


36 times higher than the original resolution, teams from Beihang University and Tsinghua University used AI to characterize tissues at high resolution on a multi-space omics platform, published in the Nature sub-journal
1. Introduction
Spatial omics has expanded the scope of molecular class analysis, but many techniques are limited by spatial resolution. Existing computational methods are mainly targeted at transcriptomic data and lack adaptability to emerging spatial omics technologies.
2. soScope framework
Researchers from Beihang University and Tsinghua University proposed soScope, a unified generation framework designed to improve the quality and resolution of spatial omics data.
3. Technical Principle
soScope summarizes multimodal tissue information from omics, spatial relationships and images. Output omics spectra with enhanced resolution through joint inference of distribution priors and omics-specific modeling.
4. Performance evaluation
The evaluation results of soScope on Visium, Xenium, spatial-CUT&Tag, slide-DNA/RNA-seq and other platforms show that:
- improves the performance of intestinal and kidney structure identification
- Revealed the fine structure of the embryonic heart
- Corrected for sample and technical bias
5. Extended applications
soScope has been extended to spatial-CITE-seq and spatial ATAC-RNA-seq, leveraging cross-omics references for multi- Omic enhancement.
6. Conclusion
soScope provides a versatile tool that improves the utilization of spatial omics technology and resources.
7. Reference
This research was published in "Nature Communications" on August 2, 2024 under the title "Tissue characterization at an enhanced resolution across spatial omics platforms with deep generative model".
Tissues are composed of cells with different molecular states and spatial organizations. Spatial omics technology has made significant progress in recent years, allowing spatial analysis of various molecular classes while maintaining spatial context.
Challenges and Solutions
Despite early success, spatial omics technology still faces two major challenges:
- Frozen or formalin-fixed tissue may affect the molecular state and reduce sequencing accuracy.
- Most techniques have limited spatial resolution, making it difficult to reveal subtle heterogeneities in tissue structure.
Computing technology can improve the resolution of spatial omics data, but most current methods only target a single tissue modality, making it difficult to fully utilize multi-modal information.
soScope: Improving spatial resolution and data quality
Research teams from Beihang University and Tsinghua University introduce Spatiomic Scope (soScope), a fully generative framework that simulates point-level data from different spatial omics technologies The profile generation process aims to improve their spatial resolution and data quality.
soScope treats each point as a collection of "sub-points" with enhanced spatial resolution, whose omics characteristics are related to spatial location and morphological patterns. SoScope then uses a multimodal deep learning framework to integrate spot omics profiles, spatial relationships, and high-resolution morphology images and jointly infer omics profiles at sub-spot resolution. By selecting omics-specific distributions, soScope can accurately model and reduce variation in different spatial omics data.
soScope Features:
- Unified tool, combined with multi-modal tissue maps
- Enhanced omics maps (different molecular classes)
- Improve spatial resolution
- Reduce unnecessary changes
- Characterization Complex tissue structures (undetectable at native resolution)
Illustration: Evaluation of soScope on spatial transcriptomic datasets from multiple tissues and platforms. (Source: Paper)
The team extensively evaluated soScope’s effectiveness and generalizability for multiple molecular types analyzed by multiple spatial techniques, including Visium, Xenium, spatial-CUT&Tag, slide-DNA-seq, slide- RNA-seq, spatial-CITE-seq and spatial ATAC-RNA-seq.
In healthy and diseased tissues, soScope improves tissue domain identification, increases the differentiability of known markers, and corrects for data and technical biases. The method is able to reveal finer tissue structures up to 36 times greater than the original resolution. It can efficiently adapt spatial multi-omics data to simultaneously enhance multi-omics profiles.
Researchers note that there are several imaging-based spatial omics technologies, such as seqFISH, STARmap and MERFISH, which can directly achieve spatial analysis at single-cell resolution, but at the expense of lower omics throughput and smaller tissue areas . Although soScope provides enhanced profiles for prespecified subspot or cellular locations, it may not achieve subcellular resolution.
Further improve resolution:
- Modify soScope to include paired single-cell omics data from the same tissue, providing higher resolution information for sub-point inference.
- Integrate H&E images as input, which can be easily annotated by human experts in certain clinical studies.
- Modify soScope to integrate human labels and guide posterior inference in a semi-supervised manner, improving latent representation and profile learning.
Reduce computational costs:
For larger data sets containing multiple contiguous slices from the same organ, soScope can:
- Train on partial data.
- Apply to remaining tissue sections.
Potential:
With the continuous expansion of spatial omics data resources and the emergence of new spatial technologies, researchers believe that soScope has the following potential:
- A versatile tool.
- Make full use of spatial omics data.
- Enhance scientists’ understanding of complex tissue structures and biological processes.
Paper link:
https://www.nature.com/articles/s41467-024-50837-5
The above is the detailed content of 36 times higher than the original resolution, teams from Beihang University and Tsinghua University used AI to characterize tissues at high resolution on a multi-space omics platform, published in the Nature sub-journal. For more information, please follow other related articles on the PHP Chinese website!

Hot AI Tools

Undresser.AI Undress
AI-powered app for creating realistic nude photos

AI Clothes Remover
Online AI tool for removing clothes from photos.

Undress AI Tool
Undress images for free

Clothoff.io
AI clothes remover

Video Face Swap
Swap faces in any video effortlessly with our completely free AI face swap tool!

Hot Article

Hot Tools

Notepad++7.3.1
Easy-to-use and free code editor

SublimeText3 Chinese version
Chinese version, very easy to use

Zend Studio 13.0.1
Powerful PHP integrated development environment

Dreamweaver CS6
Visual web development tools

SublimeText3 Mac version
God-level code editing software (SublimeText3)

Hot Topics










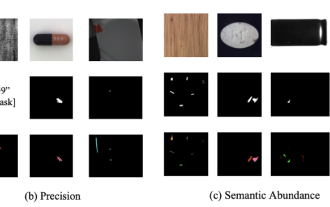
In modern manufacturing, accurate defect detection is not only the key to ensuring product quality, but also the core of improving production efficiency. However, existing defect detection datasets often lack the accuracy and semantic richness required for practical applications, resulting in models unable to identify specific defect categories or locations. In order to solve this problem, a top research team composed of Hong Kong University of Science and Technology Guangzhou and Simou Technology innovatively developed the "DefectSpectrum" data set, which provides detailed and semantically rich large-scale annotation of industrial defects. As shown in Table 1, compared with other industrial data sets, the "DefectSpectrum" data set provides the most defect annotations (5438 defect samples) and the most detailed defect classification (125 defect categories
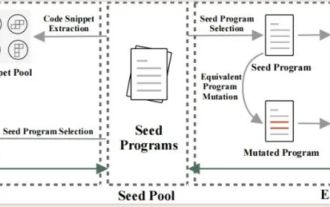
According to news from this site on June 22, Huawei yesterday introduced Huawei’s self-developed programming language-Cangjie to developers around the world. This is the first public appearance of Cangjie programming language. According to inquiries on this site, Tianjin University and Beijing University of Aeronautics and Astronautics were deeply involved in the research and development of Huawei’s “Cangjie”. Tianjin University: Cangjie Programming Language Compiler The software engineering team of the Department of Intelligence and Computing of Tianjin University joined hands with the Huawei Cangjie team to deeply participate in the quality assurance research of the Cangjie programming language compiler. According to reports, the Cangjie compiler is the basic software that is symbiotic with the Cangjie programming language. In the preparatory stage of the Cangjie programming language, a high-quality compiler that matches it became one of the core goals. As the Cangjie programming language evolves, the Cangjie compiler is constantly being upgraded and improved. In the past five years, Tianjin University
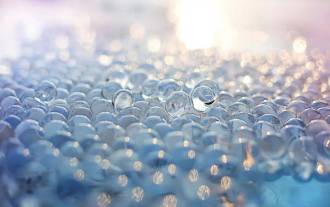
Editor |KX To this day, the structural detail and precision determined by crystallography, from simple metals to large membrane proteins, are unmatched by any other method. However, the biggest challenge, the so-called phase problem, remains retrieving phase information from experimentally determined amplitudes. Researchers at the University of Copenhagen in Denmark have developed a deep learning method called PhAI to solve crystal phase problems. A deep learning neural network trained using millions of artificial crystal structures and their corresponding synthetic diffraction data can generate accurate electron density maps. The study shows that this deep learning-based ab initio structural solution method can solve the phase problem at a resolution of only 2 Angstroms, which is equivalent to only 10% to 20% of the data available at atomic resolution, while traditional ab initio Calculation
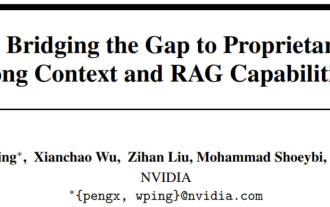
The open LLM community is an era when a hundred flowers bloom and compete. You can see Llama-3-70B-Instruct, QWen2-72B-Instruct, Nemotron-4-340B-Instruct, Mixtral-8x22BInstruct-v0.1 and many other excellent performers. Model. However, compared with proprietary large models represented by GPT-4-Turbo, open models still have significant gaps in many fields. In addition to general models, some open models that specialize in key areas have been developed, such as DeepSeek-Coder-V2 for programming and mathematics, and InternVL for visual-language tasks.
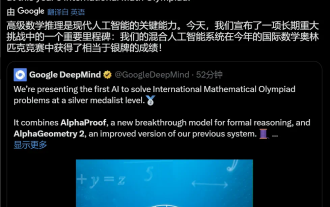
For AI, Mathematical Olympiad is no longer a problem. On Thursday, Google DeepMind's artificial intelligence completed a feat: using AI to solve the real question of this year's International Mathematical Olympiad IMO, and it was just one step away from winning the gold medal. The IMO competition that just ended last week had six questions involving algebra, combinatorics, geometry and number theory. The hybrid AI system proposed by Google got four questions right and scored 28 points, reaching the silver medal level. Earlier this month, UCLA tenured professor Terence Tao had just promoted the AI Mathematical Olympiad (AIMO Progress Award) with a million-dollar prize. Unexpectedly, the level of AI problem solving had improved to this level before July. Do the questions simultaneously on IMO. The most difficult thing to do correctly is IMO, which has the longest history, the largest scale, and the most negative
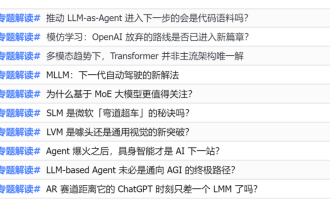
In 2023, almost every field of AI is evolving at an unprecedented speed. At the same time, AI is constantly pushing the technological boundaries of key tracks such as embodied intelligence and autonomous driving. Under the multi-modal trend, will the situation of Transformer as the mainstream architecture of AI large models be shaken? Why has exploring large models based on MoE (Mixed of Experts) architecture become a new trend in the industry? Can Large Vision Models (LVM) become a new breakthrough in general vision? ...From the 2023 PRO member newsletter of this site released in the past six months, we have selected 10 special interpretations that provide in-depth analysis of technological trends and industrial changes in the above fields to help you achieve your goals in the new year. be prepared. This interpretation comes from Week50 2023
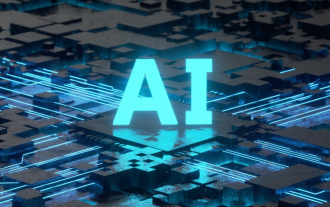
Editor |ScienceAI Question Answering (QA) data set plays a vital role in promoting natural language processing (NLP) research. High-quality QA data sets can not only be used to fine-tune models, but also effectively evaluate the capabilities of large language models (LLM), especially the ability to understand and reason about scientific knowledge. Although there are currently many scientific QA data sets covering medicine, chemistry, biology and other fields, these data sets still have some shortcomings. First, the data form is relatively simple, most of which are multiple-choice questions. They are easy to evaluate, but limit the model's answer selection range and cannot fully test the model's ability to answer scientific questions. In contrast, open-ended Q&A

Editor | KX Retrosynthesis is a critical task in drug discovery and organic synthesis, and AI is increasingly used to speed up the process. Existing AI methods have unsatisfactory performance and limited diversity. In practice, chemical reactions often cause local molecular changes, with considerable overlap between reactants and products. Inspired by this, Hou Tingjun's team at Zhejiang University proposed to redefine single-step retrosynthetic prediction as a molecular string editing task, iteratively refining the target molecular string to generate precursor compounds. And an editing-based retrosynthetic model EditRetro is proposed, which can achieve high-quality and diverse predictions. Extensive experiments show that the model achieves excellent performance on the standard benchmark data set USPTO-50 K, with a top-1 accuracy of 60.8%.
