


DeepMind develops neural network variational Monte Carlo for quantum chemical calculations
Here, researchers from DeepMind and Imperial College London tackle this problem using a recently developed Fermionic Neural Network (FermiNet) wave function that does not rely on a basis set. FermiNet was found to produce highly precise, and in some cases state-of-the-art, ground state energies in a range of atoms and small molecules with a variety of qualitative positron binding properties.
The researchers calculated the binding energies of the challenging non-polar benzene molecule and found good agreement with experimental values and obtained annihilation rates that were more favorable than those obtained using explicitly correlated Gaussian wave functions. The results demonstrate the general advantages of methods based on neural network wave functions and apply them to systems other than the standard molecular Hamiltonian.
Relevant research was titled "Neural network variational Monte Carlo for positronic chemistry" and was published in "Nature Communications" on June 18.
Paper link: https://www.nature.com/articles/s41467-024-49290-1 Advances in experimental setups to capture large numbers of positrons have driven the development of more efficient computational tools to describe positron binding state, accelerating the innovation of antimatter technology. Quantum chemical calculations of the ground state properties of positron-molecule complexes are challenging. The main difficulty lies in using an appropriate basis set to represent the coalescence between electrons and positrons.
Here, researchers propose a new method for calculating the ground state properties of a molecule's positron-bound state, based on the neural network wave function assumption recently developed for QMC. Fermion neural networks (FermiNet) model multi-body wave functions without reference to a set of basis functions. This conveniently circumvents many of the difficulties described above in describing the positron wave function.
Researchers extended FermiNet to represent the positron component of the wave function equally well as the electron component. Flexible and accurate hybrid electron-positron wave function assumptions can be obtained with minimal changes to the neural network architecture. Positron binding energies and annihilation rates were calculated for a series of systems with different positron binding mechanisms, and the highest accuracy was obtained for the ground state energies of these systems.
Results on positronic hydrides, sodium and magnesium atoms, and small diatomic molecules show that the method can achieve state-of-the-art accuracy compared to previous studies. Furthermore, results for nonpolar dilithium and benzene molecules show that this precision is preserved when describing positron binding modes that are entirely controlled by strong electron-positron correlation effects.
The diagram below provides an intuitive understanding of the binding mechanism between non-polar molecules and positrons: Correlation-dominated binding is facilitated by centers of increased electron density away from the molecule's nuclei. In dilithium this is a covalent bond; in benzene this is an increase in electron density at the center of the molecule due to delocalization of π bonds in the ring.
Illustration: Ground state single particle density of positron lithium and benzene. (Source: paper) The positron binding energy of benzene obtained by this method is very close to the experimental value and the many-body theory of Hofierka et al. at Queen's University. And the obtained annihilation rates are comparable to high-precision ECG-SVM calculations of alkali metal atoms and small molecules. Furthermore, the 2γ annihilation rates of positron atoms and molecules accumulated using the FermiNet wave function are compared with the results obtained by various other calculation methods, as shown in the following table: Table: Compared with the annihilation rates obtained by various other calculation methods Ratio, 2γ annihilation rates of positron atoms and molecules accumulated using FermiNet wave functions. (Source: paper)
1. Compared with other methods, ECG-SVM calculates the annihilation rate by constructing features that best capture the wave function.
- In summary, the ECG-SVM method produces highly accurate results for a wide variety of molecules with various positron binding mechanisms without system-specific adjustments.
The above is the detailed content of DeepMind develops neural network variational Monte Carlo for quantum chemical calculations. For more information, please follow other related articles on the PHP Chinese website!

Hot AI Tools

Undresser.AI Undress
AI-powered app for creating realistic nude photos

AI Clothes Remover
Online AI tool for removing clothes from photos.

Undress AI Tool
Undress images for free

Clothoff.io
AI clothes remover

Video Face Swap
Swap faces in any video effortlessly with our completely free AI face swap tool!

Hot Article

Hot Tools

Notepad++7.3.1
Easy-to-use and free code editor

SublimeText3 Chinese version
Chinese version, very easy to use

Zend Studio 13.0.1
Powerful PHP integrated development environment

Dreamweaver CS6
Visual web development tools

SublimeText3 Mac version
God-level code editing software (SublimeText3)

Hot Topics










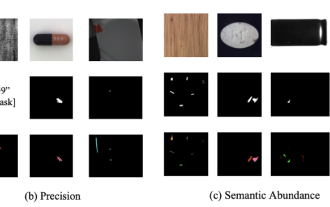
In modern manufacturing, accurate defect detection is not only the key to ensuring product quality, but also the core of improving production efficiency. However, existing defect detection datasets often lack the accuracy and semantic richness required for practical applications, resulting in models unable to identify specific defect categories or locations. In order to solve this problem, a top research team composed of Hong Kong University of Science and Technology Guangzhou and Simou Technology innovatively developed the "DefectSpectrum" data set, which provides detailed and semantically rich large-scale annotation of industrial defects. As shown in Table 1, compared with other industrial data sets, the "DefectSpectrum" data set provides the most defect annotations (5438 defect samples) and the most detailed defect classification (125 defect categories
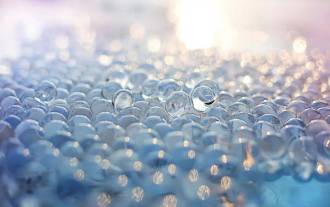
Editor |KX To this day, the structural detail and precision determined by crystallography, from simple metals to large membrane proteins, are unmatched by any other method. However, the biggest challenge, the so-called phase problem, remains retrieving phase information from experimentally determined amplitudes. Researchers at the University of Copenhagen in Denmark have developed a deep learning method called PhAI to solve crystal phase problems. A deep learning neural network trained using millions of artificial crystal structures and their corresponding synthetic diffraction data can generate accurate electron density maps. The study shows that this deep learning-based ab initio structural solution method can solve the phase problem at a resolution of only 2 Angstroms, which is equivalent to only 10% to 20% of the data available at atomic resolution, while traditional ab initio Calculation
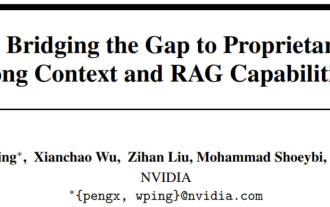
The open LLM community is an era when a hundred flowers bloom and compete. You can see Llama-3-70B-Instruct, QWen2-72B-Instruct, Nemotron-4-340B-Instruct, Mixtral-8x22BInstruct-v0.1 and many other excellent performers. Model. However, compared with proprietary large models represented by GPT-4-Turbo, open models still have significant gaps in many fields. In addition to general models, some open models that specialize in key areas have been developed, such as DeepSeek-Coder-V2 for programming and mathematics, and InternVL for visual-language tasks.
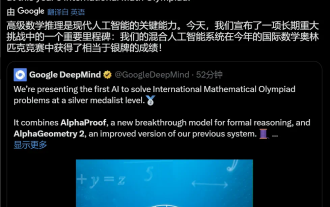
For AI, Mathematical Olympiad is no longer a problem. On Thursday, Google DeepMind's artificial intelligence completed a feat: using AI to solve the real question of this year's International Mathematical Olympiad IMO, and it was just one step away from winning the gold medal. The IMO competition that just ended last week had six questions involving algebra, combinatorics, geometry and number theory. The hybrid AI system proposed by Google got four questions right and scored 28 points, reaching the silver medal level. Earlier this month, UCLA tenured professor Terence Tao had just promoted the AI Mathematical Olympiad (AIMO Progress Award) with a million-dollar prize. Unexpectedly, the level of AI problem solving had improved to this level before July. Do the questions simultaneously on IMO. The most difficult thing to do correctly is IMO, which has the longest history, the largest scale, and the most negative
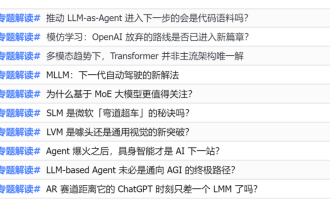
In 2023, almost every field of AI is evolving at an unprecedented speed. At the same time, AI is constantly pushing the technological boundaries of key tracks such as embodied intelligence and autonomous driving. Under the multi-modal trend, will the situation of Transformer as the mainstream architecture of AI large models be shaken? Why has exploring large models based on MoE (Mixed of Experts) architecture become a new trend in the industry? Can Large Vision Models (LVM) become a new breakthrough in general vision? ...From the 2023 PRO member newsletter of this site released in the past six months, we have selected 10 special interpretations that provide in-depth analysis of technological trends and industrial changes in the above fields to help you achieve your goals in the new year. be prepared. This interpretation comes from Week50 2023
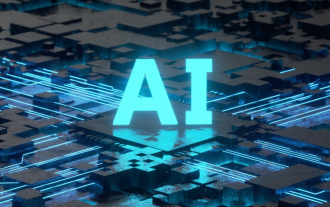
Editor |ScienceAI Question Answering (QA) data set plays a vital role in promoting natural language processing (NLP) research. High-quality QA data sets can not only be used to fine-tune models, but also effectively evaluate the capabilities of large language models (LLM), especially the ability to understand and reason about scientific knowledge. Although there are currently many scientific QA data sets covering medicine, chemistry, biology and other fields, these data sets still have some shortcomings. First, the data form is relatively simple, most of which are multiple-choice questions. They are easy to evaluate, but limit the model's answer selection range and cannot fully test the model's ability to answer scientific questions. In contrast, open-ended Q&A

Editor | KX Retrosynthesis is a critical task in drug discovery and organic synthesis, and AI is increasingly used to speed up the process. Existing AI methods have unsatisfactory performance and limited diversity. In practice, chemical reactions often cause local molecular changes, with considerable overlap between reactants and products. Inspired by this, Hou Tingjun's team at Zhejiang University proposed to redefine single-step retrosynthetic prediction as a molecular string editing task, iteratively refining the target molecular string to generate precursor compounds. And an editing-based retrosynthetic model EditRetro is proposed, which can achieve high-quality and diverse predictions. Extensive experiments show that the model achieves excellent performance on the standard benchmark data set USPTO-50 K, with a top-1 accuracy of 60.8%.
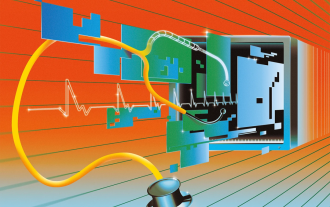
Editor | ScienceAI Based on limited clinical data, hundreds of medical algorithms have been approved. Scientists are debating who should test the tools and how best to do so. Devin Singh witnessed a pediatric patient in the emergency room suffer cardiac arrest while waiting for treatment for a long time, which prompted him to explore the application of AI to shorten wait times. Using triage data from SickKids emergency rooms, Singh and colleagues built a series of AI models that provide potential diagnoses and recommend tests. One study showed that these models can speed up doctor visits by 22.3%, speeding up the processing of results by nearly 3 hours per patient requiring a medical test. However, the success of artificial intelligence algorithms in research only verifies this
