


ICML 2024 | Gradient checkpointing too slow? Without slowing down and saving video memory, LowMemoryBP greatly improves backpropagation video memory efficiency

The AIxiv column is a column where this site publishes academic and technical content. In the past few years, the AIxiv column of this site has received more than 2,000 reports, covering top laboratories from major universities and companies around the world, effectively promoting academic exchanges and dissemination. If you have excellent work that you want to share, please feel free to contribute or contact us for reporting. Submission email: liyazhou@jiqizhixin.com; zhaoyunfeng@jiqizhixin.com
The first author of this paper is Yang Yuchen, a second-year master's student in the School of Statistics and Data Science of Nankai University, and his advisor is Associate Professor Xu Jun in the School of Statistics and Data Science of Nankai University. The research focus of Professor Xu Jun’s team is computer vision, generative AI and efficient machine learning, and has published many papers in top conferences and journals, with more than 4,700 Google Scholar citations.
Since large-scale Transformer models have gradually become a unified architecture in various fields, fine-tuning has become an important means of applying pre-trained large models to downstream tasks. However, as the size of the model increases day by day, the video memory required for fine-tuning also gradually increases. How to efficiently reduce the fine-tuning video memory has become an important issue. Previously, when fine-tuning the Transformer model, in order to save graphics memory overhead, the usual approach was to use gradient checkpointing (also called activation recalculation) to reduce the time required in the backpropagation (BP) process at the expense of training speed. Activate video memory usage.
Recently, the paper "Reducing Fine-Tuning Memory Overhead by Approximate and Memory-Sharing Backpropagation" published at ICML 2024 by the team of Teacher Xu Jun from the School of Statistics and Data Science of Nankai University proposed that by changing the backpropagation (BP) process, in Without increasing the amount of calculation, the peak activation memory usage is significantly reduced.
Paper: Reducing Fine-Tuning Memory Overhead by Approximate and Memory-Sharing Backpropagation
Paper link: https://arxiv.org/abs/2406.16282
Project link: https:// /github.com/yyyyychen/LowMemoryBP
The article proposes two backpropagation improvement strategies, namely Approximate Backpropagation (Approx-BP) and Memory-Sharing Backpropagation (MS-BP). Approx-BP and MS-BP respectively represent two solutions to improve memory efficiency in backpropagation, which can be collectively referred to as LowMemoryBP. Whether in a theoretical or practical sense, the article provides groundbreaking guidance for more efficient backpropagation training.
In theoretical memory analysis, LowMemoryBP can significantly reduce the activation memory usage from activation functions and normalization layers. Taking ViT and LLaMA as examples, fine-tuning ViT can reduce activation memory by 39.47%, and fine-tuning LLaMA can reduce activation by 29.19%. video memory.
In actual experiments, LowMemoryBP can effectively reduce the peak memory usage of Transformer model fine-tuning including ViT, LLaMA, RoBERTa, BERT, and Swin by 20%~30%, and will not increase the training throughput and Loss of test accuracy.
Approx-BP
In traditional backpropagation training, the backpropagation of the gradient of the activation function strictly corresponds to its derivative function. For the GELU and SiLU functions commonly used in the Transformer model, this means that the input needs to be The feature tensor is completely stored in the active video memory. The author of this article proposed a set of backpropagation approximation theory, namely Approx-BP theory. Guided by this theory, the author uses a piecewise linear function to approximate the activation function, and replaces the backpropagation of the GELU/SiLU gradient with the derivative of the piecewise linear function (step function). This approach leads to two asymmetric memory-efficient activation functions: ReGELU2 and ReSiLU2. This type of activation function uses a 4-stage step function for reverse passback, so that the activation storage only needs to use a 2-bit data type.
MS-BP
BP Each layer of the network usually stores the input tensor into the activation memory for backpropagation calculation. The author points out that if the backpropagation of a certain layer can be rewritten into an output-dependent form, then this layer and the subsequent layer can share the same activation tensor, thus reducing the redundancy of activation storage.
The article points out that LayerNorm and RMSNorm, which are commonly used in Transformer models, can well meet the requirements of the MS-BP strategy after merging affine parameters into the linear layer of the latter layer. The redesigned MS-LayerNorm and MS-RMSNorm no longer generate independent active graphics memory.
Experimental results
The author conducted fine-tuning experiments on several representative models in the fields of computer vision and natural language processing. Among them, in the fine-tuning experiments of ViT, LLaMA and RoBERTa, the method proposed in the article reduced the peak memory usage by 27%, 29% and 21% respectively, without causing any loss in training effect and training speed. Note that the comparison Mesa (an 8-bit Activation Compressed Training method) reduces the training speed by about 20%, while the LowMemoryBP method proposed in the article completely maintains the training speed.
Conclusion and significance
The two BP improvement strategies proposed in the article, Approx-BP and MS-BP, both achieve the activation of video memory while maintaining the training effect and training speed. significant savings. This means that optimizing based on the BP principle is a very promising memory saving solution. In addition, the Approx-BP theory proposed in the article breaks through the optimization framework of traditional neural networks and provides theoretical feasibility for using unpaired derivatives. Its derived ReGELU2 and ReSiLU2 demonstrate the important practical value of this approach.
You are welcome to read the paper or code to understand the details of the algorithm. The relevant modules have been open sourced on the github repository of the LowMemoryBP project.
The above is the detailed content of ICML 2024 | Gradient checkpointing too slow? Without slowing down and saving video memory, LowMemoryBP greatly improves backpropagation video memory efficiency. For more information, please follow other related articles on the PHP Chinese website!

Hot AI Tools

Undresser.AI Undress
AI-powered app for creating realistic nude photos

AI Clothes Remover
Online AI tool for removing clothes from photos.

Undress AI Tool
Undress images for free

Clothoff.io
AI clothes remover

Video Face Swap
Swap faces in any video effortlessly with our completely free AI face swap tool!

Hot Article

Hot Tools

Notepad++7.3.1
Easy-to-use and free code editor

SublimeText3 Chinese version
Chinese version, very easy to use

Zend Studio 13.0.1
Powerful PHP integrated development environment

Dreamweaver CS6
Visual web development tools

SublimeText3 Mac version
God-level code editing software (SublimeText3)

Hot Topics










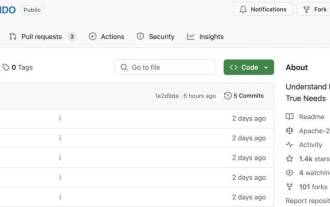
It is also a Tusheng video, but PaintsUndo has taken a different route. ControlNet author LvminZhang started to live again! This time I aim at the field of painting. The new project PaintsUndo has received 1.4kstar (still rising crazily) not long after it was launched. Project address: https://github.com/lllyasviel/Paints-UNDO Through this project, the user inputs a static image, and PaintsUndo can automatically help you generate a video of the entire painting process, from line draft to finished product. follow. During the drawing process, the line changes are amazing. The final video result is very similar to the original image: Let’s take a look at a complete drawing.

The AIxiv column is a column where this site publishes academic and technical content. In the past few years, the AIxiv column of this site has received more than 2,000 reports, covering top laboratories from major universities and companies around the world, effectively promoting academic exchanges and dissemination. If you have excellent work that you want to share, please feel free to contribute or contact us for reporting. Submission email: liyazhou@jiqizhixin.com; zhaoyunfeng@jiqizhixin.com The authors of this paper are all from the team of teacher Zhang Lingming at the University of Illinois at Urbana-Champaign (UIUC), including: Steven Code repair; Deng Yinlin, fourth-year doctoral student, researcher

The AIxiv column is a column where this site publishes academic and technical content. In the past few years, the AIxiv column of this site has received more than 2,000 reports, covering top laboratories from major universities and companies around the world, effectively promoting academic exchanges and dissemination. If you have excellent work that you want to share, please feel free to contribute or contact us for reporting. Submission email: liyazhou@jiqizhixin.com; zhaoyunfeng@jiqizhixin.com In the development process of artificial intelligence, the control and guidance of large language models (LLM) has always been one of the core challenges, aiming to ensure that these models are both powerful and safe serve human society. Early efforts focused on reinforcement learning methods through human feedback (RL
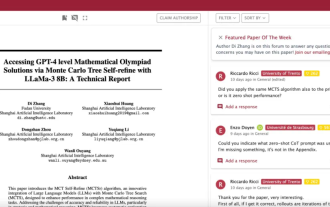
cheers! What is it like when a paper discussion is down to words? Recently, students at Stanford University created alphaXiv, an open discussion forum for arXiv papers that allows questions and comments to be posted directly on any arXiv paper. Website link: https://alphaxiv.org/ In fact, there is no need to visit this website specifically. Just change arXiv in any URL to alphaXiv to directly open the corresponding paper on the alphaXiv forum: you can accurately locate the paragraphs in the paper, Sentence: In the discussion area on the right, users can post questions to ask the author about the ideas and details of the paper. For example, they can also comment on the content of the paper, such as: "Given to
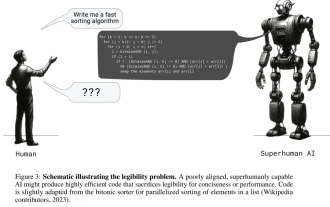
If the answer given by the AI model is incomprehensible at all, would you dare to use it? As machine learning systems are used in more important areas, it becomes increasingly important to demonstrate why we can trust their output, and when not to trust them. One possible way to gain trust in the output of a complex system is to require the system to produce an interpretation of its output that is readable to a human or another trusted system, that is, fully understandable to the point that any possible errors can be found. For example, to build trust in the judicial system, we require courts to provide clear and readable written opinions that explain and support their decisions. For large language models, we can also adopt a similar approach. However, when taking this approach, ensure that the language model generates

Recently, the Riemann Hypothesis, known as one of the seven major problems of the millennium, has achieved a new breakthrough. The Riemann Hypothesis is a very important unsolved problem in mathematics, related to the precise properties of the distribution of prime numbers (primes are those numbers that are only divisible by 1 and themselves, and they play a fundamental role in number theory). In today's mathematical literature, there are more than a thousand mathematical propositions based on the establishment of the Riemann Hypothesis (or its generalized form). In other words, once the Riemann Hypothesis and its generalized form are proven, these more than a thousand propositions will be established as theorems, which will have a profound impact on the field of mathematics; and if the Riemann Hypothesis is proven wrong, then among these propositions part of it will also lose its effectiveness. New breakthrough comes from MIT mathematics professor Larry Guth and Oxford University

The AIxiv column is a column where this site publishes academic and technical content. In the past few years, the AIxiv column of this site has received more than 2,000 reports, covering top laboratories from major universities and companies around the world, effectively promoting academic exchanges and dissemination. If you have excellent work that you want to share, please feel free to contribute or contact us for reporting. Submission email: liyazhou@jiqizhixin.com; zhaoyunfeng@jiqizhixin.com. Introduction In recent years, the application of multimodal large language models (MLLM) in various fields has achieved remarkable success. However, as the basic model for many downstream tasks, current MLLM consists of the well-known Transformer network, which
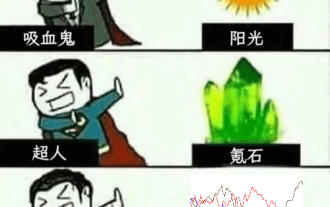
Can language models really be used for time series prediction? According to Betteridge's Law of Headlines (any news headline ending with a question mark can be answered with "no"), the answer should be no. The fact seems to be true: such a powerful LLM cannot handle time series data well. Time series, that is, time series, as the name suggests, refers to a set of data point sequences arranged in the order of time. Time series analysis is critical in many areas, including disease spread prediction, retail analytics, healthcare, and finance. In the field of time series analysis, many researchers have recently been studying how to use large language models (LLM) to classify, predict, and detect anomalies in time series. These papers assume that language models that are good at handling sequential dependencies in text can also generalize to time series.
