How to build a Hybrid Search System for RAG?
Building a hybrid search system for Retrieval-Augmented Generation (RAG) can greatly improve your search capabilities by combining traditional search techniques with advanced AI models. This system lets you retrieve the most relevant information from large datasets, improving accuracy and user experience. This guide will walk you through the essential steps to create a hybrid search system for RAG using simple language and clear steps.
Understanding RAG and Hybrid Search
RAG (Retrieval-Augmented Generation) combines information retrieval with AI-driven generation to answer questions or generate content. Hybrid Search Systems blend keyword-based and semantic searches, improving search results by considering literal text and deeper meaning.
Steps to Build a Hybrid Search System for RAG
1. Define Your Use Case
Define what you want to achieve, such as improving search results on your website or customer support system.
2. Choose Your Search Technologies
For a hybrid search system, you must pick technologies that handle both traditional and semantic searches.
- Keyword Search: Use engines like Elasticsearch or Solr. They are fast and great for matching exact terms.
- Semantic Search: Use AI models such as BERT, GPT, or any other transformer models that understand context and meaning beyond words.
3. Set Up Your Data Pipeline
You need a pipeline that feeds data into both search systems.
- Data Ingestion: Collect and clean data from websites, databases, or documents.
- Preprocessing: Standardize your data by removing duplicates, correcting errors, and ensuring consistency.
4. Implement the Keyword Search Layer
Set up 'Elasticsearch' or 'Solr' to handle exact keyword matches. This layer quickly finds documents that contain relevant terms.
- Indexing: Load your cleaned data into the search engine.
- Tuning: Adjust parameters like relevancy scores and query filters to refine the search results.
5. Integrate the Semantic Search Layer
Add a semantic search layer to handle context-aware queries.
- Model Selection: You can choose a pre-trained AI model like BERT or GPT or fine-tune your model to match your specific data.
- Query Processing: Use the AI model to understand user queries better and retrieve contextually relevant information.
6. Combine Results from Both Searches
Merge the results from the keyword and semantic searches. This blending ensures you get precise matches while capturing relevant content that may not have exact keyword overlaps.
- Scoring Mechanism: Develop a scoring method to rank results based on relevance from both systems.
- Ranking: Use a combination of scores to display the most relevant results first.
- Deploy and Test Your System Deploy your hybrid search system and run extensive tests to ensure it meets your performance and accuracy goals.
- Performance Testing: Check how quickly your system retrieves and ranks results.
- Accuracy Testing: Evaluate the relevance of the results to ensure they meet user expectations.
8. Monitor and Optimize
Regularly monitor the system's performance and make adjustments as needed.
- Feedback Loop: Collect user feedback to improve the system continuously.
- Model Updates: Update AI models to keep up with new data and maintain accuracy.
Conclusion
Building a hybrid search system for RAG involves combining the speed of keyword searches with the context-aware capabilities of AI models like BERT. By integrating these technologies, you can create a powerful search tool that delivers highly relevant results, enhancing user experience and system efficiency.
As an experienced developer with over ten years in the industry, I specialize in building complex systems like hybrid search engines tailored for RAG. My expertise in integrating traditional search technologies with advanced AI models ensures a scalable, accurate, high-performing solution. If you're looking to build or optimize a hybrid search system, feel free to connect—I can help manage and develop a robust solution that meets your needs.
The above is the detailed content of How to build a Hybrid Search System for RAG?. For more information, please follow other related articles on the PHP Chinese website!

Hot AI Tools

Undresser.AI Undress
AI-powered app for creating realistic nude photos

AI Clothes Remover
Online AI tool for removing clothes from photos.

Undress AI Tool
Undress images for free

Clothoff.io
AI clothes remover

Video Face Swap
Swap faces in any video effortlessly with our completely free AI face swap tool!

Hot Article

Hot Tools

Notepad++7.3.1
Easy-to-use and free code editor

SublimeText3 Chinese version
Chinese version, very easy to use

Zend Studio 13.0.1
Powerful PHP integrated development environment

Dreamweaver CS6
Visual web development tools

SublimeText3 Mac version
God-level code editing software (SublimeText3)

Hot Topics










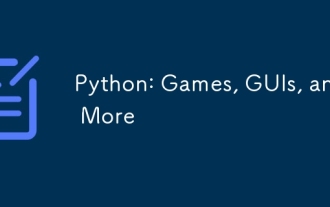
Python excels in gaming and GUI development. 1) Game development uses Pygame, providing drawing, audio and other functions, which are suitable for creating 2D games. 2) GUI development can choose Tkinter or PyQt. Tkinter is simple and easy to use, PyQt has rich functions and is suitable for professional development.
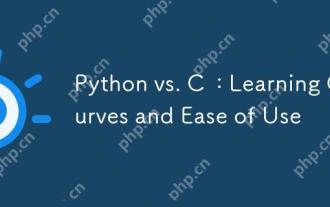
Python is easier to learn and use, while C is more powerful but complex. 1. Python syntax is concise and suitable for beginners. Dynamic typing and automatic memory management make it easy to use, but may cause runtime errors. 2.C provides low-level control and advanced features, suitable for high-performance applications, but has a high learning threshold and requires manual memory and type safety management.
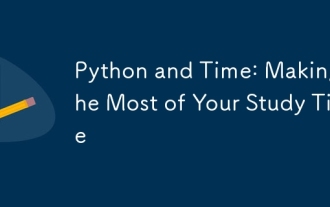
To maximize the efficiency of learning Python in a limited time, you can use Python's datetime, time, and schedule modules. 1. The datetime module is used to record and plan learning time. 2. The time module helps to set study and rest time. 3. The schedule module automatically arranges weekly learning tasks.
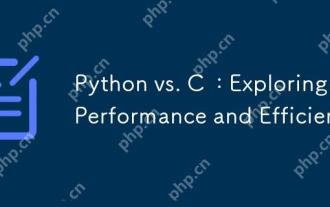
Python is better than C in development efficiency, but C is higher in execution performance. 1. Python's concise syntax and rich libraries improve development efficiency. 2.C's compilation-type characteristics and hardware control improve execution performance. When making a choice, you need to weigh the development speed and execution efficiency based on project needs.
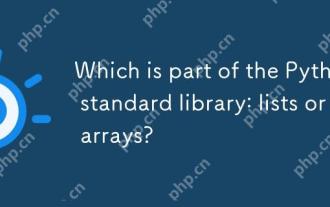
Pythonlistsarepartofthestandardlibrary,whilearraysarenot.Listsarebuilt-in,versatile,andusedforstoringcollections,whereasarraysareprovidedbythearraymoduleandlesscommonlyusedduetolimitedfunctionality.
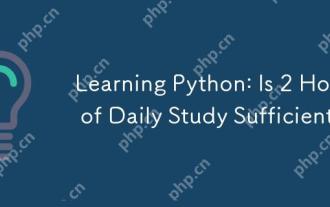
Is it enough to learn Python for two hours a day? It depends on your goals and learning methods. 1) Develop a clear learning plan, 2) Select appropriate learning resources and methods, 3) Practice and review and consolidate hands-on practice and review and consolidate, and you can gradually master the basic knowledge and advanced functions of Python during this period.
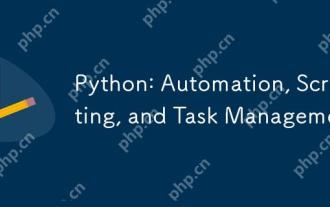
Python excels in automation, scripting, and task management. 1) Automation: File backup is realized through standard libraries such as os and shutil. 2) Script writing: Use the psutil library to monitor system resources. 3) Task management: Use the schedule library to schedule tasks. Python's ease of use and rich library support makes it the preferred tool in these areas.
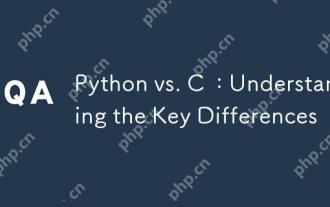
Python and C each have their own advantages, and the choice should be based on project requirements. 1) Python is suitable for rapid development and data processing due to its concise syntax and dynamic typing. 2)C is suitable for high performance and system programming due to its static typing and manual memory management.
