Creating a Multi-Agent System in AI
Multi-agent systems (MAS) are transforming how businesses approach complex problem-solving in AI. As tech evolves, companies seek more sophisticated solutions for managing decentralized, dynamic, and collaborative environments. This guide is tailored for you, offering insights into building MAS, their applications, and how they differ from Retrieval-Augmented Generation (RAG) models.
What is a Multi-Agent System (MAS)?
A Multi-Agent System (MAS) is a framework where multiple intelligent agents interact and work together to solve problems. These agents can be software entities, robots, or other autonomous systems. Each agent in MAS has specific goals, knowledge, and capabilities, allowing it to make decisions and communicate with other agents to achieve collective objectives.
Key Characteristics:
- Autonomy: Agents operate independently without direct intervention.
- Social Ability: Agents interact and collaborate to solve problems.
- Reactivity: Agents perceive their environment and respond accordingly.
- Proactiveness: Agents take the initiative to achieve goals.
Applications of MAS:
- Supply Chain Management: Automating procurement, inventory management, and logistics.
- Smart Grids: Managing energy distribution with dynamic demand and supply.
- Financial Trading: Automated trading systems making market decisions based on real-time data.
- Healthcare: Managing patient data, diagnostics, and treatment recommendations.
Creating a Multi-Agent System: Key Steps
- Define the Problem and Goals: Start by identifying the problem you want to solve and outlining the desired outcomes, such as optimizing logistics in supply chain management.
- Design the Agents: Define each agent's roles, capabilities, and goals. Ensure they can operate autonomously and communicate effectively with other agents. To streamline this process, use frameworks like JADE (Java Agent Development Framework) or Python-based platforms like SPADE (Smart Python Agent Development Environment).
Example: Defining a Simple Agent in Python using SPADE
- Establish Communication Protocols: Agents need to exchange information reliably. Use standardized protocols like FIPA (Foundation for Intelligent Physical Agents) for smooth inter-agent communication.
Example: Sending a Message between Agents
- Develop Decision-Making Algorithms: Incorporate decision-making logic into your agents, such as rule-based systems, machine learning models, or reinforcement learning for adaptability.
Example: Simple Rule-Based Decision
- Test and Validate: Run simulations to test the agents' behavior in different scenarios. Validate their performance against the defined goals and make adjustments as needed.
- Deploy and Monitor: Once tested, deploy your MAS in a real-world environment. Continuously monitor the system to ensure agents adapt to changing conditions and improve their performance over time.
MAS vs. RAG: Understanding the Differences
While MAS focuses on collaborative problem-solving, Retrieval-Augmented Generation (RAG) models are specialized AI systems for information retrieval and generation.
Multi-Agent System (MAS):
- Focus: Collaborative problem-solving using multiple intelligent agents.
- Approach: Decentralized; agents work independently and interact with one another.
- Applications: Supply chain optimization, smart grids, autonomous vehicles, etc.
- Decision-Making: Each agent makes decisions based on local information and coordination with others.
Retrieval-Augmented Generation (RAG):
- Focus: Enhancing AI models (like chatbots) with real-time information retrieval to generate responses.
- Approach: Centralized; a single model uses retrieved data to improve outputs.
- Applications: Customer support, information retrieval systems, content generation.
- Decision Making: Relies on retrieval mechanisms to fetch relevant information before generating a response.
Example: Implementing a RAG Model
Why MAS is the Future for Complex Systems?
MAS offers a robust solution for environments that require distributed control and decision-making. It enhances efficiency, scalability, and adaptability—key factors for tech startups and enterprises aiming to innovate.
- Enhanced Scalability: Each agent can be scaled independently, making the system highly adaptable.
- Decentralized Control: No single point of failure, enhancing reliability and resilience.
- Improved Collaboration: Agents work in sync, handling tasks too complex for a single system.
Conclusion
Building a Multi-Agent System requires careful planning, design, and execution. However, the benefits—especially in complex, dynamic environments—are significant. Whether you're leading a development team or managing operations, MAS offers a pathway to more efficient, scalable, and intelligent systems that can keep pace with the evolving demands of modern business.
Understanding and leveraging MAS can be a game-changer for tech leaders, driving innovation and unlocking new performance levels. If you're exploring implementing MAS in your operations, now is the time to transform your problem-solving approach.
Ready to explore how a Multi-Agent System can transform your operations? Contact me today to discuss how I can help you design and implement a MAS customized to your needs and goals.
The above is the detailed content of Creating a Multi-Agent System in AI. For more information, please follow other related articles on the PHP Chinese website!

Hot AI Tools

Undresser.AI Undress
AI-powered app for creating realistic nude photos

AI Clothes Remover
Online AI tool for removing clothes from photos.

Undress AI Tool
Undress images for free

Clothoff.io
AI clothes remover

Video Face Swap
Swap faces in any video effortlessly with our completely free AI face swap tool!

Hot Article

Hot Tools

Notepad++7.3.1
Easy-to-use and free code editor

SublimeText3 Chinese version
Chinese version, very easy to use

Zend Studio 13.0.1
Powerful PHP integrated development environment

Dreamweaver CS6
Visual web development tools

SublimeText3 Mac version
God-level code editing software (SublimeText3)

Hot Topics










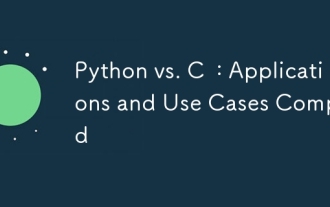
Python is suitable for data science, web development and automation tasks, while C is suitable for system programming, game development and embedded systems. Python is known for its simplicity and powerful ecosystem, while C is known for its high performance and underlying control capabilities.
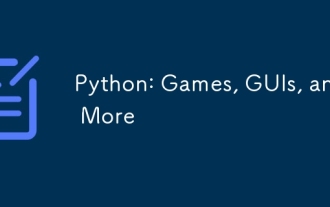
Python excels in gaming and GUI development. 1) Game development uses Pygame, providing drawing, audio and other functions, which are suitable for creating 2D games. 2) GUI development can choose Tkinter or PyQt. Tkinter is simple and easy to use, PyQt has rich functions and is suitable for professional development.
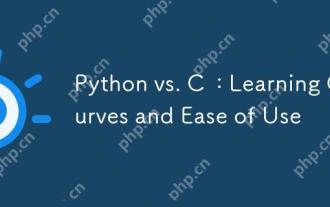
Python is easier to learn and use, while C is more powerful but complex. 1. Python syntax is concise and suitable for beginners. Dynamic typing and automatic memory management make it easy to use, but may cause runtime errors. 2.C provides low-level control and advanced features, suitable for high-performance applications, but has a high learning threshold and requires manual memory and type safety management.
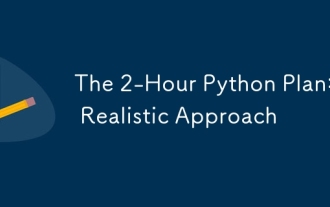
You can learn basic programming concepts and skills of Python within 2 hours. 1. Learn variables and data types, 2. Master control flow (conditional statements and loops), 3. Understand the definition and use of functions, 4. Quickly get started with Python programming through simple examples and code snippets.
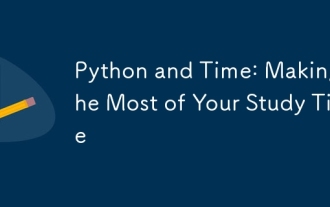
To maximize the efficiency of learning Python in a limited time, you can use Python's datetime, time, and schedule modules. 1. The datetime module is used to record and plan learning time. 2. The time module helps to set study and rest time. 3. The schedule module automatically arranges weekly learning tasks.
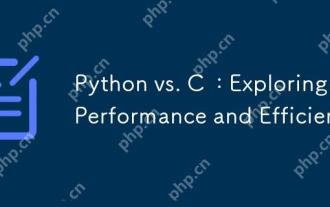
Python is better than C in development efficiency, but C is higher in execution performance. 1. Python's concise syntax and rich libraries improve development efficiency. 2.C's compilation-type characteristics and hardware control improve execution performance. When making a choice, you need to weigh the development speed and execution efficiency based on project needs.
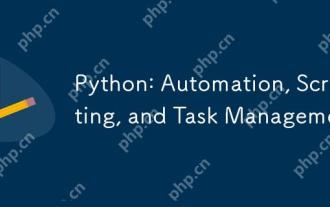
Python excels in automation, scripting, and task management. 1) Automation: File backup is realized through standard libraries such as os and shutil. 2) Script writing: Use the psutil library to monitor system resources. 3) Task management: Use the schedule library to schedule tasks. Python's ease of use and rich library support makes it the preferred tool in these areas.
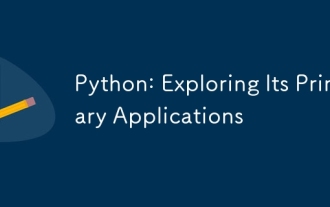
Python is widely used in the fields of web development, data science, machine learning, automation and scripting. 1) In web development, Django and Flask frameworks simplify the development process. 2) In the fields of data science and machine learning, NumPy, Pandas, Scikit-learn and TensorFlow libraries provide strong support. 3) In terms of automation and scripting, Python is suitable for tasks such as automated testing and system management.
