


To provide a new scientific and complex question answering benchmark and evaluation system for large models, UNSW, Argonne, University of Chicago and other institutions jointly launched the SciQAG framework
Editor | ScienceAI
Question and Answer (QA) data sets play a vital role in promoting natural language processing (NLP) research. High-quality QA data sets can not only be used to fine-tune models, but also effectively evaluate the capabilities of large language models (LLM), especially the ability to understand and reason about scientific knowledge.
Although there are currently many scientific QA data sets covering medicine, chemistry, biology and other fields, these data sets still have some shortcomings.
First, the data form is relatively simple, most of which are multiple-choice questions. They are easy to evaluate, but they limit the model’s answer selection range and cannot fully test the model’s ability to answer scientific questions. In contrast, open question answering (openQA) can more comprehensively evaluate the model's capabilities, but lacks suitable evaluation metrics.
Second, many of the contents of existing data sets come from textbooks at university level and below, making it difficult to evaluate LLM’s high-level knowledge retention capabilities in actual academic research or production environments.
Third, the creation of these benchmark datasets relies on human expert annotation.
Addressing these challenges is crucial to building a more comprehensive QA data set and is also conducive to more accurate assessment of scientific LLM.
Illustration: SciQAG framework for generating high-quality scientific question and answer pairs from scientific literature.
To this end, the Argonne National Laboratory in the United States, the team of Professor Ian Foster of the University of Chicago (2002 Gordon Bell Prize winner), the UNSW AI4Science team of Professor Bram Hoex of the University of New South Wales, Australia, the AI4Science company GreenDynamics and the team of Professor Jie Chunyu of the City University of Hong Kong jointly proposed SciQAG, the first novel framework to automatically generate high-quality scientific open question and answer pairs from large scientific literature corpora based on large language models (LLM).
Paper link:https://arxiv.org/abs/2405.09939
github link:https://github.com/MasterAI-EAM/SciQAG
Based on SciQAG, the researchers built SciQAG-24D, a large-scale, high-quality, open scientific QA dataset, contains 188,042 QA pairs extracted from 22,743 scientific papers in 24 scientific fields, and is designed to serve the fine-tuning of LLM and the assessment of scientific problem-solving capabilities.
Experiments demonstrate that fine-tuning LLMs on the SciQAG-24D dataset can significantly improve their performance in open-ended question answering and scientific tasks.
The data set, model and evaluation code have been open sourced (https://github.com/MasterAI-EAM/SciQAG) to promote the joint development of open scientific Q&A by the AI for Science community.
SciQAG framework with SciQAG-24D benchmark dataset
SciQAG consists of a QA generator and a QA evaluator, aiming to quickly generate diverse open question and answer pairs based on scientific literature at scale. First, the generator converts scientific papers into question and answer pairs, and then the evaluator filters out the question and answer pairs that do not meet the quality standards, thereby obtaining a high-quality scientific question and answer dataset.
QA Generator
The researchers designed a two-step prompt (prompt) through comparative experiments, allowing LLM to first extract keywords and then generate question and answer pairs based on the keywords.
Since the generated question and answer data set adopts the "closed book" mode, that is, the original paper is not provided and only focuses on the extracted scientific knowledge itself. The prompt requires that the generated question and answer pairs do not rely on or refer to the unique information in the original paper (for example, no modern nomenclature is allowed). Such as "this/this paper", "this/this research", etc., or asking questions about the tables/pictures in the article).
To balance performance and cost, the researchers chose to fine-tune an open source LLM as the generator. SciQAG users can choose any open source or closed source LLM as the generator according to their own circumstances, either using fine-tuning or prompt word engineering.
QA Evaluator
The evaluator is used to accomplish two purposes: (1) Evaluate the quality of generated question and answer pairs; (2) Discard low-quality question and answer pairs based on set criteria.
Researchers developed a comprehensive evaluation index RACAR, which consists of five dimensions: relevance, agnosticism, completeness, accuracy and reasonableness.
In this study, the researchers directly used GPT-4 as the QA evaluator to evaluate the generated QA pairs according to RACAR, with an evaluation level of 1-5 (1 means unacceptable, 5 means completely acceptable).
As shown in the figure, to measure the consistency between GPT-4 and manual evaluation, two domain experts used the RACAR metric to perform manual evaluation on 10 articles (a total of 100 question and answer pairs). Users can choose any open source or closed source LLM as an evaluator according to their needs.
Illustration: Spearman and Pearson correlations between GPT-4 assigned scores and expert annotation scores.
Application of SciQAG framework
This study obtained a total of 22,743 highly cited papers in 24 categories from the Web of Science (WoS) core collection database, from the fields of materials science, chemistry, physics, energy, etc., aiming to build a A reliable, rich, balanced and representative source of scientific knowledge.
To fine-tune the open source LLM to form a QA generator, the researchers randomly selected 426 papers from the paper collection as input and generated 4260 seed QA pairs by prompting GPT-4.
Using the trained QA generator to perform inference on the remaining papers, a total of 227,430 QA pairs (including seed QA pairs) were generated. Fifty papers were extracted from each category (1,200 papers in total), GPT-4 was used to calculate the RACAR score of each generated QA pair, and QA pairs with any dimension score lower than 3 were filtered out as the test set.
For the remaining QA pairs, a rule-based method is used to filter out all question and answer pairs that contain unique information of the paper to form a training set.
SciQAG-24D benchmark data set
Based on the above, researchers established the open scientific QA benchmark data set SciQAG-24D. The filtered training set includes 21,529 papers and 179,511 QA pairs, and the filtered The test set contains 1,199 papers and 8,531 QA pairs.
Statistics show that 99.15% of the data in the answers come from the original paper, 87.29% of the questions have a similarity below 0.3, and the answers cover 78.26% of the original content.
This data set is widely used: the training set can be used to fine-tune LLM and inject scientific knowledge into it; the test set can be used to evaluate the performance of LLM on open QA tasks in a specific or overall scientific field. Since the test set is larger, it can also be used as high-quality data for fine-tuning.
Illustration: The proportion of articles in different categories in the training and testing of the SciQAG-24D dataset.
Experimental results
The researchers conducted comprehensive experiments to compare the performance differences in scientific question answering between different language models and explore the impact of fine-tuning.
Zero-shot setting
The researchers used part of the test set in SciQAG-24D to conduct a zero-shot performance comparison of the five models. Two of them are open source LLMs: LLaMA1 (7B) and LLaMA2-chat (7B), and the rest are closed source LLMs.
Called via API: GPT3.5 (gpt-3.5-turbo), GPT-4 (gpt-4-1106-preview) and Claude 3 (claude-3-opus-20240229). Each model was prompted with 1,000 questions in the test, and its output was evaluated by the CAR metric (adapted from the RACAR metric, focusing only on response evaluation) to measure its zero-shot ability to answer scientific research questions.
As shown in the figure, among all models, GPT-4 has the highest score for completeness (4.90) and plausibility (4.99), while Claude 3 has the highest accuracy score (4.95). GPT-3.5 also performs very well, scoring closely behind GPT-4 and Claude 3 on all metrics.
Notably, LLaMA1 has the lowest scores in all three dimensions. In contrast, although the LLaMA2-chat model does not score as high as the GPT model, it significantly improves over the original LLaMA1 in all metrics. The results demonstrate the superior performance of commercial LLMs in answering scientific questions, while open source models (such as LLaMA2-chat) have also made significant progress in this regard.
Illustration: Zero-sample test and fine-tuning test (LLAMA1-QA) on SciQAG-24D
fine-tuning setting (fine-tuning setting)
The researchers selected LLaMA1 with the worst zero-sample performance Fine-tuning is performed on the training set of SciQAG-24D to obtain LLaMA1-QA. Through three experiments, the researchers demonstrated that SciQAG-24D can be used as effective fine-tuning data to improve the performance of downstream scientific tasks:
(a) LLaMA-QA versus original LLaMA1 on the unseen SciQAG-24D test set Performance comparison.
As shown in the figure above, the performance of LLaMA1-QA has been significantly improved compared to the original LLaMA1 (completeness increased by 13%, accuracy and plausibility increased by more than 30%). This shows that LLaMA1 has learned the logic of answering scientific questions from the training data of SciQAG-24D and internalized some scientific knowledge.
(b) Comparison of fine-tuning performance on SciQ, a scientific MCQ benchmark.
The first row of the table below shows that LLaMA1-QA is slightly better than LLaMA1 (+1%). According to observations, fine-tuning also enhanced the model's instruction following ability: the probability of unparsable output dropped from 4.1% in LLaMA1 to 1.7% in LLaMA1-QA.
(c) Comparison of fine-tuning performance on various scientific tasks.
In terms of evaluation indicators, F1-score is used for classification tasks, MAE is used for regression tasks, and KL divergence is used for transformation tasks. As shown in the table below, LLaMA1-QA has significant improvements compared to the LLaMA1 model in scientific tasks.
The most obvious improvement is reflected in the regression task, where the MAE dropped from 463.96 to 185.32. These findings suggest that incorporating QA pairs during training can enhance the model's ability to learn and apply scientific knowledge, thereby improving its performance in downstream prediction tasks.
Surprisingly, compared to specially designed machine learning models with features, LLM can achieve results comparable to or even surpass them in some tasks. For example, in the band gap task, although LLaMA1-QA does not perform as well as models such as MODNet (0.3327), it has surpassed AMMExpress v2020 (0.4161).
In the diversity task, LLaMA1-QA outperforms the deep learning baseline (0.3198). These findings indicate that LLM has great potential in specific scientific tasks.
Illustration: Fine-tuning performance of LLaMA1 and LLaMA1-QA on SciQ and scientific tasks (M represents multiple choice, C represents classification, R represents regression, T represents transformation)
Summary and Outlook
(1) SciQAG is a framework for generating QA pairs from scientific literature. Combined with the RACAR metric for evaluating and screening QA pairs, it can efficiently generate large amounts of knowledge-based QA data for resource-poor scientific fields.
(2) The team generated a comprehensive open source scientific QA dataset containing 188,042 QA pairs, called SciQAG-24D. The training set is used to fine-tune the LLM, and the test set evaluates the performance of the LLM on open-ended closed-book scientific QA tasks.
The zero-sample performance of several LLMs on the SciQAG-24D test set was compared, and LLaMA1 was fine-tuned on the SciQAG-24D training set to obtain LLaMA1-QA. This fine-tuning significantly improves its performance on multiple scientific tasks.
(3) Research shows that LLM has potential in scientific tasks, and the results of LLaMA1-QA can reach levels even exceeding the machine learning baseline. This demonstrates the multifaceted utility of SciQAG-24D and shows that incorporating scientific QA data into the training process can enhance LLM's ability to learn and apply scientific knowledge.
The above is the detailed content of To provide a new scientific and complex question answering benchmark and evaluation system for large models, UNSW, Argonne, University of Chicago and other institutions jointly launched the SciQAG framework. For more information, please follow other related articles on the PHP Chinese website!

Hot AI Tools

Undresser.AI Undress
AI-powered app for creating realistic nude photos

AI Clothes Remover
Online AI tool for removing clothes from photos.

Undress AI Tool
Undress images for free

Clothoff.io
AI clothes remover

Video Face Swap
Swap faces in any video effortlessly with our completely free AI face swap tool!

Hot Article

Hot Tools

Notepad++7.3.1
Easy-to-use and free code editor

SublimeText3 Chinese version
Chinese version, very easy to use

Zend Studio 13.0.1
Powerful PHP integrated development environment

Dreamweaver CS6
Visual web development tools

SublimeText3 Mac version
God-level code editing software (SublimeText3)

Hot Topics
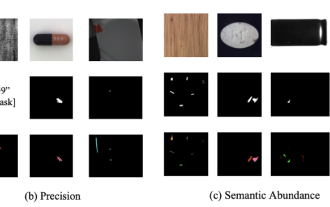
In modern manufacturing, accurate defect detection is not only the key to ensuring product quality, but also the core of improving production efficiency. However, existing defect detection datasets often lack the accuracy and semantic richness required for practical applications, resulting in models unable to identify specific defect categories or locations. In order to solve this problem, a top research team composed of Hong Kong University of Science and Technology Guangzhou and Simou Technology innovatively developed the "DefectSpectrum" data set, which provides detailed and semantically rich large-scale annotation of industrial defects. As shown in Table 1, compared with other industrial data sets, the "DefectSpectrum" data set provides the most defect annotations (5438 defect samples) and the most detailed defect classification (125 defect categories
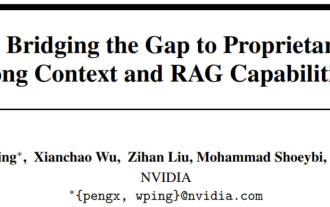
The open LLM community is an era when a hundred flowers bloom and compete. You can see Llama-3-70B-Instruct, QWen2-72B-Instruct, Nemotron-4-340B-Instruct, Mixtral-8x22BInstruct-v0.1 and many other excellent performers. Model. However, compared with proprietary large models represented by GPT-4-Turbo, open models still have significant gaps in many fields. In addition to general models, some open models that specialize in key areas have been developed, such as DeepSeek-Coder-V2 for programming and mathematics, and InternVL for visual-language tasks.
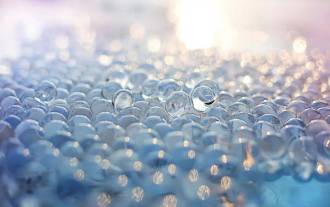
Editor |KX To this day, the structural detail and precision determined by crystallography, from simple metals to large membrane proteins, are unmatched by any other method. However, the biggest challenge, the so-called phase problem, remains retrieving phase information from experimentally determined amplitudes. Researchers at the University of Copenhagen in Denmark have developed a deep learning method called PhAI to solve crystal phase problems. A deep learning neural network trained using millions of artificial crystal structures and their corresponding synthetic diffraction data can generate accurate electron density maps. The study shows that this deep learning-based ab initio structural solution method can solve the phase problem at a resolution of only 2 Angstroms, which is equivalent to only 10% to 20% of the data available at atomic resolution, while traditional ab initio Calculation
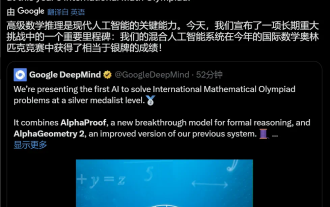
For AI, Mathematical Olympiad is no longer a problem. On Thursday, Google DeepMind's artificial intelligence completed a feat: using AI to solve the real question of this year's International Mathematical Olympiad IMO, and it was just one step away from winning the gold medal. The IMO competition that just ended last week had six questions involving algebra, combinatorics, geometry and number theory. The hybrid AI system proposed by Google got four questions right and scored 28 points, reaching the silver medal level. Earlier this month, UCLA tenured professor Terence Tao had just promoted the AI Mathematical Olympiad (AIMO Progress Award) with a million-dollar prize. Unexpectedly, the level of AI problem solving had improved to this level before July. Do the questions simultaneously on IMO. The most difficult thing to do correctly is IMO, which has the longest history, the largest scale, and the most negative
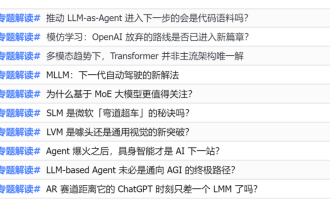
In 2023, almost every field of AI is evolving at an unprecedented speed. At the same time, AI is constantly pushing the technological boundaries of key tracks such as embodied intelligence and autonomous driving. Under the multi-modal trend, will the situation of Transformer as the mainstream architecture of AI large models be shaken? Why has exploring large models based on MoE (Mixed of Experts) architecture become a new trend in the industry? Can Large Vision Models (LVM) become a new breakthrough in general vision? ...From the 2023 PRO member newsletter of this site released in the past six months, we have selected 10 special interpretations that provide in-depth analysis of technological trends and industrial changes in the above fields to help you achieve your goals in the new year. be prepared. This interpretation comes from Week50 2023
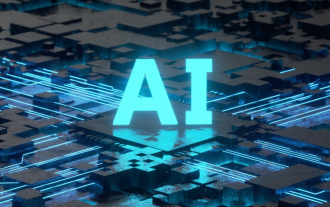
Editor |ScienceAI Question Answering (QA) data set plays a vital role in promoting natural language processing (NLP) research. High-quality QA data sets can not only be used to fine-tune models, but also effectively evaluate the capabilities of large language models (LLM), especially the ability to understand and reason about scientific knowledge. Although there are currently many scientific QA data sets covering medicine, chemistry, biology and other fields, these data sets still have some shortcomings. First, the data form is relatively simple, most of which are multiple-choice questions. They are easy to evaluate, but limit the model's answer selection range and cannot fully test the model's ability to answer scientific questions. In contrast, open-ended Q&A
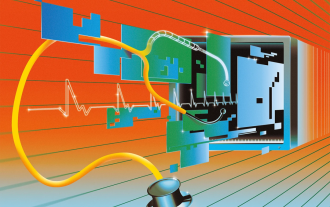
Editor | ScienceAI Based on limited clinical data, hundreds of medical algorithms have been approved. Scientists are debating who should test the tools and how best to do so. Devin Singh witnessed a pediatric patient in the emergency room suffer cardiac arrest while waiting for treatment for a long time, which prompted him to explore the application of AI to shorten wait times. Using triage data from SickKids emergency rooms, Singh and colleagues built a series of AI models that provide potential diagnoses and recommend tests. One study showed that these models can speed up doctor visits by 22.3%, speeding up the processing of results by nearly 3 hours per patient requiring a medical test. However, the success of artificial intelligence algorithms in research only verifies this

Editor | KX Retrosynthesis is a critical task in drug discovery and organic synthesis, and AI is increasingly used to speed up the process. Existing AI methods have unsatisfactory performance and limited diversity. In practice, chemical reactions often cause local molecular changes, with considerable overlap between reactants and products. Inspired by this, Hou Tingjun's team at Zhejiang University proposed to redefine single-step retrosynthetic prediction as a molecular string editing task, iteratively refining the target molecular string to generate precursor compounds. And an editing-based retrosynthetic model EditRetro is proposed, which can achieve high-quality and diverse predictions. Extensive experiments show that the model achieves excellent performance on the standard benchmark data set USPTO-50 K, with a top-1 accuracy of 60.8%.
