
人类正在迎来人工智能领域的爆炸式更新,技术向未知拓展的每一步,几乎都引起惊人的关注度。
在人工智能边界扩张的过程中,重要赛道的技术路线创新与分歧并存。技术先锋者的判断和选择,影响着众多跟随者的脚步。
过去一年,本站独家率先将月之暗面、生数科技、爱诗科技、无问芯穹等优秀公司介绍给大家,为他们在互联网世界留下了第一份 “万字访谈底稿”。在技术路线尚未收敛的阶段,我们看到了到真正拥有信念、勇气以及系统化认知的 AI 创业者的引领力量。
因此,我们推出 “AI Pioneers” 的专栏,希望继续寻找和纪录 AGI 时代人工智能各细分赛道具有领袖气质的创业者,介绍 AI 赛道最出众、高潜的创业公司,分享他们在 AI 领域最前沿、鲜明的认知。
作者:姜菁玲
离开快手创业后,「李岩」悄悄拿到了快手联合创始人宿华、红点创投以及经纬创投的3200万美金种子轮融资。作为快手初始AI体系的核心人物,李岩曾搭建了快手内部第一个深度学习部门,后来还帮助快手搭建起了多模态内容理解Multi-Media Understanding 技术体系。他的其中一位投资人总结,在教授学者、移动互联网实干派,以及学术小天才,这三派AGI创业画像里,元石科技是唯一有能力整合「多模态、搜索、推荐」这三大核心技术栈的团队。不过,自2023年初李岩正式确认自己创业后,一年多来,他就几乎消失了。过去一年中,我们向李岩团队发出过多次采访邀请,希望跟他聊聊他的创业想法,都被「产品尚未准备好(公开)」婉拒。不久前,元石科技的产品「问小白」正式上线,冷启动内测开启,这也是李岩团队第一次创业进展披露。于是我们再次找到李岩,希望和他聊聊他的创业计划。在这次独家专访中,出乎意料的是,李岩的选择并不是一个纯模型公司,甚至也没有从多模态方面切入。在「问小白」这个产品中,用户开屏即在「feed」中,看到由AI针对用户兴趣个性化生成的AIGC内容,并可随时用「chat」功能与AI基于内容做更多的交互。可以理解为,这是一个基于自研LLM模型的生成式内容社区产品,与此前的内容社区产品相比,李岩的动作在于「生成式推荐」。这是一个前沿的技术研究领域,至今只有Meta与CMU有一些落地成果。他告诉我,相比于之前的推荐算法,生成式推荐算法将不再基于协同过滤的推荐系统,推荐将变得更加智能,从当下的「千人十面」变成真正意义上的「千人千面」。通过对用户更深层次需求的挖掘,推荐效率得到进一步提高,用户能够得到与自己更匹配的信息。而且,大量高质量语料对大模型的灌输,让生成式推荐算法拥有「价值观」。可以不再仅仅只是「讨好」用户,而是引导用户关注真正需要关注的优质信息。目前在国内,李岩团队是首家以LLM驱动的生成式推荐算法作为产品核心和发展方向的创业公司。元石的一位投资人认为,这种新的技术引擎在内容行业带来的成本与效率上的优化,与今日头条的成功路径基本一致。而在做生成式推荐算法产品这条路上,「我们看到,同时具备多模态、搜索、推荐三种背景的团队可能只有李岩」。李岩:我们希望通过技术创新,汇聚智能,帮助用户进入心流状态,对抗精神墒。(来自米哈里·契克森米哈赖的“心流”理论)李岩:我们觉得当下是一个信息爆炸的时代,接收信息的渠道很多, 但是真正能够获得自己关心信息的渠道缺失了。比如最近的WAIC,可能你会看到铺天盖地的报道但是每篇报导都是只言片语,但你并不能获取到你真正关心的信息,这时候你反而会陷入一种焦虑。我们理解这是一种「精神熵」,这个概念是一个心理学家米哈里·契克森米哈赖提出的,跟我们希望做的事情非常准确地匹配。我们想做的事,就是帮助大家提升看到信息之后的幸福感和收获感。這種狀態跟當下我們重度使用一些資訊產品過後的「更焦慮、更疲憊、更開心」會有所區別。 本站:什麼樣的訊息會讓人看到更幸福、更有收穫,而不是更焦慮、更疲憊? 李岩:這裡有一個「心流」的概念,它指的是,人只有看到自己真的想看到的資訊的時候,才會進入心流狀態,從而感受到幸福,而不是去看到很多跟自己無關,或是不感興趣的事。 這同樣是一個心理學研究結果,舉一個具體的例子,比如父母讓小孩寫作業,雖然最終是落實了,但小孩是被動的,他非常痛苦,他只有在做自己想做的事情的時候,他會覺得自己很快樂。所以我們希望幫助用戶進入心流的狀態,對抗精神熵。 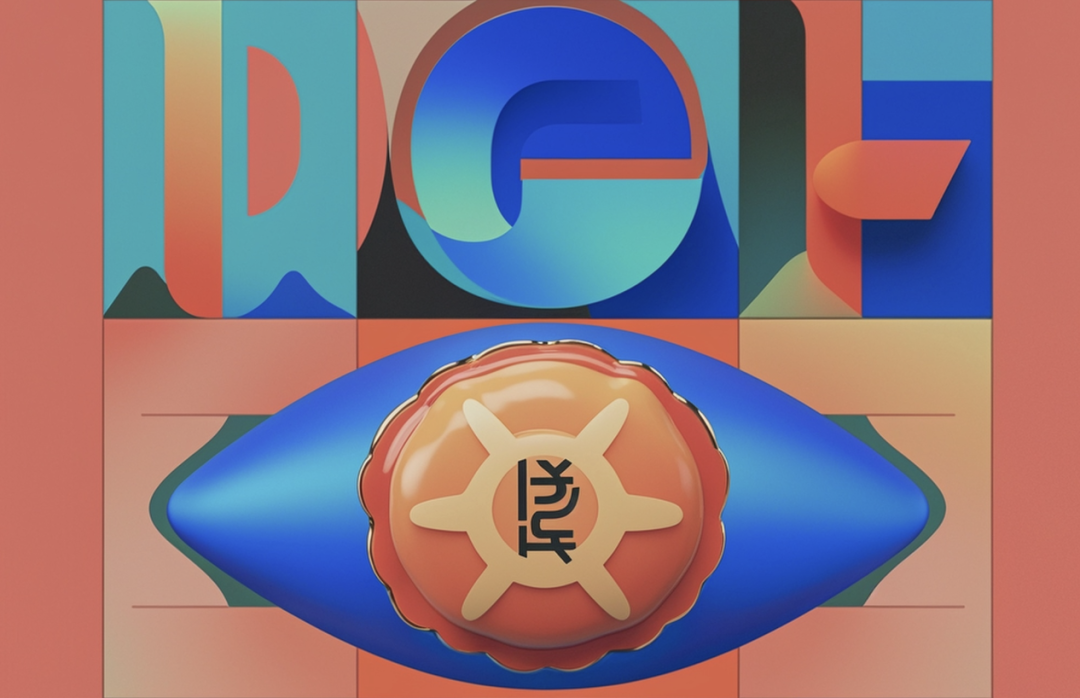
本站:現在其實大部分社交社群的底層推薦系統,本質上也是希望實現這種目標(給用戶推ta真正想看的)? 李岩:是有區別的。假如我們站在十年後,2034年看今天的推薦系統,包括產品和背後的技術,其實是非常落後的。現在產品所做的這些,其實並沒有達到一個很完美的狀態。 李岩:我可以做個類比,目前的訊息分發更像是原始人類階段的本能反應,在精神並沒有太豐富的階段,可能人的本能就是「我要吃飯」、「我要哭」、「我要笑」,這樣很直接的。 反應在推薦系統裡,可能就是,比如你喜歡帥哥,就會一直給你推帥哥——推薦系統並沒有過多的深入思考。而我們的產品希望做到的是,不是對使用者本能反應的討好,而是帶著更高的智慧,帶有關心和愛的推薦。 本站:這個聽起來是一個美學上更高的維度,有點想要「教育用戶」的意味。 李岩:準確地說並不是教育。很多事情在一個比較短的周期去看,是看不清楚的。但比如說我們拉長到整個人類發展的歷史上去看,我們會發現人類文明的每一次前進都會伴隨著批判、反思甚至推翻、重構,一些東西在當下是看起來不錯的,但在未來這可能就是有局限的。在網路世界也是這樣 ,我們希望把更文明的成分,人類所累積的先進思想帶到內容分發當中去。 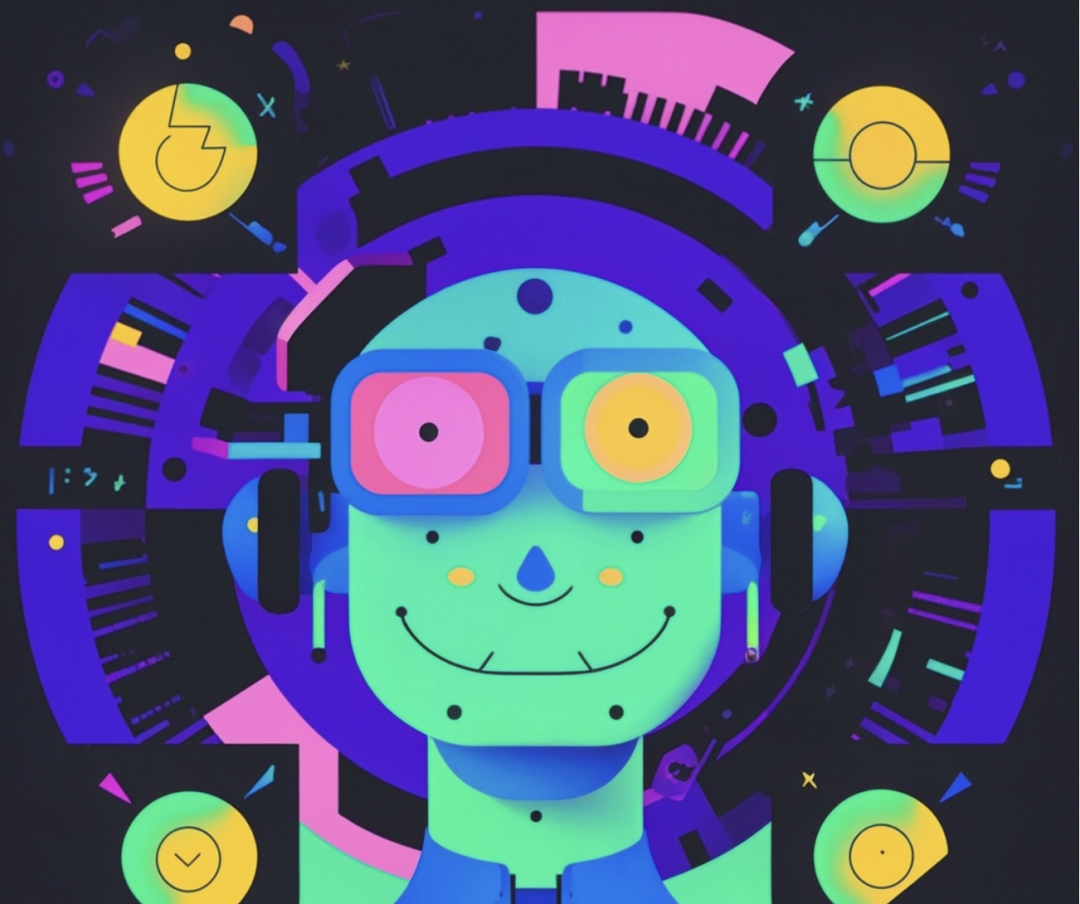
Technical implementation path: Choose higher quality data to train the model, so that the model has values
This site: I just said that I hope to create content that helps users better achieve flow. Products, why do you start by making a better LLM? Li Yan:We believe that LLM is a very important node leading to AGI. Large language models can better understand users and content, and know what users care about, like, and dislike. All the user's personal interests and hobbies can be tokenized, and large models can understand them very well. The previous recommendation system was unable to achieve this level of understanding. It could only label the user with many discrete labels, and use this to try to characterize and understand the user. Now, large models can not only better understand users' existing interests, but also enhance the mining of user interests and infer users' implicit interests and hobbies. With the large model, we can compress the highest quality corpus on the entire Internet and compress the human civilization carried in the text, thereby using these civilizations and further applying these capabilities to generative recommendations. , it will have its values and world view, and thus have a higher-dimensional recommended value system. The large model actually plays the role of a bridge, linking these most advanced cognitions with your information consumption, and then further improving your content consumption level. This site: Do these "advanced" contents refer to papers? Does it include both social sciences and natural sciences, or is it more focused on one? Li Yan: The large model will read all the advanced civilization and information accumulated by humans on the entire Internet, and it can be advanced in all aspects. This site: How does the large model determine what "advanced civilization" is? Li Yan: In fact, we humans have already made judgments on this matter, rather than big models. For example, our authoritative papers and journals, and books written by well-known scholars, these are not big model definitions , but high-quality information established by humans themselves over a long period of time. This site: Well, what exactly is this part of high-quality data? source? Li Yan: We value the construction of data-driven model capabilities. In our model, we use algorithms to increase the amount of available high-quality data by more than an order of magnitude. In addition, in terms of data selection, we use more classic books, theories, and papers to train our large models, so that our models have the ability to understand users more deeply. More specifically, , when it comes to content recommendation, we will not blindly let users stay in the short-term pleasure. Instead, there is the long-term happiness of accumulating high-quality information. This site: I just mentioned that the generative recommendation algorithm can improve the understanding of users. Is there any quantitative standard to compare the understanding of users by different recommendation algorithms? Li Yan:Since different companies pursue different goals, the optimization goals are also different. Generally speaking, it may be duration, click-through rate, and retention. Since our technical principles and business direction are new areas, we currently have a very complex internal data system to evaluate this matter. This site: On LLM, what are the current technical advantages of Yuanshi? Li Yan:Starting from the first day of the company’s establishment, that is, around April 2023, the first version of the large model was based on the MoE architecture. The overall technical route selection in the market is very forward-looking. From April 2023 to now for more than a year, our model has iterated four versions. On many public test sets, our results are better than many other models. In addition, our high-quality corpus makes the quality of answers very high, and the model has the ability to think deeply. Third, the speed of our large model is also very competitive, with extremely low latency. We have made extreme optimizations in model training and inference, which has greatly reduced the cost of training large models. We are now free and do not need to pay for use during peak periods.This site: Why do you think MoE is the superior route? Li Yan: We believe that to make our own products, we need the ability to integrate the underlying links of the model. In the era of large models, the model effect is better, which often means that the number of parameters is larger. . But as a to c product, if the cost of model inference is high, it will not work commercially. Therefore, we need both a large number of parameters and low inference cost. As a prerequisite for commercial feasibility, in the end we can only choose MoE. We thought about this problem clearly from the first day, and the first line of code we wrote was MoE. This site: Because Yuanshi is positioned as an application company, have you considered using some open source models during the research and development process, which may be more economical? Li Yan: Our goal is not to be a model-level company, but the reason why we still choose to develop large models ourselves is that we think other people’s models do not serve our goals. Yes, we are a product company driven by our own large model. We have not made any attempts at the business model at the model level. This is related to my personal knowledge. Some people think that large models are water and electricity, which means that once I make a good large model, you don't need to do it, and you can all use my abilities. But we believe that the greater significance of the large model lies in its ability to serve users to the extreme and its scenario-based capabilities. It serves users better in a fixed scenario and provides an experience that was completely unavailable before. Also, it turns out that the ability to fine-tune changes is limited. Because we are quite innovative in doing this, we need to make major changes in the underlying model architecture. We will also use our self-developed models internally to compare with open source models. Facts have proved that the results of our internal self-developed models are far better than those of open source models. Because this model is completely built for my scenario, a lot of work has been done from the construction of training data to the design of the algorithm. This site: You are also one of the early explorers of multi-modality in China. Do you have a timetable for multi-modality? Li Yan: At present, the text large model is still the core of the core. It is the basis of intelligence. 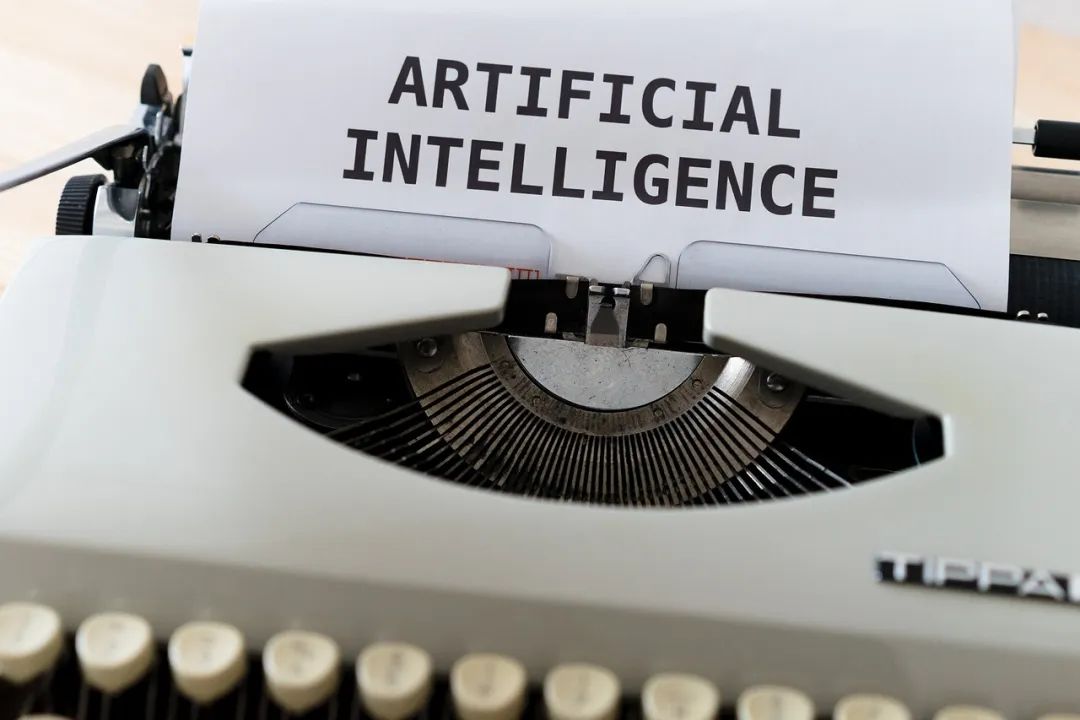
产品价值:能够更关注用户的个性化需求
本站:元石科技产品形态其实跟市面上几乎所有大模型C端产品都不一样,为什么会想要定义这样一款产品?李岩:我们不是一个针对特定人群的产品,我们面向广泛的人群,我们也不是一个垂直内容社区。我们认为随AI生成能力和分发能力的提升,AI时代未来的内容垂类的边界甚至会越来越模糊。在产品层面,目前我们产品有两个功能,一个是Feed一个是Chat。我们叫「问小白」,一个方面就是用户生活中有什么问题,都可以去问ta。另一方面是由小白「问」,基于用户问AI的问题,小白也会主动关心用户,主动给用户推送。名字叫小白,是希望用户有安全感,亲切感,抛弃掉冷冰冰的AI或者暴力的AI,跟用户是可以近距离接触的。本站:所以可以把它理解成一个带有AI功能的内容产品吗?李岩:可以,在此之外它还是一个实时在线的了解你喜好的朋友,那作为用户你有事你可以安排它去做,没事它可以观察你,看能帮到你什么主动做点事情。本站:Feed流的内容全部是AIGC的吗?怎么保证这部分内容的质量?李岩:用大模型生产内容的话,它首先是需要知道用户喜欢什么样的内容,再针对这些议题去生成,高质量地组织文章内容。这两个层面一方面是理解能力,一方面的生成能力,现在来看的话,这两方面的能力大模型都还有很大的提升空间。也是之所以创业的原因,因为我们认为自己有能力去将这个事情实现极大的提升。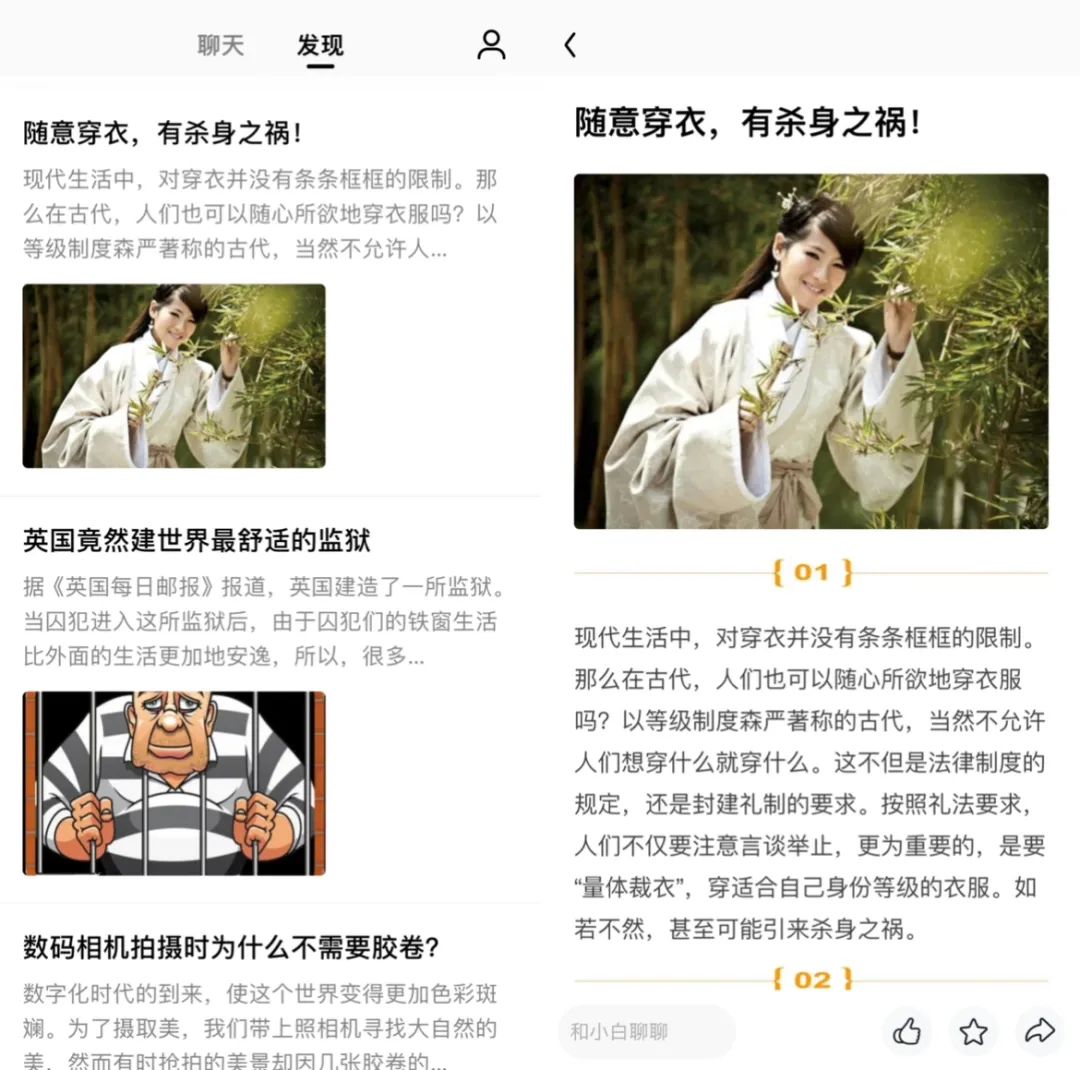
이 사이트: 제품이 Zhihu, Xiaohongshu, Toutiao의 AI 버전과 약간 비슷해 보입니다. 이들과 비교하면 차이점과 장점이 무엇인가요? Li Yan: 우선, 우리는 사용자의 개인화된 요구에 더 많은 관심을 기울입니다. 이전 세대에서 방금 말씀하신 모든 제품의 경우 추천 시스템의 가장 기본적인 원칙은 협업 필터링입니다. 즉, 한 사용자가 A와 B를 좋아하고 다른 사용자가 A와 C를 좋아하면 B와 C도 마찬가지입니다. 또한 비슷합니다. 그러면 각각 B와 C를 추천해 드리겠습니다. 이 협업 필터링 방법에는 매우 명백한 문제가 있습니다. 즉, 항상 일부 척추 카테고리를 추천한다는 것입니다. 왜요? 왜냐하면 당신이 어떤 주제를 좋아한다면 당신도 그 주제를 좋아하는 다른 사람들처럼 아름다운 여자와 잘생긴 남자를 더 좋아할 확률이 높고, 그들 모두는 오락을 좋아하는 경향이 있기 때문입니다. 그래서 시스템은 결국 당신이 실제로 오락을 좋아하고 잘생긴 남자와 아름다운 여자를 좋아한다고 결정할 것입니다. 이 방법에는 장점이 있으며 사용자 시간을 빠르게 단축하여 지속적인 성장을 이룰 수 있습니다. 하지만 문제는 사용자의 개인적인 관심과 틈새 관심을 묻어 사용자를 자세히 이해하기 어렵다는 것입니다. 그리고 우리는 대형 모델을 기반으로 이 작업을 수행합니다. 우선, 저희는 잘생긴 남자, 미인, 예능 콘텐츠를 추천하기보다는 귀하의 개인화된 관심사를 배려하기를 바랍니다. 이 경우, 이 추천 시스템은 진정한 개인화 추천 시스템이 아닙니다. 그래서 충분히 똑똑한 추천 시스템은 이론적으로 사용자의 모든 관심을 돌볼 수 있어야 합니다. 그것이 귀하의 일반적인 관심사이든 틈새 개인의 관심사이든 상관 없습니다. 지금까지 수행된 작업이 충분하지 않습니다. 이 사이트: 현재 흔히 볼 수 있는 챗봇이나 감성동반 상품 형태가 아닌, 창업할 때 왜 이 방향을 선택하셨나요? Li Yan:저희는 추천과 LLM의 통합이 새로운 유형의 상호 작용, 즉 "활성" 상호 작용이 필요하지 않은 원활한 경험을 정의할 수 있는 기회를 제공한다고 믿습니다. 현재 순수한 채팅 유형 상호 작용에는 여전히 사용자에 대한 특정 사용 임계값이 있으며 사용자는 적극적으로 질문을 시작해야 합니다. 어느 정도는 더 넓은 범위의 사용자가 침투하고 사용하는 것을 제한합니다. 오늘날 우리가 사용하는 모든 추천 제품과 마찬가지로 사용자가 많이 사용하지만 여전히 사용자가 해당 제품을 반복적으로 제거하는 것을 볼 수 있습니다. 척을 반복한다는 것은 그것 없이는 살 수 없다는 것을 의미하지만, 계속 미루는 것은 100% 만족하지 않는다는 것을 의미한다. 이는 추천 상품이 실제로는 여전히 좋은 기회를 갖고 있다는 생각을 하게 만드는 지점이다. 이를 바탕으로 저와 팀은 검색, AI 연구 및 대규모 제품 구현에 대한 깊은 경험을 가지고 있으며 이를 수행하는 데 매우 적합하다고 믿습니다. 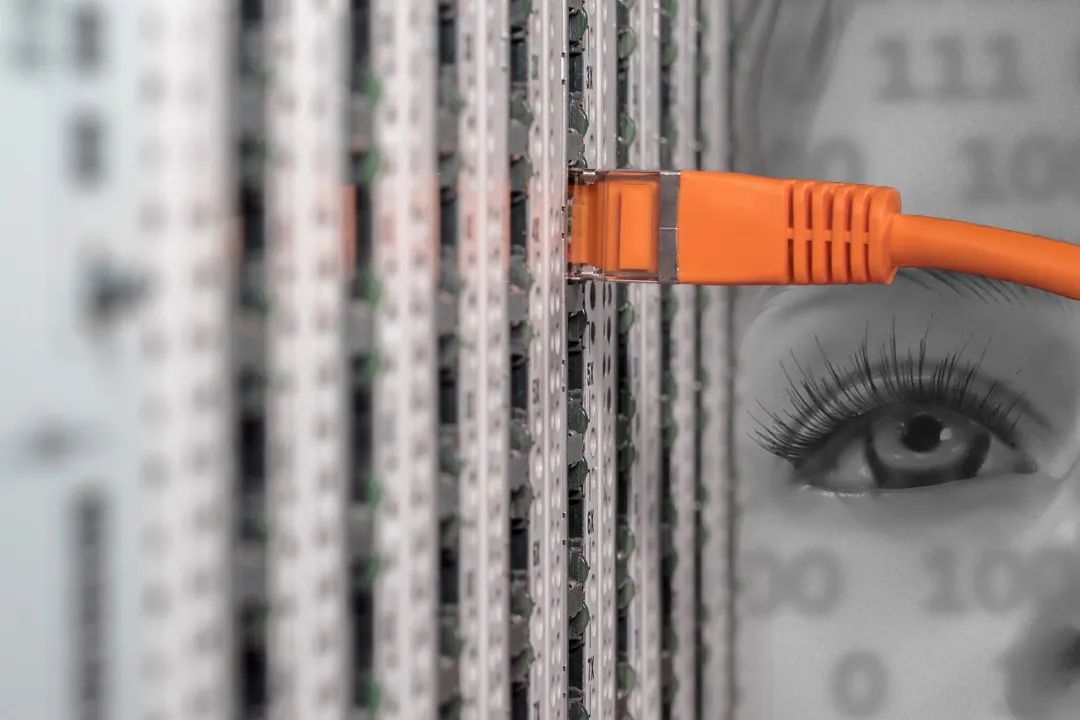
이 사이트: 그러나 현재 콘텐츠 기반 제품은 일반적으로 불분명한 상용화 경로의 딜레마에 직면하고 있으며 이에 대해 어떻게 생각하십니까? Li Yan: 우리는 아직 사용자의 가치를 더욱 완벽하게 보여주는 단계에 있습니다. 큰 사용자 가치를 바탕으로 상업적 가치를 이야기하는 것은 의미가 있습니다. Kuaishou와 같은 많은 제품이 대용량 콘텐츠 제품의 강력한 수익화 기능을 보여주는 매우 성공적인 사례를 제공했습니다. 이 사이트: 제품으로 돌아가서, 응답 능력이 더 뛰어난 제품의 가치는 무엇입니까? 리얀: 두 개 있는 것 같아요. 첫 번째는 답변이 좋을수록 사용자 충성도가 높아진다는 것입니다. 이 경우 더 많은 사용자 신호를 알 수 있고 사용자를 더 잘 이해할 수 있습니다. 궁극적으로 시스템은 이를 사용하여 사용자가 좋아하고 실제로 필요한 콘텐츠를 만들 수 있습니다. 긍정적인 경험과 데이터 사이클을 지속적으로 형성합니다. 이 사이트: 낙관적으로 생각하면, 생성 추천 알고리즘의 점진적인 성숙이 콘텐츠 산업에 어떤 영향을 미칠 수 있을까요? 당신의 상상 속에서 성숙한 "Ask Novice"는 어떤 모습일까요? Li Yan: 생성 추천은 콘텐츠 트랙에 새로운 활력을 불어넣어 정교한 개선이 아닌 이 분야에 큰 변화를 가져올 수 있게 해줍니다. 현재 대형 모델과 기타 관련 기술은 비약적으로 발전하고 있지만 인간-AI 통신에는 분명한 병목 현상이 있습니다. 두 가지 측면 모두에서 더 나은 성능을 발휘할 수 있습니다. 샤오바이에게 물어보세요, 샤오바이는 AI 기술의 포용성을 크게 촉진하고 AI가 필요한 일반 사용자가 AI의 힘을 더 많이 느낄 수 있기를 바랍니다. The above is the detailed content of Exclusive conversation with Li Yan: Funded by Suhua, Jingwei, and Redpoint, the first 'generative recommendation” startup | AI Pioneers. For more information, please follow other related articles on the PHP Chinese website!