ability to pick up three-dimensional chess pieces through mechanical claws on a home robot, and perform human-machine Functions such as chess playing, everyone playing chess, notation review, etc.
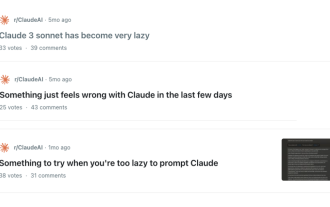
The start of school is about to begin, and it’s not just the students who are about to start the new semester who should take care of themselves, but also the large AI models. Some time ago, Reddit was filled with netizens complaining that Claude was getting lazy. "Its level has dropped a lot, it often pauses, and even the output becomes very short. In the first week of release, it could translate a full 4-page document at once, but now it can't even output half a page!" https:// www.reddit.com/r/ClaudeAI/comments/1by8rw8/something_just_feels_wrong_with_claude_in_the/ in a post titled "Totally disappointed with Claude", full of
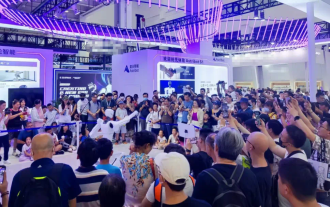
At the World Robot Conference being held in Beijing, the display of humanoid robots has become the absolute focus of the scene. At the Stardust Intelligent booth, the AI robot assistant S1 performed three major performances of dulcimer, martial arts, and calligraphy in one exhibition area, capable of both literary and martial arts. , attracted a large number of professional audiences and media. The elegant playing on the elastic strings allows the S1 to demonstrate fine operation and absolute control with speed, strength and precision. CCTV News conducted a special report on the imitation learning and intelligent control behind "Calligraphy". Company founder Lai Jie explained that behind the silky movements, the hardware side pursues the best force control and the most human-like body indicators (speed, load) etc.), but on the AI side, the real movement data of people is collected, allowing the robot to become stronger when it encounters a strong situation and learn to evolve quickly. And agile
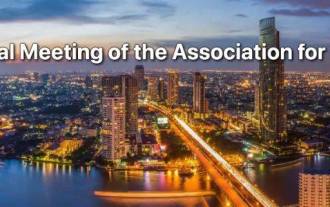
At this ACL conference, contributors have gained a lot. The six-day ACL2024 is being held in Bangkok, Thailand. ACL is the top international conference in the field of computational linguistics and natural language processing. It is organized by the International Association for Computational Linguistics and is held annually. ACL has always ranked first in academic influence in the field of NLP, and it is also a CCF-A recommended conference. This year's ACL conference is the 62nd and has received more than 400 cutting-edge works in the field of NLP. Yesterday afternoon, the conference announced the best paper and other awards. This time, there are 7 Best Paper Awards (two unpublished), 1 Best Theme Paper Award, and 35 Outstanding Paper Awards. The conference also awarded 3 Resource Paper Awards (ResourceAward) and Social Impact Award (

Deep integration of vision and robot learning. When two robot hands work together smoothly to fold clothes, pour tea, and pack shoes, coupled with the 1X humanoid robot NEO that has been making headlines recently, you may have a feeling: we seem to be entering the age of robots. In fact, these silky movements are the product of advanced robotic technology + exquisite frame design + multi-modal large models. We know that useful robots often require complex and exquisite interactions with the environment, and the environment can be represented as constraints in the spatial and temporal domains. For example, if you want a robot to pour tea, the robot first needs to grasp the handle of the teapot and keep it upright without spilling the tea, then move it smoothly until the mouth of the pot is aligned with the mouth of the cup, and then tilt the teapot at a certain angle. . this
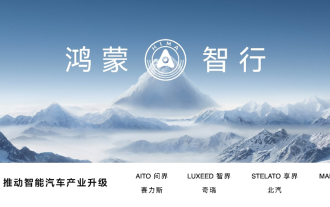
This afternoon, Hongmeng Zhixing officially welcomed new brands and new cars. On August 6, Huawei held the Hongmeng Smart Xingxing S9 and Huawei full-scenario new product launch conference, bringing the panoramic smart flagship sedan Xiangjie S9, the new M7Pro and Huawei novaFlip, MatePad Pro 12.2 inches, the new MatePad Air, Huawei Bisheng With many new all-scenario smart products including the laser printer X1 series, FreeBuds6i, WATCHFIT3 and smart screen S5Pro, from smart travel, smart office to smart wear, Huawei continues to build a full-scenario smart ecosystem to bring consumers a smart experience of the Internet of Everything. Hongmeng Zhixing: In-depth empowerment to promote the upgrading of the smart car industry Huawei joins hands with Chinese automotive industry partners to provide
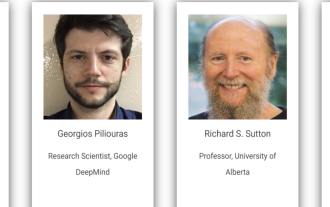
Conference Introduction With the rapid development of science and technology, artificial intelligence has become an important force in promoting social progress. In this era, we are fortunate to witness and participate in the innovation and application of Distributed Artificial Intelligence (DAI). Distributed artificial intelligence is an important branch of the field of artificial intelligence, which has attracted more and more attention in recent years. Agents based on large language models (LLM) have suddenly emerged. By combining the powerful language understanding and generation capabilities of large models, they have shown great potential in natural language interaction, knowledge reasoning, task planning, etc. AIAgent is taking over the big language model and has become a hot topic in the current AI circle. Au
