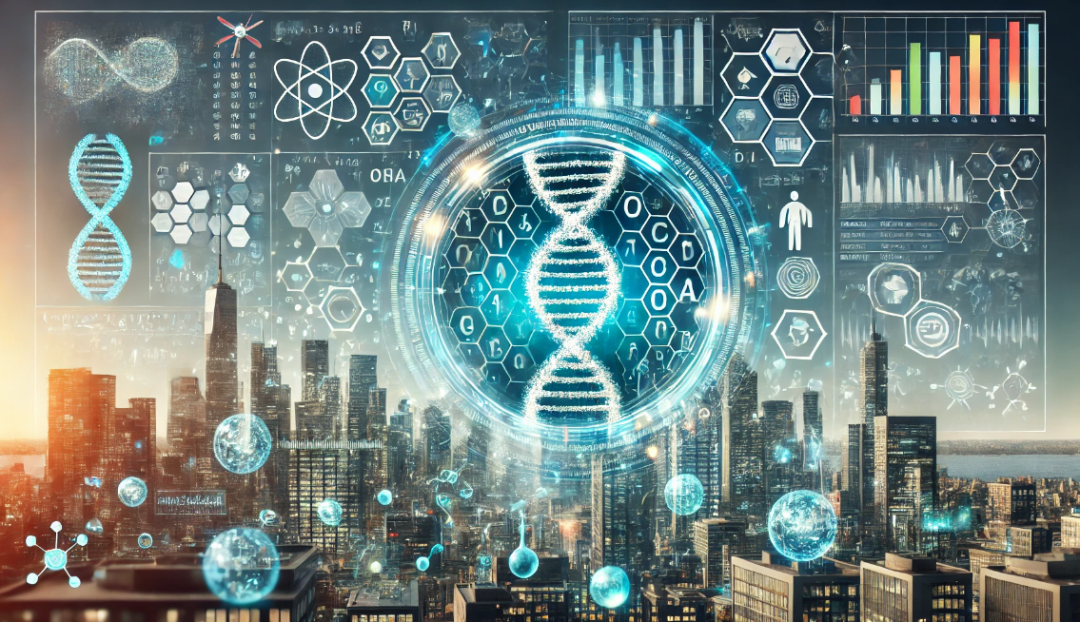
Editor | Radish Skin
Understanding protein function and developing molecular therapies requires identifying the cell types in which proteins play a role and analyzing the interactions between proteins.
However, modeling protein interactions across biological contexts remains challenging for existing algorithms.
In the latest study, researchers at Harvard Medical School developed PINNACLE, a geometric deep learning method for generating context-aware protein representations.
PINNACLE leverages multi-organ single cell atlases to learn on contextualized protein interaction networks, generating 394,760 protein representations from 156 cell type contexts across 24 tissues.
The study was titled "Contextual AI models for single-cell protein biology" and was published in "Nature Methods" on July 22, 2024.
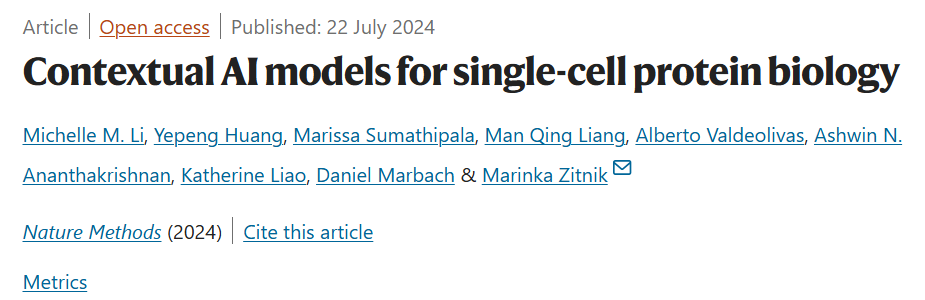
Protein Function and Network
- Proteins are the basic functional units of cells and achieve biological functions through interactions.
- High-throughput technologies have driven the mapping of protein interaction networks and improved understanding of protein structure, function and target design through computational methods.
- Indicates that the learning method integrates molecular cell atlases, can analyze protein interaction networks, and expands the understanding of protein functions.
Context-dependent protein function
- Proteins play different roles in different biological contexts, and gene expression and function vary according to health and disease states.
- Background-free protein means that functional changes between cell types cannot be identified, affecting prediction accuracy.
Single Cell Gene Expression and Protein Network
- Sequencing technology measures single cell gene expression, paving the way for solving context-dependent problems.
- Attention-based deep learning can focus on large inputs and learn important elements in the context.
- Single cell atlas can enhance the mapping of gene regulatory networks related to disease progression and reveal targets.
PINNACLE MODEL
- There are still challenges in integrating protein-coding gene expression into protein interaction networks.
- PINNACLE model provides context-specific understanding of proteins.
- PINNACLE is a geometric deep learning model that generates protein representations by analyzing protein interactions in cellular environments.
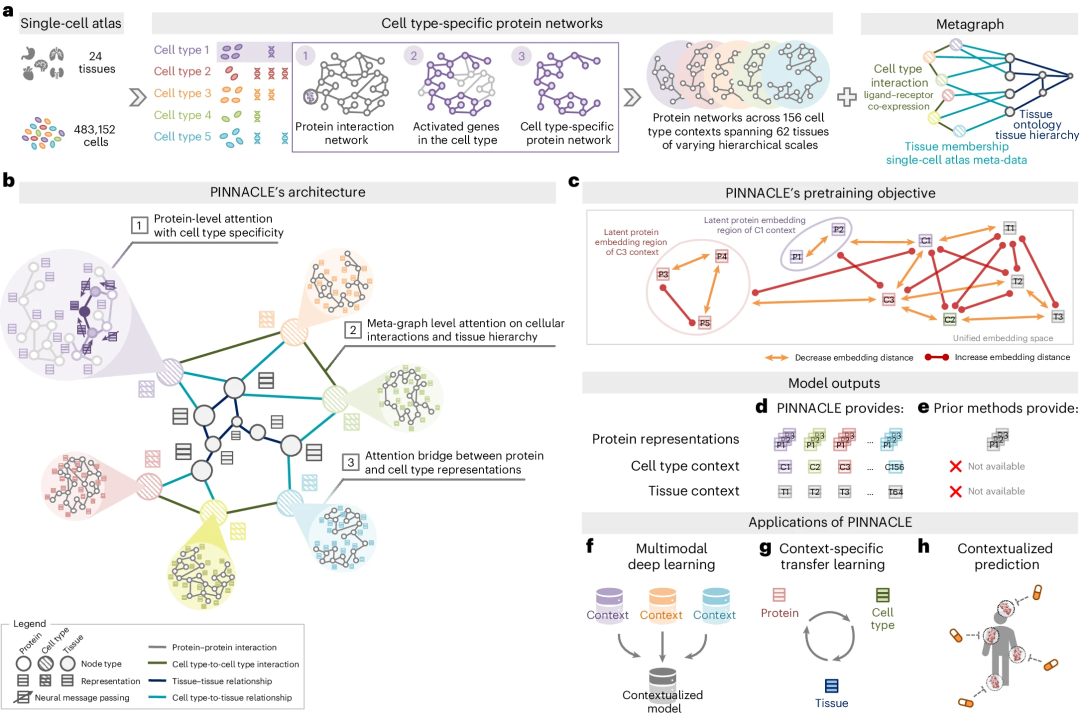
1. PINNACLE Overview
Illustration: PINNACLE Overview. (Source: Paper)
2. Contextualized Protein Representation
PINNACLE is trained on an integrated context-aware PPI network, supplemented by a network that captures cellular interactions and tissue hierarchies, to generate protein representations customized for cell types .
3. Multi-scale representation
Unlike context-free models, PINNACLE generates multiple representations for each protein, depending on its cell type context. Additionally, PINNACLE generates cell type context and tissue-level representations.
4. Multi-scale learning
PINNACLE learns the topology of proteins, cell types and tissues by optimizing a unified latent representation space.
5. Context-Aware Models
PINNACLE integrates context-specific data into a single model and transfers knowledge between protein, cell type and tissue-level data.
6. Embedding Space
To inject cellular and tissue information into the embedding space, PINNACLE employs protein, cell type, and tissue level attention.
7. Physical interaction mapping
Physically interacting protein pairs are tightly embedded in the embedding space.
8. Cell Type Environment
Proteins are embedded near their cell type environment.
9. Graph Neural Network Propagation
PINNACLE propagates information between proteins, cell types and tissues using an attention mechanism customized for each node and edge type.

Illustration: Enrichment of PINNACLE protein embedded regions. (Source: paper) Protein-level pre-training tasks consider self-supervised link prediction of protein interactions and cell type classification of protein nodes. These tasks enable PINNACLE to shape an embedding space that encapsulates the topology of context-aware protein interaction networks and the cell type identity of proteins.
PINNACLE’s cell-type and tissue-specific pre-training tasks rely entirely on self-supervised link prediction to facilitate learning of cell and tissue organization. The topology of cell types and tissues is passed to the protein representation through an attention bridging mechanism, effectively reinforcing tissue and cell organization onto the protein representation.
PINNACLE’s contextualized protein representation captures the structure of context-aware protein interaction networks. The regional arrangement of these contextualized protein representations in latent space reflects the cellular and tissue organization represented by the metagraph. This will lead to a comprehensive and context-specific representation of proteins within a unified cell-type and tissue-specific framework.
With 394,760 contextualized protein representations generated by PINNACLE, each of which is cell type specific, researchers demonstrate PINNACLE's ability to combine protein interactions with the underlying protein-coding gene transcriptomes of 156 cell type contexts.
PINNACLE’s embedding space reflects cellular and tissue structures, enabling zero-shot retrieval of tissue hierarchies. Pretrained protein representations can be adapted to downstream tasks: enhance 3D structure-based representations to resolve immuno-oncology protein interactions and study the effects of drugs on different cell types.
PINNACLE outperforms state-of-the-art models in specifying therapeutic targets for rheumatoid arthritis and inflammatory bowel disease, and has higher predictive power than context-free models to pinpoint cell type context. PINNACLE's ability to adapt its output to the environment in which it operates paves the way for large-scale context-specific predictions in biology.
Paper link: https://www.nature.com/articles/s41592-024-02341-3
The above is the detailed content of Generating 394,760 protein representations, Harvard team develops AI model to fully understand protein context. For more information, please follow other related articles on the PHP Chinese website!