


The performance is 11 times stronger. Georgia Tech and Tsinghua teams used AI to assist in discovering new energy storage materials, published in Nature sub-journal
Electrostatic capacitors are key energy storage components in advanced power systems in the fields of defense, aviation, energy and transportation. Energy density is the figure of merit of an electrostatic capacitor and is primarily determined by the choice of dielectric material.
Most industrial-grade polymer dielectric materials are flexible polyolefins or rigid aromatics that have either high energy density or high thermal stability, but not both at the same time.
Here, a research team from the Georgia Institute of Technology, the University of Connecticut, and Tsinghua University used artificial intelligence (AI), polymer chemistry, and molecular engineering to discover polynorbornene and poly A range of dielectrics in the imide family.
Many discovered dielectrics exhibit high thermal stability and high energy density over a wide temperature range. One of the dielectrics has an energy density of 8.3 J/cc at 200 °C, 11 times higher than any commercially available polymer dielectric at this temperature.
The researchers are also evaluating ways to further enhance the polynorbornene and polyimide families so that these capacitors can perform well in demanding applications such as aerospace while being environmentally sustainable.
These findings expand the potential applications of electrostatic capacitors in the 85-200°C temperature range; also demonstrate the impact of artificial intelligence on chemical structure generation and property prediction, highlighting the potential for advancements in materials design beyond electrostatic capacitors.
The research was titled "AI-assisted discovery of high-temperature dielectrics for energy storage" and was published in "Nature Communications" on July 19, 2024.
Electrostatic capacitors play a vital role as energy storage devices in modern electrical systems. Compared with other energy storage devices such as batteries, fuel cells and supercapacitors, electrostatic capacitors provide excellent power density (107 W/kg) and are used in wind pitch control (maximum temperature around 125 °C), hybrid and It has advantages in various fields such as fully electric vehicles (approx. 150 °C), pulsed power systems (approx. 180 °C), aircraft and launchers (approx. 300 °C) and space exploration (approx. 480 °C).
However, it remains a challenge to significantly increase the energy density Ue of electrostatic capacitors at high temperatures, which is crucial to achieve significant savings in space and weight.
Currently, biaxially oriented polypropylene (BOPP) has been used as a dielectric material for more than thirty years. Although BOPP has low dielectric loss and large electronic band gap Eg, its dielectric constant and high temperature stability are poor.
BOPP alternatives with high thermal stability have been explored commercially, but these polymers often come at the expense of low Eg and low Ue. These materials are insufficient to meet the needs of modern and future technologies.
Challenges of Materials Discovery
The performance of polymers depends largely on their chemical composition. The number of variants that can be produced from a single polymer through chemical transformations is staggering.
Among all the chemical possibilities of polymers, there may be many high-performance dielectric materials yet to be discovered. Artificial intelligence (AI) trained and calibrated, capable of processing vast amounts of data beyond human imagination, can quickly help discover new materials.
Efficient material discovery involves selecting or generating a chemical subspace, estimating the properties of each material within it, and then selecting candidate materials for synthesis and testing based at least in part on the estimated properties.
The challenge is to (1) create a subspace broad enough to discover unknown new materials while (2) limiting hypothetical materials that are difficult to synthesize (false positives). Furthermore, property estimates must be (3) accurate and (4) efficient, with the latter becoming increasingly important as chemical subspaces expand.
However, solving all these problems at the same time is not easy.
New paradigm polyVERSE
Researchers from Georgia Institute of Technology, Tsinghua University and other institutions proposed the polyVERSE (polymers designed by Virtually-Executed Rule-Based Synthesis Experiments) paradigm, demonstrating its realization of these four properties in the context of high-temperature dielectric search success.
In an AI-driven approach, an expert system is used to generate polymers from commercially available monomers and a multi-task graph neural network is used to estimate properties. These property estimates can be used to select (screen) promising polymers from larger populations.
1. Innovative polynorbornene dielectric
Researchers discovered a previously unknown polynorbornene dielectric called PONB-2Me5Cl.
2. Excellent energy density
在 200°C 時,PONB-2Me5Cl 具有 8.3 J/cc 的出色能量密度,高於所有商業替代品,使其成為在此溫度下報道的最佳聚合物電介質之一。
3. 與其他聚合物比較
在 200°C 以下,PONB-2Me5Cl 的能量密度也高於所有商業聚合物,僅次於 PSBNP-co-PTNI0.02。
4. 合成考量
由於 PSBNP-co-PTNI0.02 是一種共聚物,其合成可能需要更多步驟。此外,測量協議的差異也可能影響比較結果。
PONB-2Me5Cl 是使用 polyVERSE 演算法 在電腦中發現的,隨後進行了合成和表徵。 高性能 PONB-2Me5Cl 聚合物的發現 和 polyVERSE 範式的開發 是這項工作的兩個成果。
此外,團隊提出了一系列未來需要考慮的聚合物設計最佳化。這些包括:
- PONB-2Me5Cl 的改良版本 (透過R 基團工程或加入奈米填料或塗層實現)
- 精選聚酰亞胺,這是研究人員根據現有的功能性
,這是研究人員根據現有的功能性
精選聚酰亞胺,這是研究人員根據現有的功能性高溫聚合物優先考慮的一類。
這些設計顯示出提高高溫 Ue 和減少損失的潛力,同時也允許使用綠色溶劑進行合成。 僅使用眾多聚合模板中的一種,這項研究就展示了
人工智慧🎜 在生產具有出色能量儲存能力的先進聚合物電介質方面的強大功能。 🎜🎜🎜論文連結:🎜 https://www.nature.com/articles/s41467-024-50413-x🎜The above is the detailed content of The performance is 11 times stronger. Georgia Tech and Tsinghua teams used AI to assist in discovering new energy storage materials, published in Nature sub-journal. For more information, please follow other related articles on the PHP Chinese website!

Hot AI Tools

Undresser.AI Undress
AI-powered app for creating realistic nude photos

AI Clothes Remover
Online AI tool for removing clothes from photos.

Undress AI Tool
Undress images for free

Clothoff.io
AI clothes remover

AI Hentai Generator
Generate AI Hentai for free.

Hot Article

Hot Tools

Notepad++7.3.1
Easy-to-use and free code editor

SublimeText3 Chinese version
Chinese version, very easy to use

Zend Studio 13.0.1
Powerful PHP integrated development environment

Dreamweaver CS6
Visual web development tools

SublimeText3 Mac version
God-level code editing software (SublimeText3)

Hot Topics


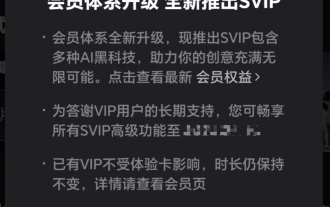
This site reported on June 27 that Jianying is a video editing software developed by FaceMeng Technology, a subsidiary of ByteDance. It relies on the Douyin platform and basically produces short video content for users of the platform. It is compatible with iOS, Android, and Windows. , MacOS and other operating systems. Jianying officially announced the upgrade of its membership system and launched a new SVIP, which includes a variety of AI black technologies, such as intelligent translation, intelligent highlighting, intelligent packaging, digital human synthesis, etc. In terms of price, the monthly fee for clipping SVIP is 79 yuan, the annual fee is 599 yuan (note on this site: equivalent to 49.9 yuan per month), the continuous monthly subscription is 59 yuan per month, and the continuous annual subscription is 499 yuan per year (equivalent to 41.6 yuan per month) . In addition, the cut official also stated that in order to improve the user experience, those who have subscribed to the original VIP
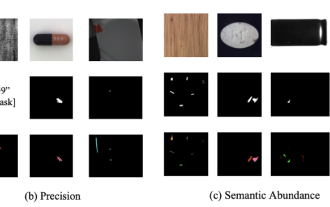
In modern manufacturing, accurate defect detection is not only the key to ensuring product quality, but also the core of improving production efficiency. However, existing defect detection datasets often lack the accuracy and semantic richness required for practical applications, resulting in models unable to identify specific defect categories or locations. In order to solve this problem, a top research team composed of Hong Kong University of Science and Technology Guangzhou and Simou Technology innovatively developed the "DefectSpectrum" data set, which provides detailed and semantically rich large-scale annotation of industrial defects. As shown in Table 1, compared with other industrial data sets, the "DefectSpectrum" data set provides the most defect annotations (5438 defect samples) and the most detailed defect classification (125 defect categories
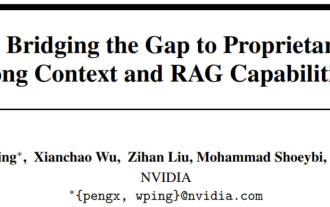
The open LLM community is an era when a hundred flowers bloom and compete. You can see Llama-3-70B-Instruct, QWen2-72B-Instruct, Nemotron-4-340B-Instruct, Mixtral-8x22BInstruct-v0.1 and many other excellent performers. Model. However, compared with proprietary large models represented by GPT-4-Turbo, open models still have significant gaps in many fields. In addition to general models, some open models that specialize in key areas have been developed, such as DeepSeek-Coder-V2 for programming and mathematics, and InternVL for visual-language tasks.
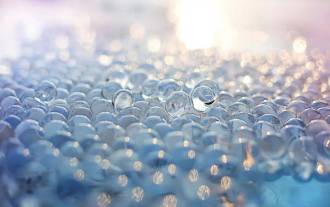
Editor |KX To this day, the structural detail and precision determined by crystallography, from simple metals to large membrane proteins, are unmatched by any other method. However, the biggest challenge, the so-called phase problem, remains retrieving phase information from experimentally determined amplitudes. Researchers at the University of Copenhagen in Denmark have developed a deep learning method called PhAI to solve crystal phase problems. A deep learning neural network trained using millions of artificial crystal structures and their corresponding synthetic diffraction data can generate accurate electron density maps. The study shows that this deep learning-based ab initio structural solution method can solve the phase problem at a resolution of only 2 Angstroms, which is equivalent to only 10% to 20% of the data available at atomic resolution, while traditional ab initio Calculation
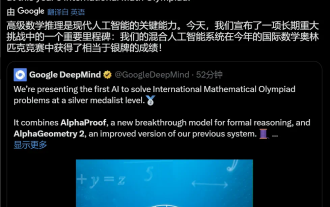
For AI, Mathematical Olympiad is no longer a problem. On Thursday, Google DeepMind's artificial intelligence completed a feat: using AI to solve the real question of this year's International Mathematical Olympiad IMO, and it was just one step away from winning the gold medal. The IMO competition that just ended last week had six questions involving algebra, combinatorics, geometry and number theory. The hybrid AI system proposed by Google got four questions right and scored 28 points, reaching the silver medal level. Earlier this month, UCLA tenured professor Terence Tao had just promoted the AI Mathematical Olympiad (AIMO Progress Award) with a million-dollar prize. Unexpectedly, the level of AI problem solving had improved to this level before July. Do the questions simultaneously on IMO. The most difficult thing to do correctly is IMO, which has the longest history, the largest scale, and the most negative
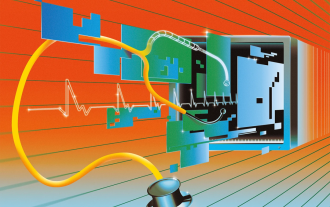
Editor | ScienceAI Based on limited clinical data, hundreds of medical algorithms have been approved. Scientists are debating who should test the tools and how best to do so. Devin Singh witnessed a pediatric patient in the emergency room suffer cardiac arrest while waiting for treatment for a long time, which prompted him to explore the application of AI to shorten wait times. Using triage data from SickKids emergency rooms, Singh and colleagues built a series of AI models that provide potential diagnoses and recommend tests. One study showed that these models can speed up doctor visits by 22.3%, speeding up the processing of results by nearly 3 hours per patient requiring a medical test. However, the success of artificial intelligence algorithms in research only verifies this
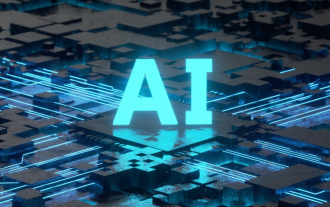
Editor |ScienceAI Question Answering (QA) data set plays a vital role in promoting natural language processing (NLP) research. High-quality QA data sets can not only be used to fine-tune models, but also effectively evaluate the capabilities of large language models (LLM), especially the ability to understand and reason about scientific knowledge. Although there are currently many scientific QA data sets covering medicine, chemistry, biology and other fields, these data sets still have some shortcomings. First, the data form is relatively simple, most of which are multiple-choice questions. They are easy to evaluate, but limit the model's answer selection range and cannot fully test the model's ability to answer scientific questions. In contrast, open-ended Q&A
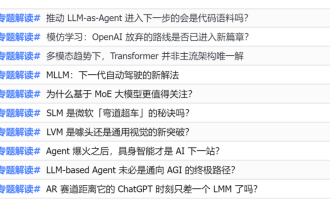
In 2023, almost every field of AI is evolving at an unprecedented speed. At the same time, AI is constantly pushing the technological boundaries of key tracks such as embodied intelligence and autonomous driving. Under the multi-modal trend, will the situation of Transformer as the mainstream architecture of AI large models be shaken? Why has exploring large models based on MoE (Mixed of Experts) architecture become a new trend in the industry? Can Large Vision Models (LVM) become a new breakthrough in general vision? ...From the 2023 PRO member newsletter of this site released in the past six months, we have selected 10 special interpretations that provide in-depth analysis of technological trends and industrial changes in the above fields to help you achieve your goals in the new year. be prepared. This interpretation comes from Week50 2023
