The AIxiv column is a column where this site publishes academic and technical content. In the past few years, the AIxiv column of this site has received more than 2,000 reports, covering top laboratories from major universities and companies around the world, effectively promoting academic exchanges and dissemination. If you have excellent work that you want to share, please feel free to contribute or contact us for reporting. Submission email: liyazhou@jiqizhixin.com; zhaoyunfeng@jiqizhixin.com
Large-scale language models represented by GPT herald the dawn of general artificial intelligence in the digital cognitive space. These models demonstrate powerful understanding and reasoning capabilities by processing and generating natural language, and have shown broad application prospects in multiple fields. Whether in content generation, automated customer service, productivity tools, AI search, or in fields such as education and medical care, large-scale language models are constantly promoting the advancement of technology and the popularization of applications. However, to promote general artificial intelligence to explore the physical world, the first step is to solve the problem of visual understanding, that is, multi-modal understanding of large models. Multimodal understanding enables AI to more fully understand and interact with the world by acquiring and processing information through multiple senses, just like humans. Breakthroughs in this field will enable artificial intelligence to make greater progress in robotics, autonomous driving, etc., and truly realize the leap from the digital world to the physical world. GPT-4V was released in June last year, but compared to large language models, the development of multi-modal understanding models appears to be slower, especially in the Chinese field. In addition, unlike the technical route and selection of large language models that are relatively certain, the industry has not yet fully reached a consensus on the architecture and selection of training methods for multi-modal models. 
dollars dollars dollars dollars dollars dollars State-of-the-art understanding of large models. The model has been innovative and deeply optimized in terms of architecture, training methods and data processing, significantly improving its performance and supporting the understanding of images with any aspect ratio and up to 7K resolution. Unlike most multi-modal models that are mainly tuned in open source benchmarks, Tencent's hybrid multi-modal model pays more attention to the versatility, practicality and reliability of the model, and has rich multi-modal scene understanding capabilities. In the recently released Chinese multi-modal large model SuperCLUE-V benchmark evaluation (August 2024), Tencent Hunyuan ranked first in the country, surpassing multiple mainstream closed-source models.
Method introduction: MoE architecture
Tencent’s large mixed language model is the first in China to adopt the mixed expert model (MoE) architecture. The overall performance of the model is 50% higher than the previous generation, and some Chinese capabilities It has tied up with GPT-4o, and has greatly improved its performance in answering "current" questions, as well as in mathematics, reasoning and other abilities. As early as the beginning of this year, Tencent Hunyuan applied this model to Tencent Yuanbao. Tencent Hunyuan believes that the MoE architecture that can solve a large number of general tasks is also the best choice for multi-modal understanding scenarios. MoE can be better compatible with more modalities and tasks, ensuring that different modalities and tasks are mutually reinforcing rather than competitive.
Relying on the capabilities of Tencent Hunyuan's large language model, Tencent Hunyuan has launched a large multi-modal understanding model based on MoE architecture. It has made innovations and in-depth optimizations in terms of architecture, training methods and data processing, and its performance has been significantly improved. promote. This is also the first multi-modal large model based on MoE architecture in China.
模 Tencent mixed element multi -modal model architecture schematic diagram In addition to using the MOE architecture, the design of the Tencent mixed element multi -mode model also follows simple and reasonable , Principles of scalability:
Support native arbitrary resolutions: Compared with the industry’s mainstream fixed resolution or cut subgraph methods, Tencent’s hybrid multi-modal model can process native images of any resolution. Implemented the first multi-modal model to support image understanding with resolutions exceeding 7K and any aspect ratio (e.g. 16:1, see example below).
-
Using a simple MLP adapter: Compared with the previous mainstream Q-former adapter, the MLP adapter has less loss during information transmission.
This simple design makes it easier to expand and scale models and data.
SuperClue-V ranks first in the domestic listIn August 2024, SuperCLUE released the multi-modal understanding evaluation list for the first time - SuperClue-V.
The SuperCLUE-V benchmark includes two general directions: basic capabilities and application capabilities. It evaluates multi-modal large models in the form of open questions, including 8 first-level dimensions and 30 second-level dimensions.
In this evaluation, the Hunyuan multi-modal understanding system hunyuan-vision achieved a score of 71.95, second only to GPT-4o. In terms of multi-modal applications, hunyuan-vision is ahead of Claude3.5-Sonnet and Gemini-1.5-Pro. 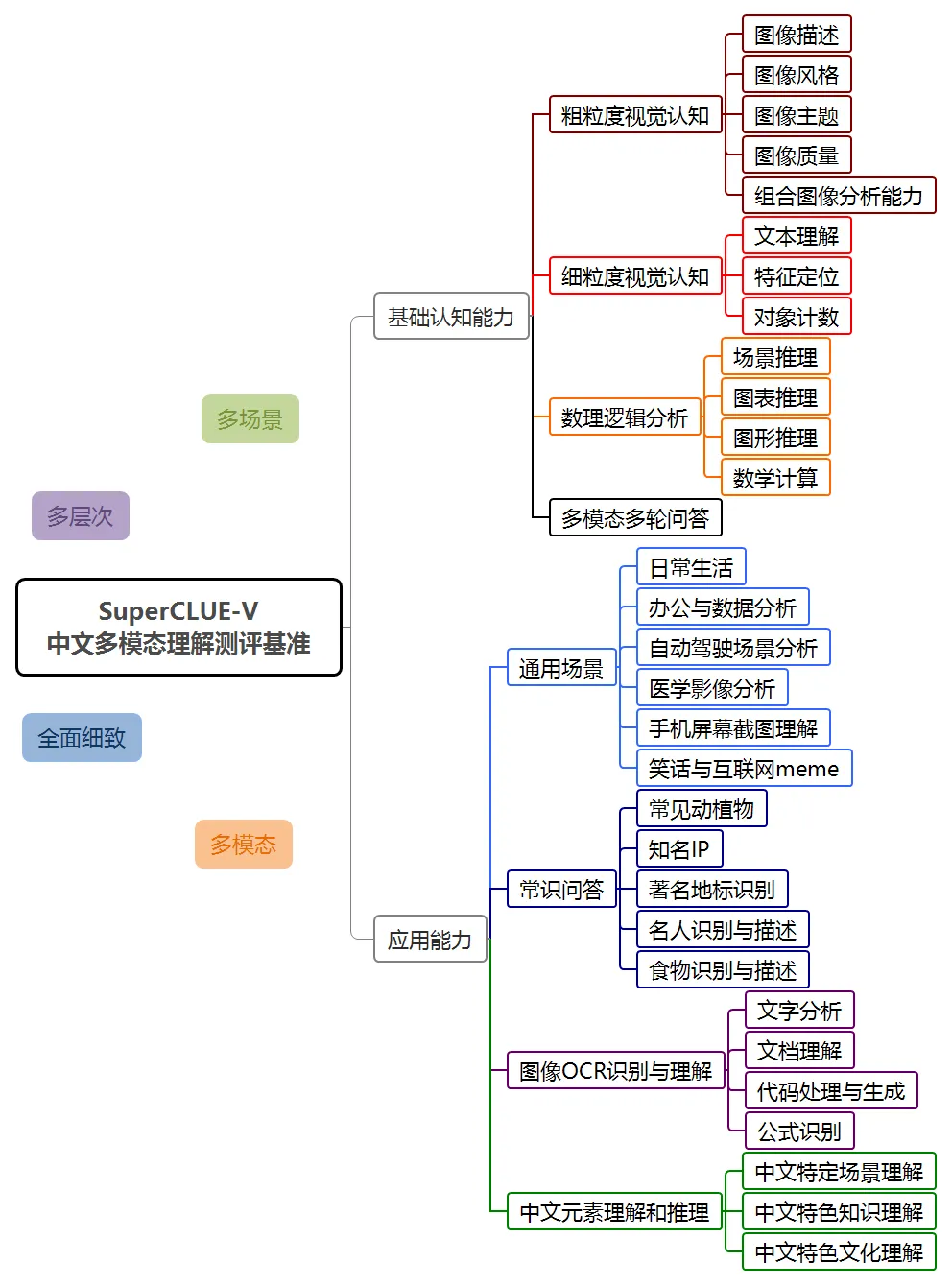
It is worth noting that previous multi-modal assessments in the industry mostly focused on English proficiency, and most of the assessment questions were multiple-choice or true-false questions. The SuperCLUE-V evaluation focuses more on Chinese proficiency evaluation and focuses on users’ real problems. In addition, since this is the first release, overfitting has not yet occurred.
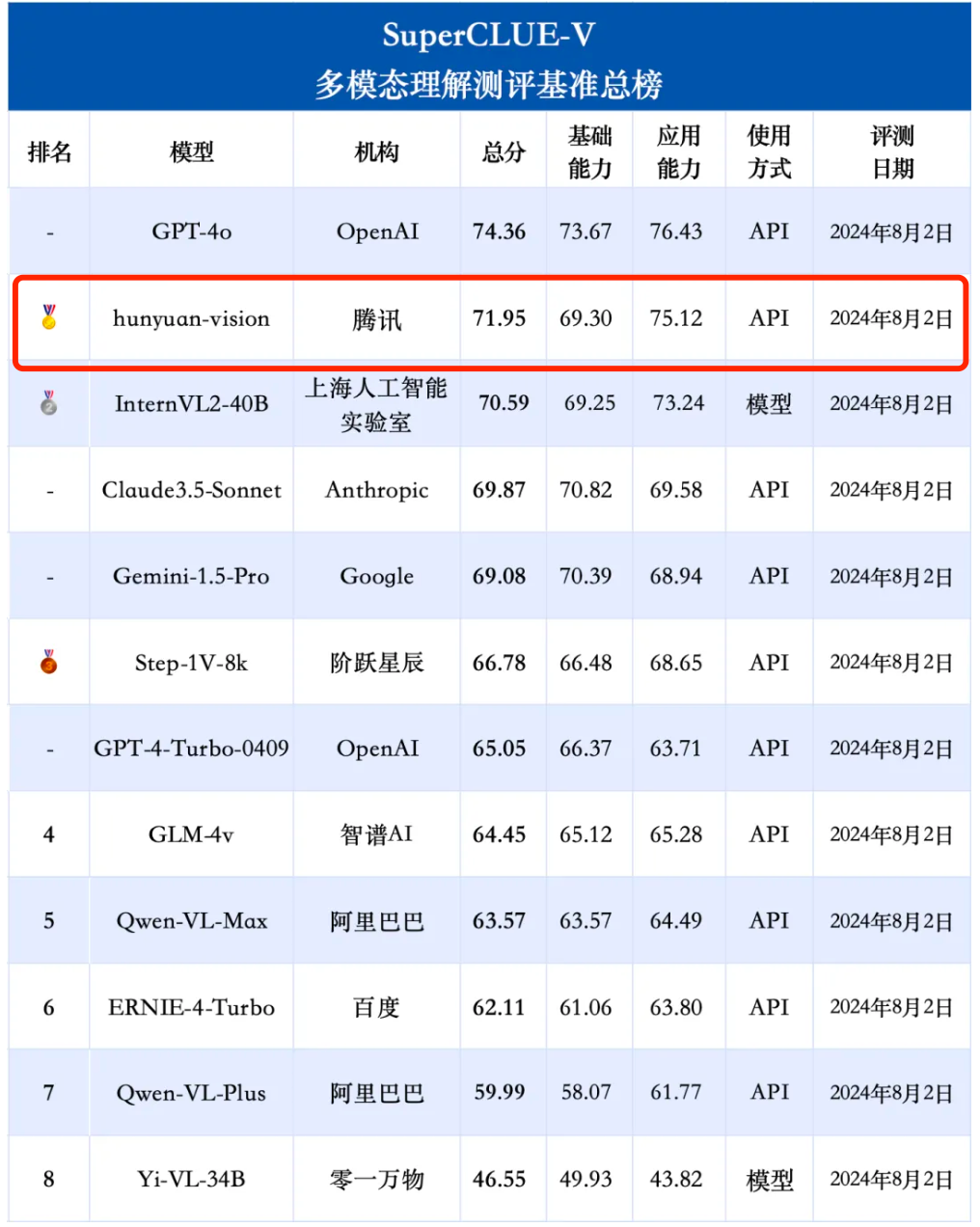
Tencent Hunyuan Graphics and Text Large Model shows good performance in multiple dimensions such as general scenes, image OCR recognition and understanding, and Chinese element understanding and reasoning, and also reflects the potential of the model in future applications. 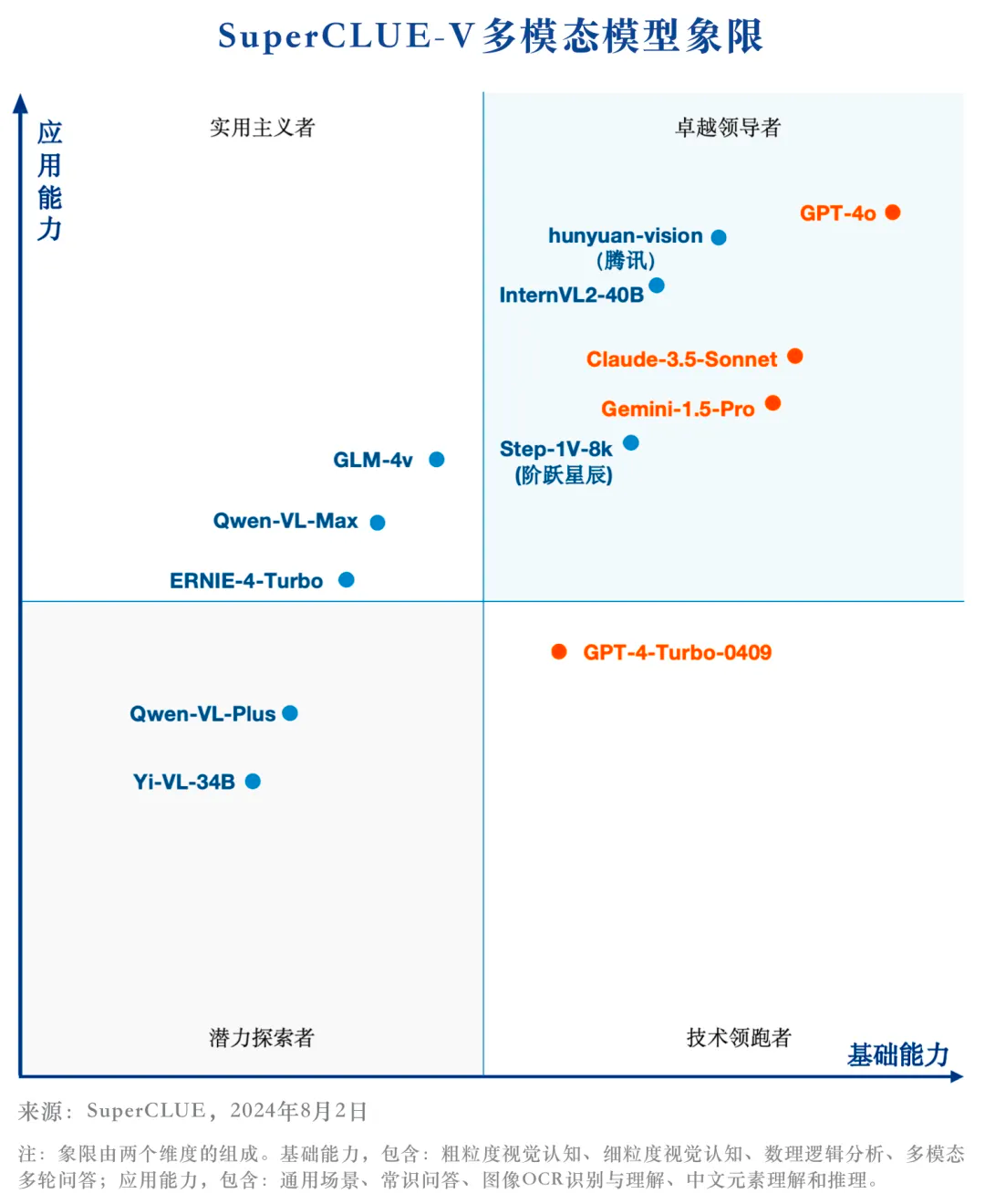
Aimed at general application scenarios
The mixed-element multi-modal understanding model is optimized for general scenarios and massive applications, and has accumulated tens of millions of related question and answer corpus, covering basic image understanding, content creation, It can be used in many scenarios such as reasoning analysis, knowledge question and answer, OCR document analysis, and subject answering. The following are some typical application examples.
Here are more typical examples: 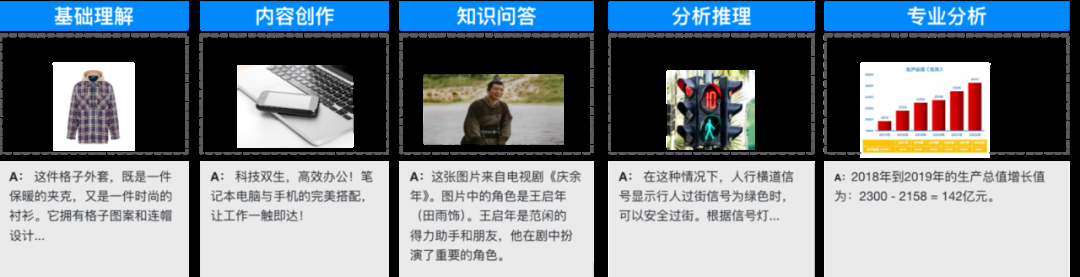
Convert an image into a text table:
Explain a piece of code: 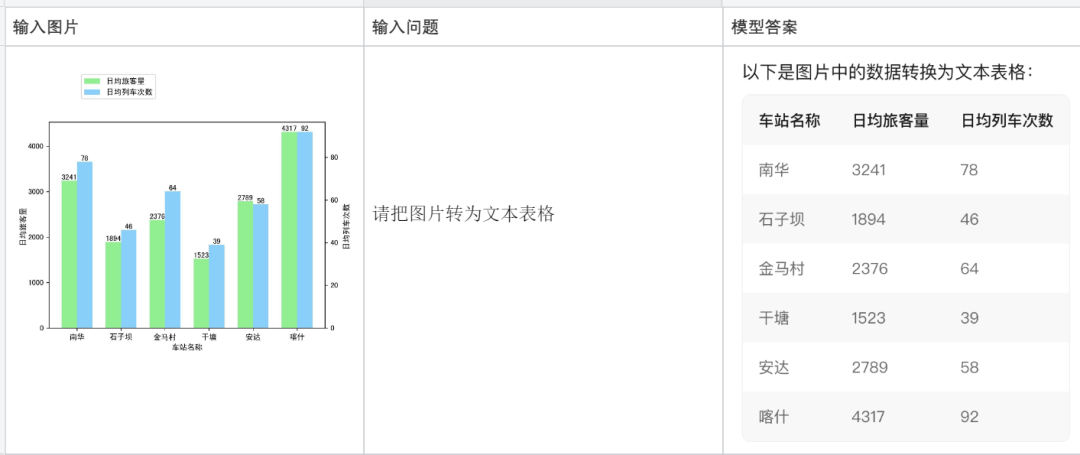
Analyze a bill: 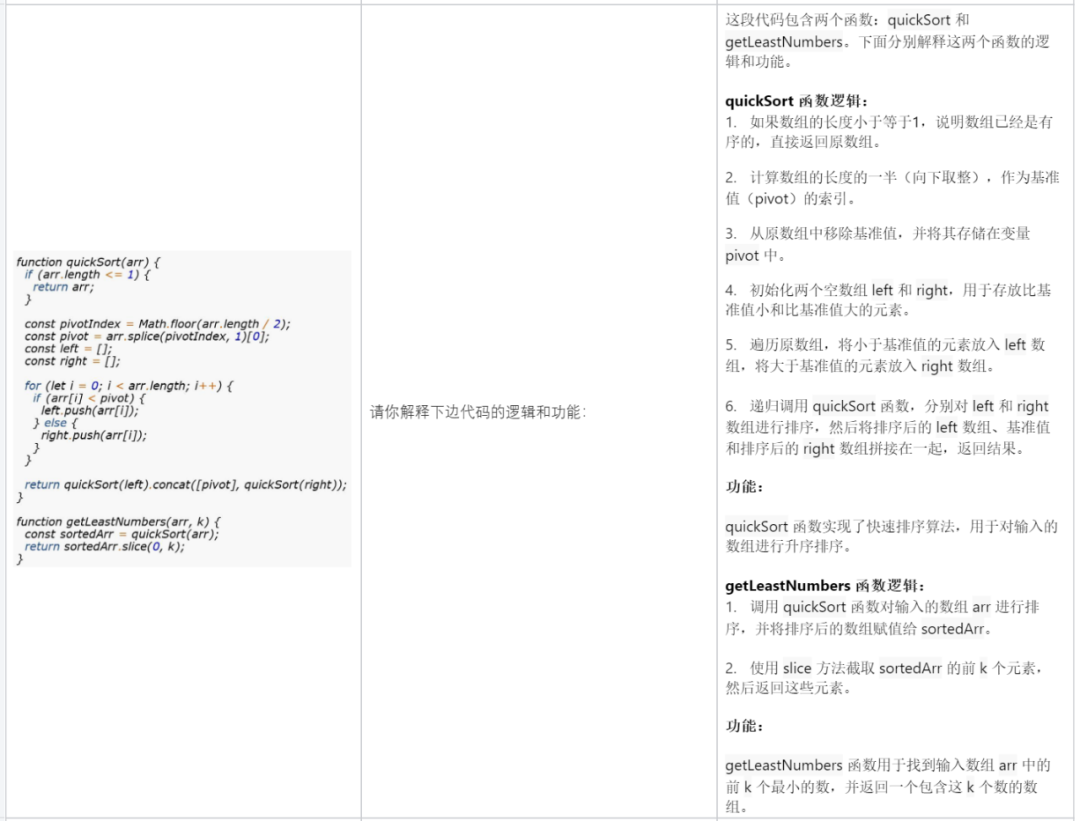
Description Picture content: 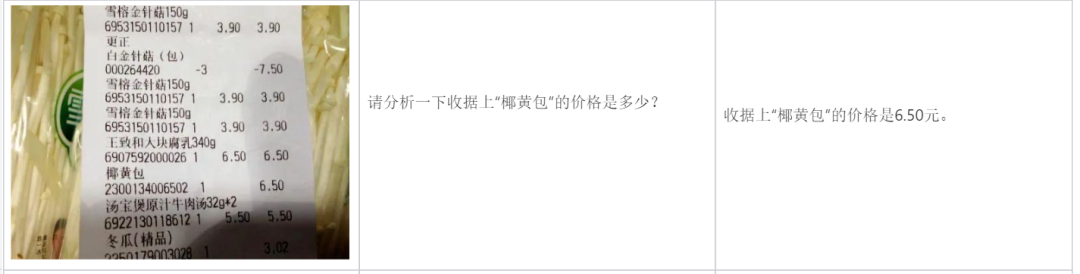
Do math problems: 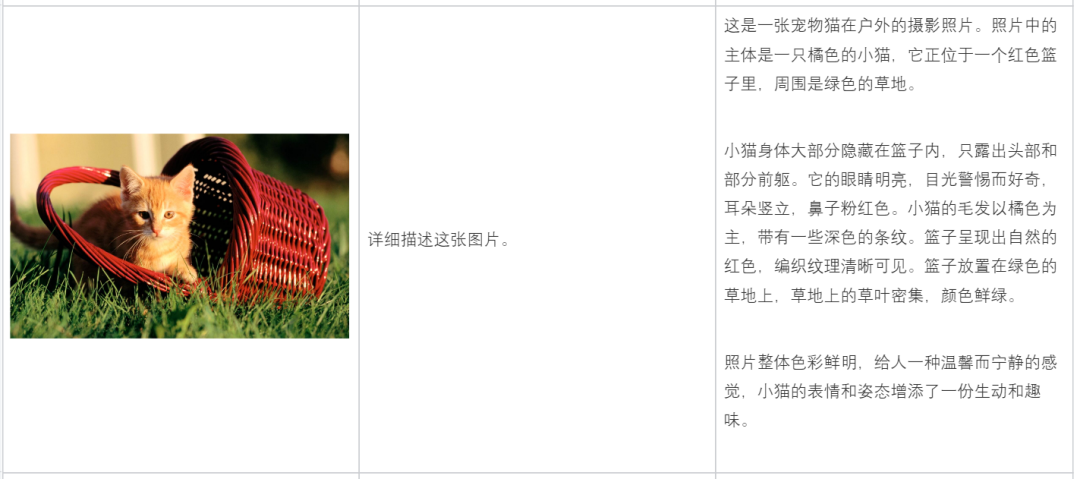
Analyze based on the content of the picture: 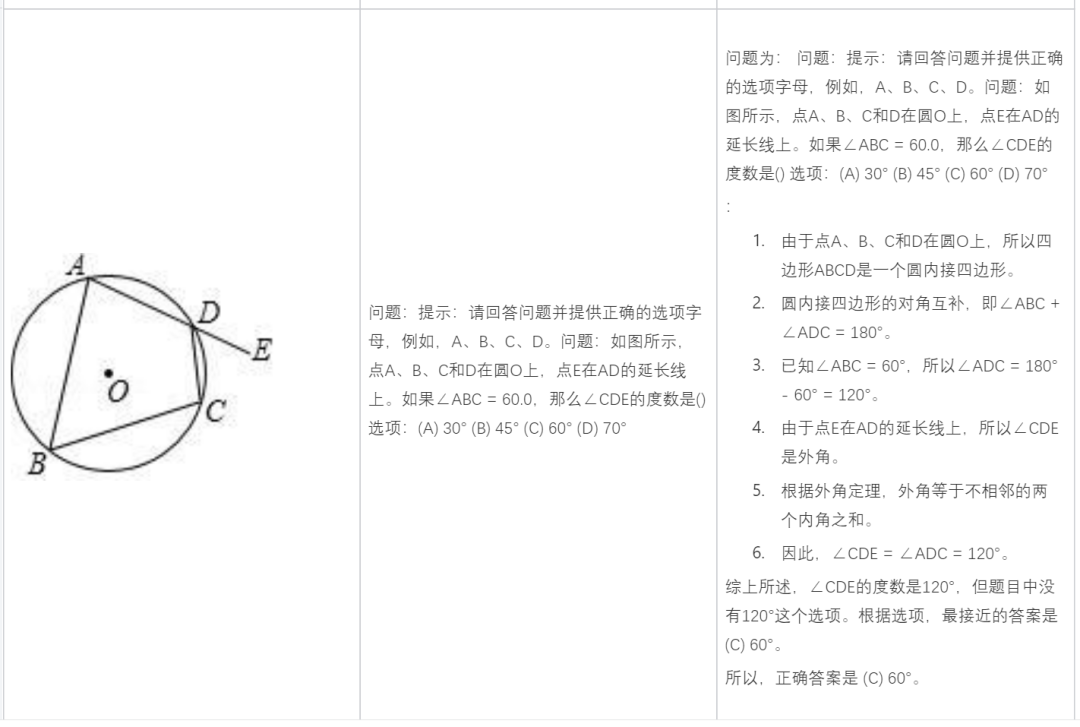
Help you write copy: 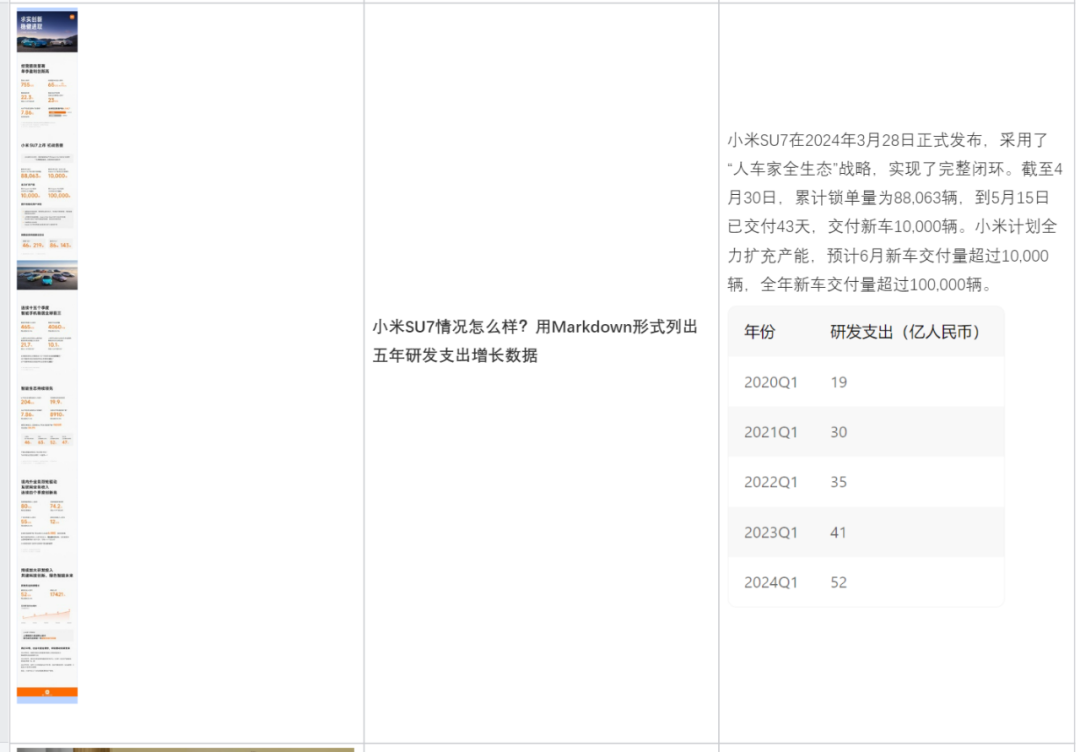
現在、Tencent の Hunyuan マルチモーダル理解大規模モデルは、AI アシスタント製品である Tencent Yuanbao でリリースされており、Tencent Cloud を通じて企業と個人の開発者に公開されています。
テンセント元宝アドレス: https://yuanbao.tencent.com/chat
The above is the detailed content of China's first self-developed MoE multi-modal large model reveals Tencent's mixed-element multi-modal understanding. For more information, please follow other related articles on the PHP Chinese website!