


What-if I told you complex Data Integration makes good Task Orchestration?
No matter how complex your data problem is, you can always Divide & Conquer it. ???
In this article, we’ll break down how to solve intricate data challenges, data treatment, data management using a fully open-source Python library: Taipy. Whether you're cleaning data, orchestrating tasks, or exploring different outcomes, Taipy's intuitive design empowers you to handle it all.
Star Taipy ⭐️
Taipy's Scenario Management basis
Let’s go through three steps to show how Taipy can help streamline your data workflow:
1. Data Integration: Get the Data You Need
Every AI, ML, and data-driven project starts with data, obviously!
And it’s rarely as simple as a single, clean dataset. In most cases, you'll be pulling data from databases, APIs, flat files, or other external sources, and sometimes, all in the same project. This is where Taipy's data integration comes in—gathering and unifying data from these different sources.
In Taipy, this process is simplified with a key abstraction: the Data Node.
A Data Node represents your data but doesn’t store it directly. Instead, it holds all the necessary metadata to read and write the actual data, whether it’s a CSV file, a database table, or even an API response.
Here’s a quick example of defining a Data Node for a CSV:
from taipy import Config initial_dataset_cfg = Config.configure_data_node(id="initial_dataset", storage_type="csv", path="data/dataset.csv", scope=Scope.GLOBAL)
With this abstraction, Taipy takes care of data management, allowing you to focus on transforming and processing your data.
2. Task Orchestration: What Are You Doing with All That Data?
Now that your data is in place, what do you do with it? In any data workflow, the next step involves defining tasks that process and transform the data. This is what we call the Task Orchestration
A Task in Taipy is like a function, taking Data Node(s) as inputs, performing transformations, and then outputting Data Node(s).
For instance, you may want to filter some data or calculate new metrics. Here's an example of creating a Task to calculate the sum of a column:
clean_data_task_cfg = Config.configure_task(id="clean_data", function=clean_data, input=initial_dataset_cfg, output=cleaned_dataset_cfg, skippable=True)
Once you've defined your tasks, you can arrange them into a pipeline to ensure the steps execute in the correct order. This lets you easily build workflows that transform data, build models, generate reports, and more.
3. What-If Analysis: Explore Different Scenarios
Once your data and workflows are set up, you can begin exploring different scenarios. This is where the What-if analysis comes into play.
A Scenario in Taipy represents a specific instance of a problem you’re trying to solve, allowing you to test different parameters and see how they impact your results. By adjusting input data or assumptions, you can model various scenarios without starting over from scratch.
Here's how you can define a Scenario in Taipy:
scenario_cfg = Config.configure_scenario(id="scenario", task_configs=[clean_data_task_cfg, predict_task_cfg,evaluate_task_cfg], frequency=Frequency.MONTHLY) tp.Core().run() my_first_scenario = create_and_run_scenario(dt.datetime(2021, 1, 25)) predictions = my_first_scenario.predictions.read() print("Predictions\n", predictions)
This makes it easy to perform sensitivity analysis or optimize outcomes, all within the same framework. Want to test different discount rates on your sales model? Just create new scenarios, tweak the parameters, and rerun them.
Taipy vs. Other Pipeline Management Tools
You may be wondering, how does Taipy compare to other popular pipeline orchestration tools like Apache Airflow, Luigi, or Prefect? While those tools are great for managing task scheduling across distributed environments, Taipy stands out by focusing on Python simplicity, especially when it comes to scenario management and what-if analysis.
Airflow/Luigi/Prefect: Generally focus on orchestrating ETL processes, scheduling, and monitoring workflows.
Taipy: It not only provides workflow orchestration but also simplifies what-if analysis with its unique scenario abstraction, allowing you to model various outcomes seamlessly.
For developers looking to handle complex data workflows in Python with minimal setup, Taipy offers a more straightforward, code-first approach.
Conclusion: Divide and Conquer with Taipy
The Divide and Conquer strategy wins every time, no matter the size or complexity of your data problems! With Taipy, you can handle everything from data integration to task orchestration and what-if analysis, all in one place. And you can finalize with data visualization as well.
Ready to give Taipy a try? Check out the GitHub repo and see how it can streamline your data workflows today!
Star Taipy ⭐️
Don’t forget to leave a star ⭐ and share your feedback or scenarios you've worked on in the comments below!
The above is the detailed content of What-if I told you complex Data Integration makes good Task Orchestration?. For more information, please follow other related articles on the PHP Chinese website!

Hot AI Tools

Undresser.AI Undress
AI-powered app for creating realistic nude photos

AI Clothes Remover
Online AI tool for removing clothes from photos.

Undress AI Tool
Undress images for free

Clothoff.io
AI clothes remover

Video Face Swap
Swap faces in any video effortlessly with our completely free AI face swap tool!

Hot Article

Hot Tools

Notepad++7.3.1
Easy-to-use and free code editor

SublimeText3 Chinese version
Chinese version, very easy to use

Zend Studio 13.0.1
Powerful PHP integrated development environment

Dreamweaver CS6
Visual web development tools

SublimeText3 Mac version
God-level code editing software (SublimeText3)

Hot Topics










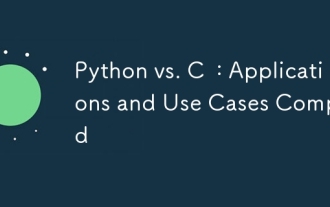
Python is suitable for data science, web development and automation tasks, while C is suitable for system programming, game development and embedded systems. Python is known for its simplicity and powerful ecosystem, while C is known for its high performance and underlying control capabilities.
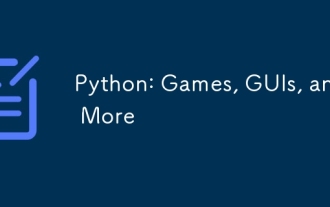
Python excels in gaming and GUI development. 1) Game development uses Pygame, providing drawing, audio and other functions, which are suitable for creating 2D games. 2) GUI development can choose Tkinter or PyQt. Tkinter is simple and easy to use, PyQt has rich functions and is suitable for professional development.
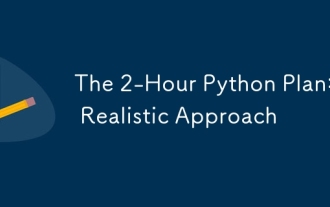
You can learn basic programming concepts and skills of Python within 2 hours. 1. Learn variables and data types, 2. Master control flow (conditional statements and loops), 3. Understand the definition and use of functions, 4. Quickly get started with Python programming through simple examples and code snippets.
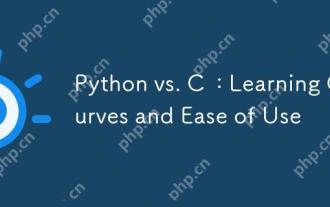
Python is easier to learn and use, while C is more powerful but complex. 1. Python syntax is concise and suitable for beginners. Dynamic typing and automatic memory management make it easy to use, but may cause runtime errors. 2.C provides low-level control and advanced features, suitable for high-performance applications, but has a high learning threshold and requires manual memory and type safety management.
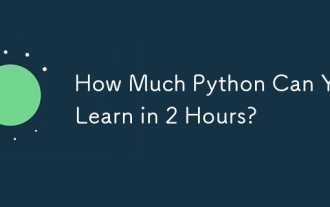
You can learn the basics of Python within two hours. 1. Learn variables and data types, 2. Master control structures such as if statements and loops, 3. Understand the definition and use of functions. These will help you start writing simple Python programs.
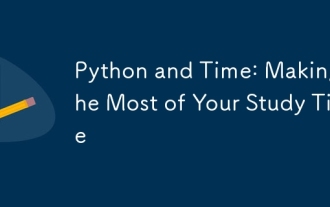
To maximize the efficiency of learning Python in a limited time, you can use Python's datetime, time, and schedule modules. 1. The datetime module is used to record and plan learning time. 2. The time module helps to set study and rest time. 3. The schedule module automatically arranges weekly learning tasks.
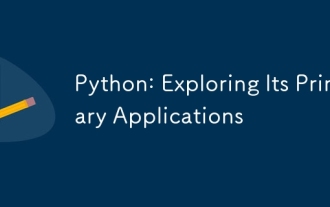
Python is widely used in the fields of web development, data science, machine learning, automation and scripting. 1) In web development, Django and Flask frameworks simplify the development process. 2) In the fields of data science and machine learning, NumPy, Pandas, Scikit-learn and TensorFlow libraries provide strong support. 3) In terms of automation and scripting, Python is suitable for tasks such as automated testing and system management.
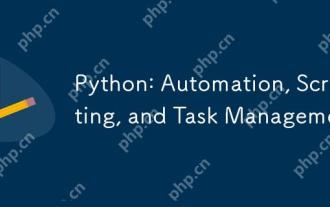
Python excels in automation, scripting, and task management. 1) Automation: File backup is realized through standard libraries such as os and shutil. 2) Script writing: Use the psutil library to monitor system resources. 3) Task management: Use the schedule library to schedule tasks. Python's ease of use and rich library support makes it the preferred tool in these areas.
