The AIxiv column is a column where this site publishes academic and technical content. In the past few years, the AIxiv column of this site has received more than 2,000 reports, covering top laboratories from major universities and companies around the world, effectively promoting academic exchanges and dissemination. If you have excellent work that you want to share, please feel free to contribute or contact us for reporting. Submission email: liyazhou@jiqizhixin.com; zhaoyunfeng@jiqizhixin.com
The authors of this article are from Shanghai Jiao Tong University, Tsinghua University, Cambridge University and Shanghai Artificial Intelligence Laboratory. The first author, Chen Zhe, is a PhD student at Shanghai Jiao Tong University, studying under Professor Wang Yu from the School of Artificial Intelligence of Shanghai Jiao Tong University. The corresponding authors are Professor Wang Yu (homepage: https://yuwangsjtu.github.io/) and Professor Zhang Chao from the Department of Electronic Engineering of Tsinghua University (homepage: https://mi.eng.cam.ac.uk/~cz277). 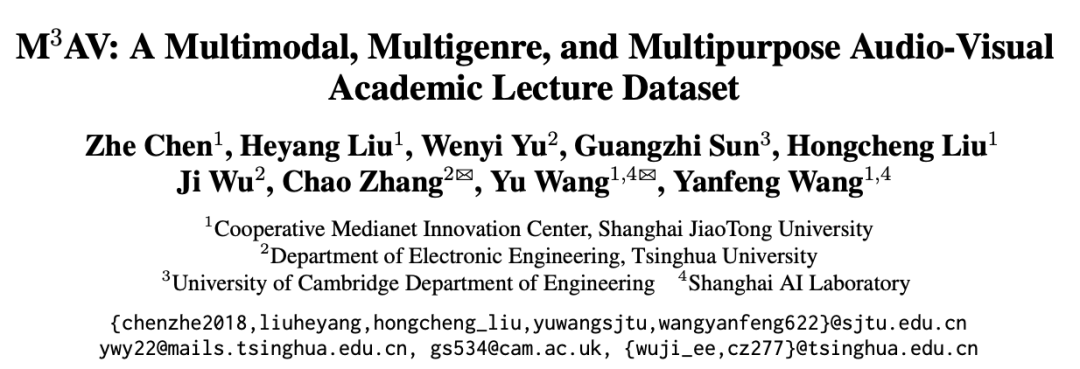
- Paper link: https://arxiv.org/abs/2403.14168
- Project homepage: https://jack-zc8.github.io/M3AV-dataset-page/
- Paper title: M3AV: A Multimodal, Multigenre, and Multipurpose Audio-Visual Academic Lecture Dataset
Open source academic lecture recording is a commonly popular way to share academic knowledge online Methods. These videos contain rich multimodal information, including the speaker's voice, facial expressions, and body movements, the text and images in the slides, and the corresponding paper text information. There are currently very few datasets that can simultaneously support multi-modal content recognition and understanding tasks, partly due to the lack of high-quality human annotation. 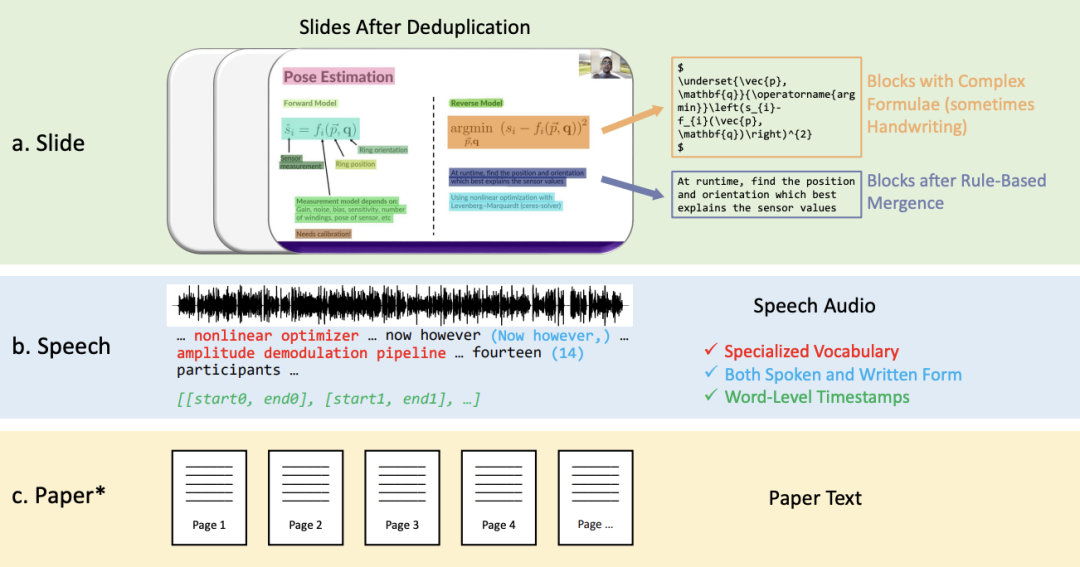
This work proposes a new multi-modal, multi-type, multi-purpose audio-visual academic speech dataset (M3AV), which contains nearly 367 hours of videos from five sources, covering computer science, mathematics, Medical and Biological Topics. With high-quality human annotations, especially high-value named entities, the dataset can be used for a variety of audio-visual recognition and understanding tasks. Evaluations on contextual speech recognition, speech synthesis, and slide and script generation tasks show that the diversity of M3AV makes it a challenging dataset. This work has been accepted by the ACL 2024 main conference. M3AV dataset mainly consists of the following parts: 1. Slides with complex blocks, they will be arranged according to their spatial positions Relationships are merged. 2. Speech-transcribed text in spoken and written form, including special vocabulary and word-level timestamps. 3. The paper text corresponding to the video. As can be seen from the table below, the M3AV dataset contains the most manually annotated slides, speech and paper resources, so it not only supports multi-modal content recognition tasks, but also supports advanced academic knowledge Understand the task . 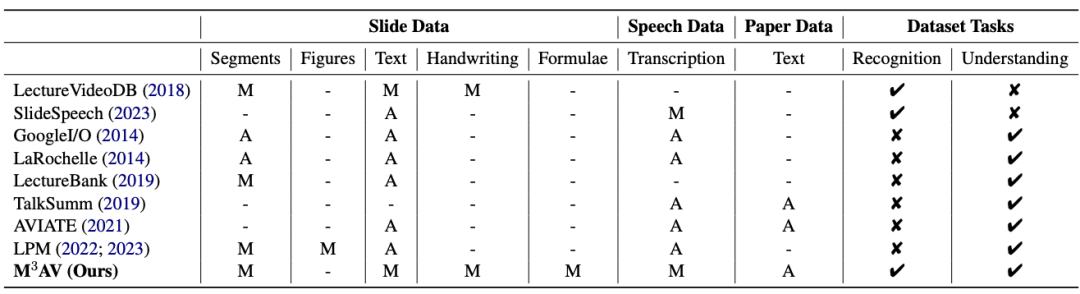
At the same time, the M3AV data set is richer in content than other academic data sets in all aspects, and it is also an accessible resource.
The M3AV data set is designed with three tasks in multi-modal perception and understanding, namely context-based speech recognition, spontaneous style speech synthesis, and slide and script generation. Task 1: Context-based speech recognition General end-to-end models have problems in rare word recognition. As can be seen from the AED and RNN-T models in the table below, the rare word error rate (BWER) has increased by more than twice compared to the total word error rate (WER). By leveraging OCR information for context-based speech recognition using TCPGen, the RNN-T model achieved a relative reduction of 37.8% and 34.2% in BWER on the development and test sets, respectively.
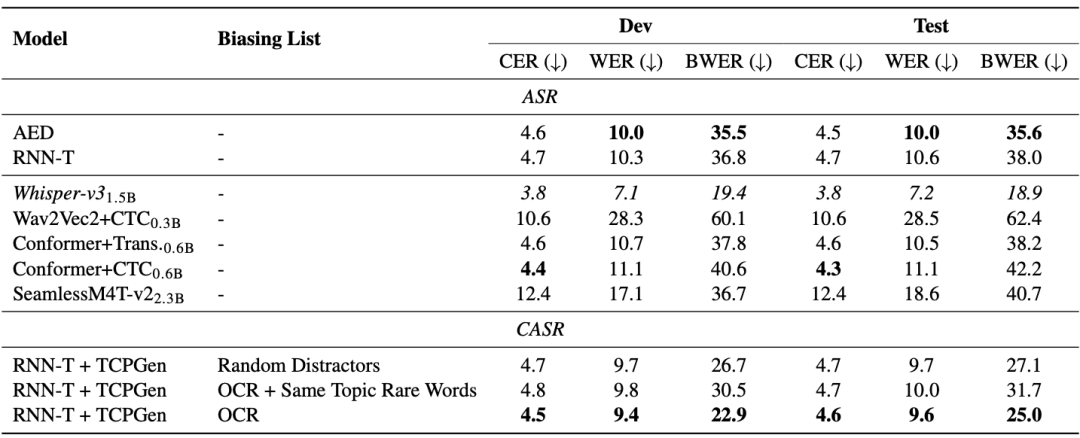
Task 2: Spontaneous style speech synthesis Spontaneous style speech synthesis systems urgently need speech data in real scenarios to produce speech that is closer to natural conversational patterns. The author of the paper introduced MQTTS as an experimental model and found that compared with various pre-trained models, MQTTS has the best evaluation indicators. This shows that real speech in the M3AV dataset can drive AI systems to simulate more natural speech. 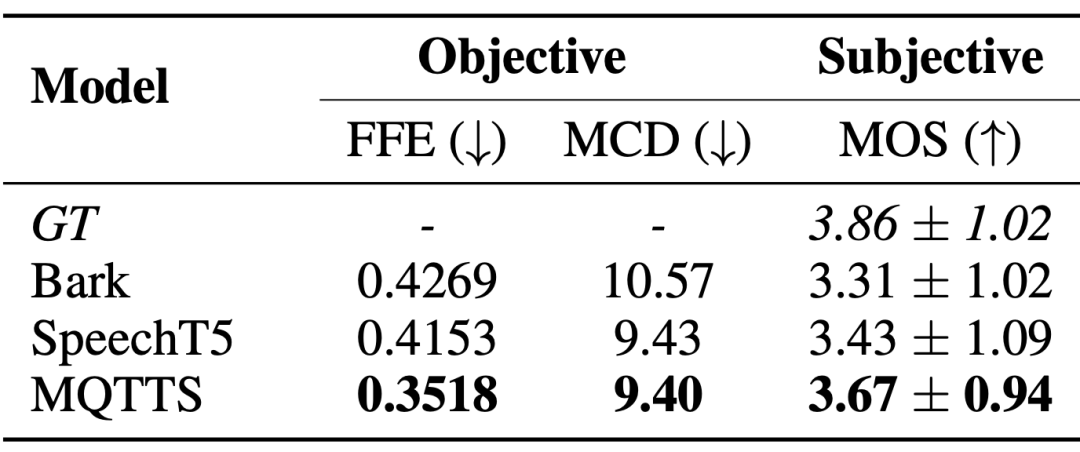
Task 3: Slide and Script Generation The Slide and Script Generation (SSG) task is designed to promote AI models to understand and reconstruct advanced academic knowledge, thereby helping researchers process quickly Update and iterate academic materials to effectively conduct academic research. As can be seen from the table below, the performance improvement of open source models (LLaMA-2, InstructBLIP) is limited when increasing from 7B to 13B, lagging behind closed source models (GPT-4 and GPT-4V). Therefore, in addition to increasing the model size, the author of the paper believes that high-quality multi-modal pre-training data is also needed. Notably, the advanced multimodal large model (GPT-4V) has outperformed cascaded models composed of multiple single-modal models. 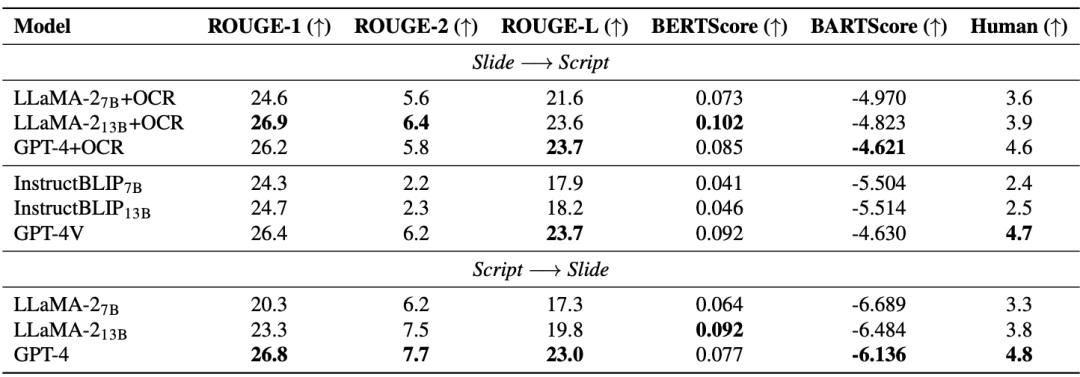
In addition, Retrieval Enhanced Generation (RAG) effectively improves model performance: The table below shows that the introduced paper text also improves the quality of the generated slides and scripts. 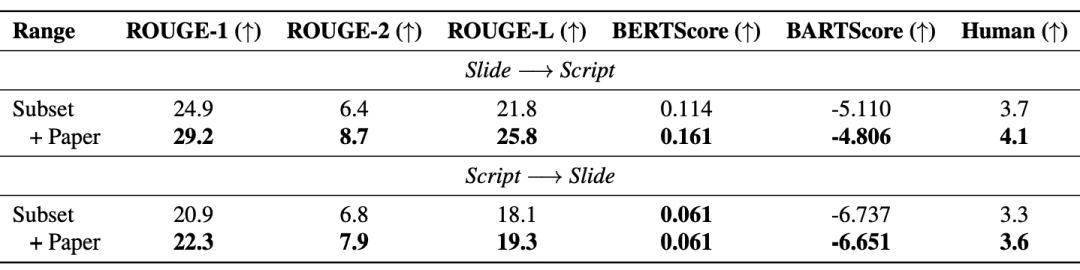
This work releases a multi-modal, multi-type, multi-purpose audiovisual dataset (M3AV) covering multiple academic fields. This dataset contains human-annotated speech transcriptions, slides, and additional extracted essay text, providing a basis for evaluating AI models’ ability to recognize multimodal content and understand academic knowledge. The authors of the paper describe the creation process in detail and conduct various analyzes on the data set. Additionally, they built benchmarks and conducted multiple experiments around the dataset. Ultimately, the authors of the paper found that existing models still have room for improvement in perceiving and understanding academic lecture videos. Partial annotation interface
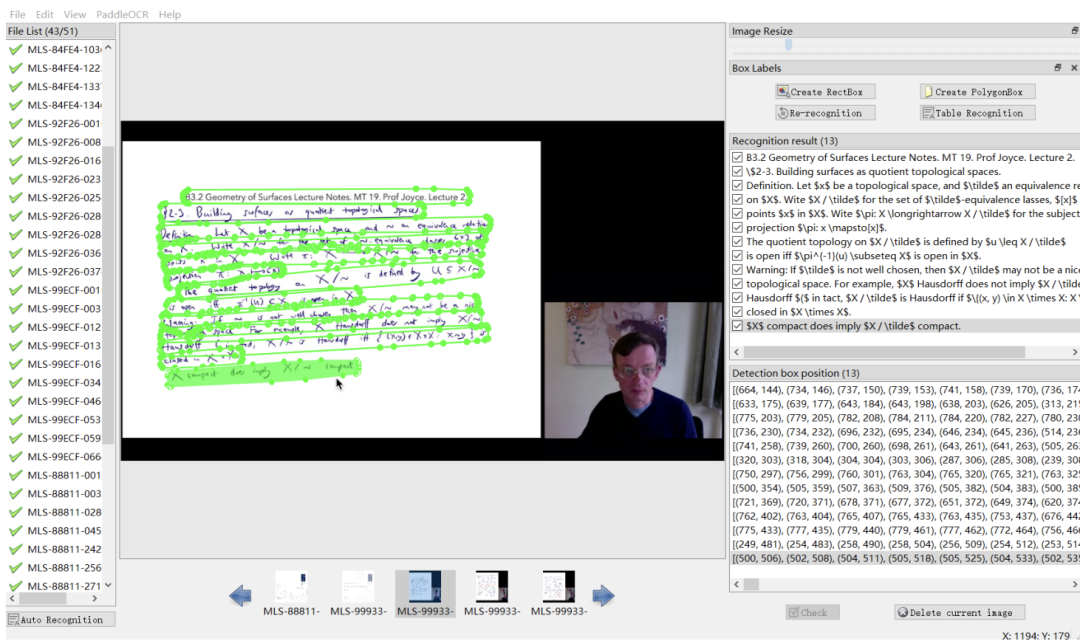
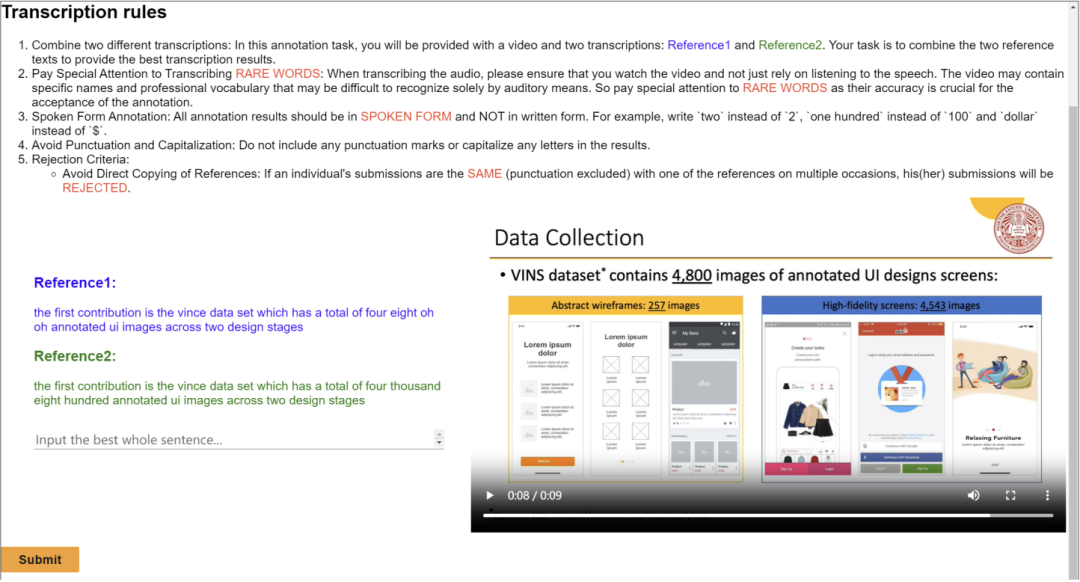
The above is the detailed content of ACL 2024 | Leading academic audiovisual research, Shanghai Jiao Tong University, Tsinghua University, Cambridge University, and Shanghai AILAB jointly released the academic audiovisual data set M3AV. For more information, please follow other related articles on the PHP Chinese website!