

In-depth interpretation of Privasea, can facial data cast NFT still be played like this?
Author: Shishijun
1. Introduction
2. From Web2 to Web3-human-machine confrontation never stops
Let’s take the ticket grabbing scenario as an example. By creating multiple virtual accounts to grab tickets, cheaters can greatly increase the probability of successful ticket grabbing, and some even directly deploy automated programs on Next to the service provider's computer room, ticket purchasing can be achieved with almost zero delay.
Ordinary users have almost no chance of winning against these high-tech users.
Service providers have also made some efforts in this regard. On the client side, in the Web2 scenario, real-name authentication, behavior verification codes and other methods are introduced to distinguish humans and machines. On the server side, feature filtering and interception are carried out through WAF policies and other means. .
Can this problem be solved?
Obviously not, because the benefits from cheating are huge.
At the same time, the confrontation between man and machine is continuous, and both cheaters and testers are constantly upgrading their arsenals.
Take cheaters as an example. Taking advantage of the rapid development of AI in recent years, the client's behavior verification code has almost been dimensionally reduced by various visual models. AI even has faster and more accurate recognition capabilities than humans. . This forces the verifiers to passively upgrade, gradually transitioning from early user behavioral feature detection (image verification code) to biometric feature detection (perceptual verification: such as client environment monitoring, device fingerprints, etc.). Some High-risk operations may require upgrading to biological feature detection (fingerprints, face recognition).
For Web3, human-machine detection is also a strong demand.
For some project airdrops, cheaters can create multiple fake accounts to launch witch attacks. At this time, we need to identify the real person.
Due to the financial attributes of Web3, for some high-risk operations, such as account login, currency withdrawal, transaction, transfer, etc., it is not only the real person who needs to verify the user, but also the account owner, so face recognition becomes indispensable. Choice of two.
How should we build a decentralized machine learning computing network? How to ensure that the privacy of user data is not leaked? How to maintain the operation of the network, etc.?
3. Privasea AI NetWork-Exploration of Privacy Computing + AI
Data Owner: Used to submit tasks and data securely through Privasea API.
Privanetix Node: It is the core of the entire network, equipped with advanced HESea library and integrated with blockchain-based incentive mechanism, which can perform safe and efficient calculations while protecting the privacy of the underlying data and ensuring calculation integrity and confidentiality.
Decryptor: Get the decrypted result through Privasea API and verify the result.
Result Receiver: The task results will be returned to the person designated by the data owner and task issuer.
3.2 The core workflow of Privasea AI NetWork
The following is the general workflow diagram of Privasea AI NetWork:
STEP 1: User registration: Data owner passed Provide the necessary authentication and authorization credentials to initiate the registration process on the Privacy AI Network. This step ensures that only authorized users can access the system and participate in network activities.
STEP 2: Task submission: Submit the calculation task and input data. The data is encrypted by the HEsea library. At the same time, the data owner also specifies the authorized decryptor and result receiver who can access the final result. By.
STEP 3: Task Allocation : Blockchain-based smart contracts deployed on the network allocate computing tasks to appropriate Privanetix nodes based on availability and capabilities. This dynamic allocation process ensures efficient resource allocation and distribution of computing tasks.
STEP 4: Encrypted calculation: The designated Privanetix node receives the encrypted data and uses the HESea library to perform calculations. These calculations can be performed without decrypting sensitive data, thus maintaining its confidentiality. To further verify the integrity of the calculations, Privanetix nodes generate zero-knowledge proofs for these steps.
STEP 5:密鑰切換:完成計算後,指定的 Privanetix 節點採用密鑰切換技術來確保最終結果是經過授權的,並且只有指定的解密器才能存取。
-
STEP 6:結果驗證:完成計算後,Privanetix 節點將加密結果和相應的零知識
:完成計算後,Privanetix 節點將加密結果和相應的零知識 - :完成計算後,Privanetix 節點將加密結果和相應的零知識證明。
STEP 7:激勵機制
- :追蹤 Privanetix 節點的貢獻,並分配獎勵
提取器。他們的首要任務是驗證計算的完整性,確保 Privanetix 節點按照資料所有者的意圖執行了計算。
STEP 9:結果交付:解密結果與資料擁有者預先確定的指定結果接收者共用。
4、FHE同態加密-新的密碼學聖杯?
5、FHE的限制
演算法最佳化方面,新的FHE方案如CKKS和優化的bootstrap方法顯著減少了噪音增長和計算開銷; 硬體方面,定制的提升多項式運算的效能。
6、總結
The above is the detailed content of In-depth interpretation of Privasea, can facial data cast NFT still be played like this?. For more information, please follow other related articles on the PHP Chinese website!

Hot AI Tools

Undresser.AI Undress
AI-powered app for creating realistic nude photos

AI Clothes Remover
Online AI tool for removing clothes from photos.

Undress AI Tool
Undress images for free

Clothoff.io
AI clothes remover

Video Face Swap
Swap faces in any video effortlessly with our completely free AI face swap tool!

Hot Article

Hot Tools

Notepad++7.3.1
Easy-to-use and free code editor

SublimeText3 Chinese version
Chinese version, very easy to use

Zend Studio 13.0.1
Powerful PHP integrated development environment

Dreamweaver CS6
Visual web development tools

SublimeText3 Mac version
God-level code editing software (SublimeText3)

Hot Topics










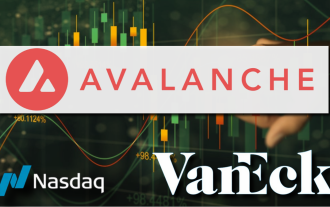
This new financial instrument would track the token's market price, with a third-party custodian holding the underlying AVAX
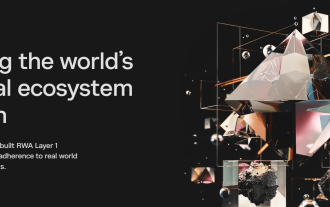
In a devastating blow to investors, the OM Mantra cryptocurrency has collapsed by approximately 90% in the past 24 hours, with the price plummeting to $0.58.
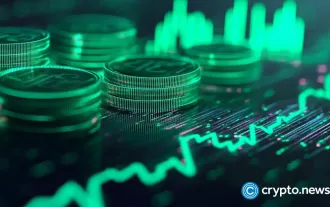
Zcash was one of the top gainers during the latest market rally, reaching a high of $35.69 as traders moved a record amount of tokens out of circulation.

A group of former Kraken executives acquired U.S.-listed company Janover, which secured $42 million in venture capital funding to begin building a Solana (SOL) treasury.
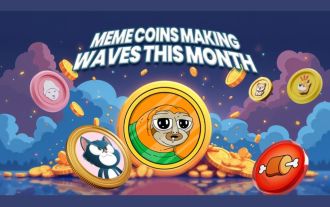
Have you noticed the meteoric rise of meme coins in the cryptocurrency world? What started as an online joke has quickly evolved into a lucrative investment opportunity
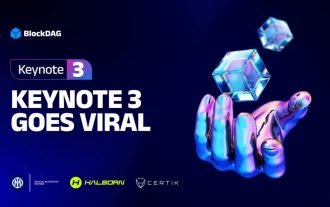
As fear drives selling in the crypto market, major coins like Cardano and Solana face tough times.
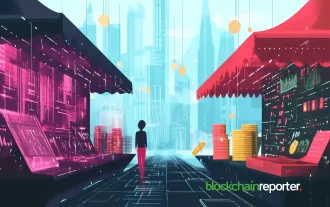
The crypto market has witnessed a rebound following the recent sheer downturn. As per the exclusive market data, the total crypto market capitalization has reached $2.71Ts
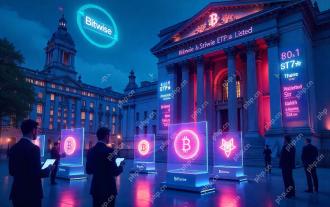
Bitwise, a leading digital asset manager, has announced the listing of four of its crypto Exchange-Traded Products (ETPs) on the London Stock Exchange (LSE).