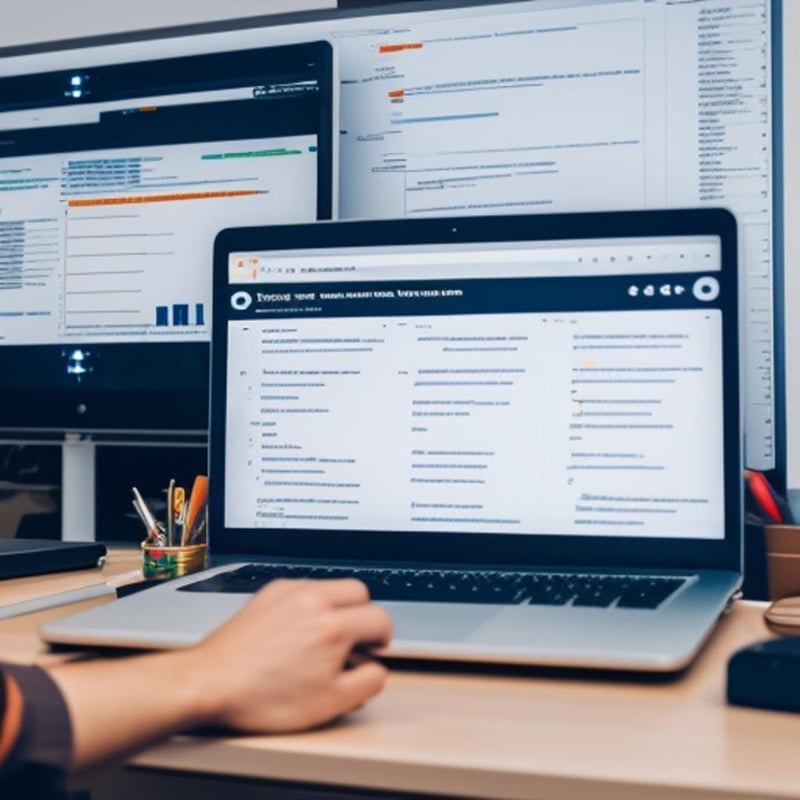
Hey there, I'm excited to share some insights about test coverage tools with you today. As a tech entrepreneur in the software development space, I've seen firsthand how crucial these tools are for maintaining code quality and ensuring smooth releases. Let's dive in.
What Are Test Coverage Tools?
Test coverage tools help measure how much of your code is tested by your automated tests. They highlight the parts of your codebase that have been covered by tests and, more importantly, the parts that haven't. This gives you a clear idea of where your tests are lacking and where potential bugs might be hiding.
Why Test Coverage Matters
In our fast-paced development world, ensuring your code is robust and bug-free is non-negotiable. Without proper test coverage, you risk shipping code that's poorly tested, leading to bugs that can frustrate users and damage your reputation.
Imagine releasing a new feature only to find out it breaks existing functionality because it wasn't properly tested. That's a nightmare scenario we've all faced, and good test coverage can help prevent it.
Popular Test Coverage Tools
- JaCoCo (Java Code Coverage): If you're working with Java, JaCoCo is a go-to. It integrates smoothly with build tools like Maven and Gradle. When we started using JaCoCo, our test coverage insights improved drastically. We could easily spot gaps and write tests to cover them.
- Istanbul (JavaScript): For JavaScript projects, Istanbul is pretty popular. It integrates well with test frameworks like Mocha, Jest, and Jasmine. Using Istanbul on our JavaScript projects helped us identify untested code and improve overall coverage.
- Cobertura: Another Java coverage tool, Cobertura is easy to set up and provides detailed reports. We used it in one of our legacy projects, and it was invaluable in highlighting untested parts of our codebase.
- Coverage.py: If you’re into Python, Coverage.py is a must-have. It’s straightforward and integrates well with most CI tools. We saw a significant improvement in our Python projects' test coverage after implementing it.
How to Implement Test Coverage Tools
- Choose the Right Tool: Pick a tool that fits your tech stack. For instance, JaCoCo for Java, Istanbul for JavaScript, and Coverage.py for Python.
- Integrate with CI/CD: Ensure your test coverage tool is part of your CI/CD pipeline. This way, you get regular updates on your test coverage every time you push new code.
- Set Coverage Targets: Define clear test coverage goals for your team. Aim for at least 80% coverage. But remember, 100% coverage doesn’t guarantee bug-free code, so focus on meaningful tests.
- Review and Improve: Regularly review coverage reports and address any uncovered code. Encourage your team to write tests for these areas.
Challenges with Test Coverage
- False Sense of Security: High test coverage doesn’t mean your code is bug-free. It’s easy to get complacent with high coverage numbers. Focus on writing quality tests, not just increasing coverage percentages.
- Performance Overheads: Running coverage tools can slow down your build process. This is especially true for large projects. We experienced this firsthand and had to optimize our build pipeline to balance coverage checks and build times.
- Maintaining Coverage: As your codebase grows, maintaining test coverage can be challenging. Regularly refactoring tests and code can help keep coverage high.
Best Practices
- Start Early: Integrate test coverage tools from the beginning of your project. It’s easier to maintain coverage than to catch up later.
- Involve the Whole Team: Make test coverage a team effort. Everyone should be responsible for writing tests, not just a few dedicated testers.
- Review and Educate: Regularly review coverage reports with your team. Conduct training sessions if necessary to ensure everyone understands how to write effective tests.
- Use Coverage as a Guide: Use coverage reports to guide your testing efforts, not dictate them. Focus on critical paths and high-risk areas.
Final Thoughts
Test coverage tools are essential for ensuring the quality and reliability of your software. They provide visibility into what parts of your code are tested and what parts aren’t, helping you catch potential issues early. By choosing the right tools, integrating them into your workflow, and following best practices, you can significantly improve your test coverage and, ultimately, the quality of your code.
Remember, the goal isn’t just high coverage numbers but meaningful, effective tests that ensure your software works as intended. So, pick the right tools, set clear goals, and keep testing. Your users will thank you for it.
The above is the detailed content of Understanding test coverage tools. For more information, please follow other related articles on the PHP Chinese website!