How to Build a Basic Chatbot Using TensorFlow and JavaScript
We all come across chatbots when visiting various sites, while some of them operate behind real-human interaction, others are powered by AI.
In this article, we'll walk through building a simple AI-powered chatbot using TensorFlow and JavaScript. The chatbot will recognize user commands and respond with predefined answers.
Step-by-Step Guide
Setting Up Our Project
First, we create a new directory for our project and initialize it with npm, ensure you have Node.js installed on your system before starting this step.
mkdir chatbot cd chatbot npm init -y
Install necessary packages
We would be using the following npm packages for our simple project:
- tensorflow/tfjs: TensorFlow.js library for machine learning.
- tensorflow-models/universal-sentence-encoder: Pre-trained Universal Sentence Encoder model for intent recognition.
npm install @tensorflow/tfjs @tensorflow-models/universal sentence-encoder
-
Create intents
Create a file named intents.js to store intents/commands, These are categories of user inputs that the chatbot will recognize (e.g., greetings, product inquiries, order status).
// intents.js const intents = { greeting: ["hello", "hi", "hey", "good morning", "good evening", "howdy"], goodbye: ["bye", "goodbye", "see you later", "farewell", "catch you later"], thanks: ["thank you", "thanks", "much appreciated", "thank you very much"], product_inquiry: ["tell me about your products", "what do you sell?", "product information", "what can I buy?", "show me your products"], order_status: ["where is my order?", "order status", "track my order", "order tracking", "order update"], shipping_info: ["shipping information", "how do you ship?", "shipping methods", "delivery options", "how long does shipping take?"], return_policy: ["return policy", "how to return?", "return process", "can I return?", "returns"], payment_methods: ["payment options", "how can I pay?", "payment methods", "available payments"], support_contact: ["contact support", "how to contact support?", "customer support contact", "support info", "customer service contact"], business_hours: ["business hours", "working hours", "when are you open?", "opening hours", "store hours"] }; module.exports = { intents }
Copy after login -
Create responses
Create another file named responses.js to store predefined responses, These are the predefined responses the chatbot will give based on the recognized intent.
// responses.js const responses = { greeting: "Hello! How can I help you today?", goodbye: "Goodbye! Have a great day!", thanks: "You're welcome! If you have any other questions, feel free to ask.", product_inquiry: "We offer a variety of products including electronics, books, clothing, and more. How can I assist you further?", order_status: "Please provide your order ID, and I will check the status for you.", shipping_info: "We offer various shipping methods including standard, express, and next-day delivery. Shipping times depend on the method chosen and your location.", return_policy: "Our return policy allows you to return products within 30 days of purchase. Please visit our returns page for detailed instructions.", payment_methods: "We accept multiple payment methods including credit/debit cards, PayPal, and bank transfers. Please choose the method that suits you best at checkout.", support_contact: "You can contact our support team via email at support@example.com or call us at 1-800-123-4567.", business_hours: "Our business hours are Monday to Friday, 9 AM to 5 PM. We are closed on weekends and public holidays." }; module.exports = { responses };
Copy after login -
Loading TensorFlow and the Sentence Encoder
Create a main script file named chatbot.js and load the necessary libraries and models, we load the universal sentence encoder model asynchronously and start the chatbot once the model is loaded.
// chatbot.js const tf = require('@tensorflow/tfjs'); const use = require('@tensorflow-models/universal-sentence-encoder'); const { intents } = require('./intents'); const { responses } = require('./responses'); const readline = require('readline'); // Load the Universal Sentence Encoder model let model; use.load().then((loadedModel) => { model = loadedModel; console.log("Model loaded"); startChatbot(); });
Copy after login -
Implementing Intent Recognition
Add a function to recognize the intent of the user's input, we embed the user input into a high-dimensional vector using the universal encoder and then track the highest similarity score based on the intent.
async function recognizeIntent(userInput) { const userInputEmb = await model.embed([userInput]); let maxScore = -1; let recognizedIntent = null; for (const [intent, examples] of Object.entries(intents)) { // Embedding the example phrases for each intent & Calculating similarity scores between the user input embedding and the example embeddings const examplesEmb = await model.embed(examples); const scores = await tf.matMul(userInputEmb, examplesEmb, false, true).data(); const maxExampleScore = Math.max(...scores); if (maxExampleScore > maxScore) { maxScore = maxExampleScore; recognizedIntent = intent; } } return recognizedIntent; }
Copy after login -
Generating Responses
Add a function to generate responses based on the recognized intent:
async function generateResponse(userInput) { const intent = await recognizeIntent(userInput); if (intent && responses[intent]) { return responses[intent]; } else { return "I'm sorry, I don't understand that. Can you please rephrase?"; } }
Copy after login -
Implementing Chatbot Interaction
Finally, implement the interaction loop with the chatbot by setting up the interface for reading user input from the command line, prompting the user for input and generating responses accordingly:
function startChatbot() { const rl = readline.createInterface({ input: process.stdin, output: process.stdout }); console.log("Welcome to the customer service chatbot! Type 'quit' to exit."); rl.prompt(); rl.on('line', async (line) => { const userInput = line.trim(); if (userInput.toLowerCase() === 'quit') { console.log("Chatbot: Goodbye!"); rl.close(); return; } const response = await generateResponse(userInput); console.log(`Chatbot: ${response}`); rl.prompt(); }); }
Copy after loginHere is the completed code for chatbot.js :
// chatbot.js const tf = require('@tensorflow/tfjs'); const use = require('@tensorflow-models/universal-sentence-encoder'); const { intents } = require('./intents'); const { responses } = require('./responses'); const readline = require('readline'); // Load the Universal Sentence Encoder model let model; use.load().then((loadedModel) => { model = loadedModel; console.log("Model loaded"); startChatbot(); }); async function recognizeIntent(userInput) { const userInputEmb = await model.embed([userInput]); let maxScore = -1; let recognizedIntent = null; for (const [intent, examples] of Object.entries(intents)) { const examplesEmb = await model.embed(examples); const scores = await tf.matMul(userInputEmb, examplesEmb, false, true).data(); const maxExampleScore = Math.max(...scores); if (maxExampleScore > maxScore) { maxScore = maxExampleScore; recognizedIntent = intent; } } return recognizedIntent; } async function generateResponse(userInput) { const intent = await recognizeIntent(userInput); if (intent && responses[intent]) { return responses[intent]; } else { return "I'm sorry, I don't understand that. Can you please rephrase?"; } } function startChatbot() { const rl = readline.createInterface({ input: process.stdin, output: process.stdout }); console.log("Welcome to the customer service chatbot! Type 'quit' to exit."); rl.prompt(); rl.on('line', async (line) => { const userInput = line.trim(); if (userInput.toLowerCase() === 'quit') { console.log("Chatbot: Goodbye!"); rl.close(); return; } const response = await generateResponse(userInput); console.log(`Chatbot: ${response}`); rl.prompt(); }); }
Copy after login -
To run the chatbot, execute the chatbot.js file:
node chatbot.js
Copy after login
Voila! Our command output should have the chatbot running:
Conclusion
In this article, we've built a simple customer service chatbot using TensorFlow and JavaScript. While this implementation is basic, it provides a solid foundation for building more sophisticated chatbots. You can expand this project by integrating APIs using AXIOS, adding more intents and responses, or deploying it on a web platform.
Happy coding!
?? Learn more about me
?? Connect on LinkedIn
?? Subscribe to my blog
The above is the detailed content of How to Build a Basic Chatbot Using TensorFlow and JavaScript. For more information, please follow other related articles on the PHP Chinese website!

Hot AI Tools

Undresser.AI Undress
AI-powered app for creating realistic nude photos

AI Clothes Remover
Online AI tool for removing clothes from photos.

Undress AI Tool
Undress images for free

Clothoff.io
AI clothes remover

Video Face Swap
Swap faces in any video effortlessly with our completely free AI face swap tool!

Hot Article

Hot Tools

Notepad++7.3.1
Easy-to-use and free code editor

SublimeText3 Chinese version
Chinese version, very easy to use

Zend Studio 13.0.1
Powerful PHP integrated development environment

Dreamweaver CS6
Visual web development tools

SublimeText3 Mac version
God-level code editing software (SublimeText3)

Hot Topics










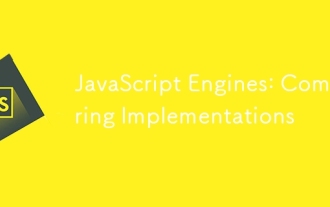
Different JavaScript engines have different effects when parsing and executing JavaScript code, because the implementation principles and optimization strategies of each engine differ. 1. Lexical analysis: convert source code into lexical unit. 2. Grammar analysis: Generate an abstract syntax tree. 3. Optimization and compilation: Generate machine code through the JIT compiler. 4. Execute: Run the machine code. V8 engine optimizes through instant compilation and hidden class, SpiderMonkey uses a type inference system, resulting in different performance performance on the same code.
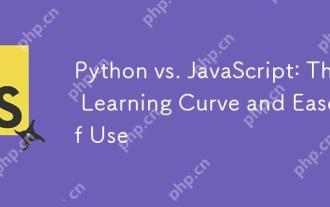
Python is more suitable for beginners, with a smooth learning curve and concise syntax; JavaScript is suitable for front-end development, with a steep learning curve and flexible syntax. 1. Python syntax is intuitive and suitable for data science and back-end development. 2. JavaScript is flexible and widely used in front-end and server-side programming.
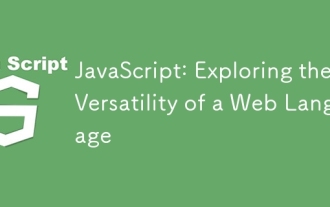
JavaScript is the core language of modern web development and is widely used for its diversity and flexibility. 1) Front-end development: build dynamic web pages and single-page applications through DOM operations and modern frameworks (such as React, Vue.js, Angular). 2) Server-side development: Node.js uses a non-blocking I/O model to handle high concurrency and real-time applications. 3) Mobile and desktop application development: cross-platform development is realized through ReactNative and Electron to improve development efficiency.
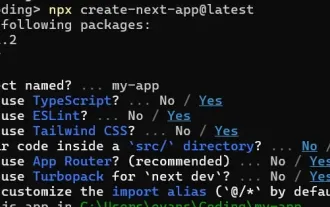
This article demonstrates frontend integration with a backend secured by Permit, building a functional EdTech SaaS application using Next.js. The frontend fetches user permissions to control UI visibility and ensures API requests adhere to role-base
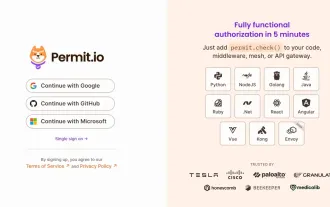
I built a functional multi-tenant SaaS application (an EdTech app) with your everyday tech tool and you can do the same. First, what’s a multi-tenant SaaS application? Multi-tenant SaaS applications let you serve multiple customers from a sing
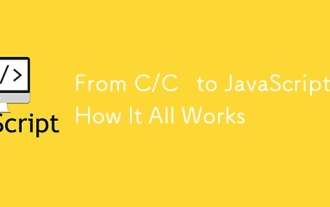
The shift from C/C to JavaScript requires adapting to dynamic typing, garbage collection and asynchronous programming. 1) C/C is a statically typed language that requires manual memory management, while JavaScript is dynamically typed and garbage collection is automatically processed. 2) C/C needs to be compiled into machine code, while JavaScript is an interpreted language. 3) JavaScript introduces concepts such as closures, prototype chains and Promise, which enhances flexibility and asynchronous programming capabilities.
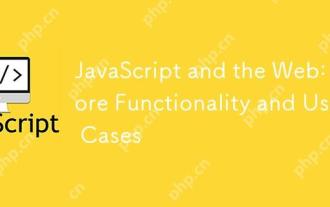
The main uses of JavaScript in web development include client interaction, form verification and asynchronous communication. 1) Dynamic content update and user interaction through DOM operations; 2) Client verification is carried out before the user submits data to improve the user experience; 3) Refreshless communication with the server is achieved through AJAX technology.
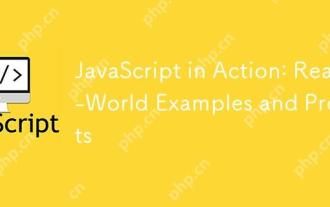
JavaScript's application in the real world includes front-end and back-end development. 1) Display front-end applications by building a TODO list application, involving DOM operations and event processing. 2) Build RESTfulAPI through Node.js and Express to demonstrate back-end applications.
