Visualizing Big Data with Python: Best Practices and Tools
In the era of big data, effective visualization is essential for transforming complex datasets into actionable insights. Python, with its extensive libraries and tools, provides a robust framework for visualizing large datasets. This article explores the best practices and tools for visualizing big data using Python.
The Importance of Data Visualization
Data visualization plays a crucial role in:
- Making data comprehensible.
- Identifying trends, patterns, and outliers.
- Communicating results to stakeholders.
Best Practices for Visualizing Big Data
- Simplify the Data
- Aggregation: Summarize data using means, medians, or sums to reduce complexity.
- Sampling: Use a representative subset of the data when full data visualization is impractical.
- Filtering: Focus on the most relevant data points or time periods.
- Choose the Right Type of Visualization
- Line Charts: Ideal for time series data.
- Bar Charts: Suitable for comparing quantities.
- Scatter Plots: Useful for identifying correlations.
- Heatmaps: Effective for showing data density and distributions.
- Use Efficient Libraries and Tools
- Leverage libraries designed for performance and scalability.
- Optimize Performance
- Asynchronous Loading: Load data incrementally to avoid long waits.
- Data Caching: Cache data to speed up repeated queries.
- Parallel Processing: Utilize multiple processors to handle large datasets.
- Enhance Interactivity
- Interactive elements like tooltips, zooming, and panning help users explore data more effectively.
*Essential Python Tools for Big Data Visualization
*
- Matplotlib
Matplotlib is a versatile library that provides a foundation for other visualization libraries. It’s great for creating static, animated, and interactive visualizations.
import matplotlib.pyplot as plt plt.plot(data['date'], data['value']) plt.xlabel('Date') plt.ylabel('Value') plt.title('Time Series Data') plt.show()
- Seaborn
Built on top of Matplotlib, Seaborn offers a high-level interface for drawing attractive statistical graphics.
import seaborn as sns sns.set(style="darkgrid") sns.lineplot(x="date", y="value", data=data)
- Plotly
Plotly is known for its interactive plots, which can be embedded in web applications. It supports large datasets through WebGL.
import plotly.express as px fig = px.scatter(data, x='date', y='value', title='Interactive Scatter Plot') fig.show()
- Bokeh
Bokeh creates interactive plots and dashboards with high-performance interactivity over large datasets.
from bokeh.plotting import figure, show, output_file output_file("line.html") p = figure(title="Line Chart", x_axis_label='Date', y_axis_label='Value', x_axis_type='datetime') p.line(data['date'], data['value'], legend_label='Value', line_width=2) show(p)
- Altair
Altair is a declarative statistical visualization library that is user-friendly and integrates well with Jupyter notebooks.
import altair as alt chart = alt.Chart(data).mark_line().encode(x='date', y='value').interactive() chart.show()
- Dask
Dask can handle parallel computing, making it suitable for processing and visualizing large datasets efficiently.
import dask.dataframe as dd dask_df = dd.read_csv('large_dataset.csv')
Example: Visualizing a Large Dataset with Plotly and Dask
Here's an example that demonstrates how to visualize a large dataset using Plotly and Dask:
import dask.dataframe as dd import plotly.express as px # Load a large dataset with Dask dask_df = dd.read_csv('large_dataset.csv') # Convert to Pandas DataFrame for plotting df = dask_df.compute() # Create an interactive scatter plot with Plotly fig = px.scatter(df, x='date', y='value', title='Large Dataset Visualization') fig.show()
Conclusion
Visualizing big data with Python requires the right combination of tools and best practices to handle performance and clarity challenges. By leveraging libraries like Matplotlib, Seaborn, Plotly, Bokeh, and Altair, along with optimization techniques, you can create compelling and insightful visualizations that help uncover the hidden stories within your data. Remember, the key to effective data visualization lies in simplifying the data, choosing appropriate visualization types, and ensuring interactivity for deeper data exploration.
Please make sure to ask your questions in the comment below. Thank you for reading.
The above is the detailed content of Visualizing Big Data with Python: Best Practices and Tools. For more information, please follow other related articles on the PHP Chinese website!

Hot AI Tools

Undresser.AI Undress
AI-powered app for creating realistic nude photos

AI Clothes Remover
Online AI tool for removing clothes from photos.

Undress AI Tool
Undress images for free

Clothoff.io
AI clothes remover

Video Face Swap
Swap faces in any video effortlessly with our completely free AI face swap tool!

Hot Article

Hot Tools

Notepad++7.3.1
Easy-to-use and free code editor

SublimeText3 Chinese version
Chinese version, very easy to use

Zend Studio 13.0.1
Powerful PHP integrated development environment

Dreamweaver CS6
Visual web development tools

SublimeText3 Mac version
God-level code editing software (SublimeText3)

Hot Topics










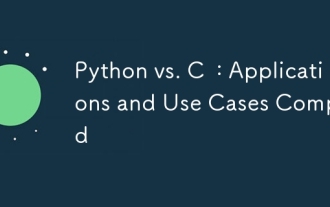
Python is suitable for data science, web development and automation tasks, while C is suitable for system programming, game development and embedded systems. Python is known for its simplicity and powerful ecosystem, while C is known for its high performance and underlying control capabilities.
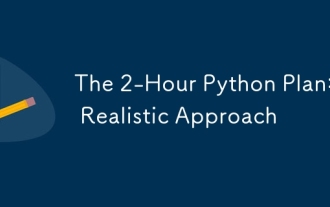
You can learn basic programming concepts and skills of Python within 2 hours. 1. Learn variables and data types, 2. Master control flow (conditional statements and loops), 3. Understand the definition and use of functions, 4. Quickly get started with Python programming through simple examples and code snippets.
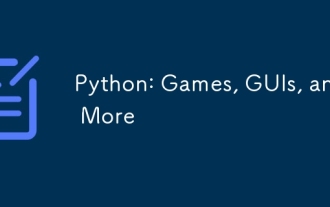
Python excels in gaming and GUI development. 1) Game development uses Pygame, providing drawing, audio and other functions, which are suitable for creating 2D games. 2) GUI development can choose Tkinter or PyQt. Tkinter is simple and easy to use, PyQt has rich functions and is suitable for professional development.
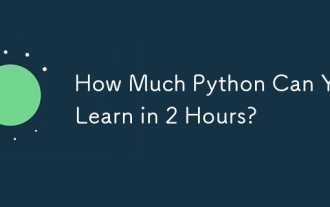
You can learn the basics of Python within two hours. 1. Learn variables and data types, 2. Master control structures such as if statements and loops, 3. Understand the definition and use of functions. These will help you start writing simple Python programs.
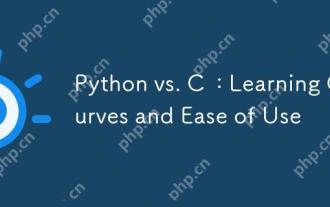
Python is easier to learn and use, while C is more powerful but complex. 1. Python syntax is concise and suitable for beginners. Dynamic typing and automatic memory management make it easy to use, but may cause runtime errors. 2.C provides low-level control and advanced features, suitable for high-performance applications, but has a high learning threshold and requires manual memory and type safety management.
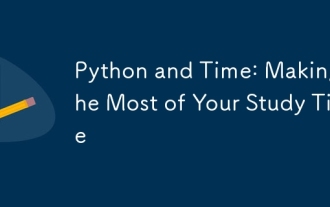
To maximize the efficiency of learning Python in a limited time, you can use Python's datetime, time, and schedule modules. 1. The datetime module is used to record and plan learning time. 2. The time module helps to set study and rest time. 3. The schedule module automatically arranges weekly learning tasks.
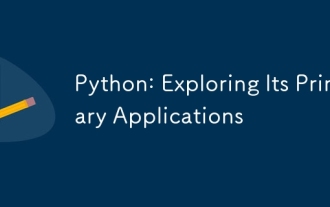
Python is widely used in the fields of web development, data science, machine learning, automation and scripting. 1) In web development, Django and Flask frameworks simplify the development process. 2) In the fields of data science and machine learning, NumPy, Pandas, Scikit-learn and TensorFlow libraries provide strong support. 3) In terms of automation and scripting, Python is suitable for tasks such as automated testing and system management.
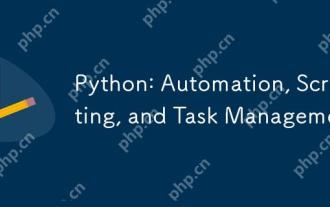
Python excels in automation, scripting, and task management. 1) Automation: File backup is realized through standard libraries such as os and shutil. 2) Script writing: Use the psutil library to monitor system resources. 3) Task management: Use the schedule library to schedule tasks. Python's ease of use and rich library support makes it the preferred tool in these areas.
