Kth Largest Element in an Array
#️⃣ Array, Priority Queue, Quick Select
https://leetcode.com/problems/kth-largest-element-in-an-array/description
? Understand Problem
If the array is [8, 6, 12, 9, 3, 4] and k is 2, you need to find the 2nd largest element in this array. First, you will sort the array: [3, 4, 6, 8, 9, 12] The output will be 9 because it is the second-largest element.
✅ Bruteforce
var findKthLargest = function(nums, k) { // Sort the array in ascending order nums.sort((a, b) => a - b); // Return the kth largest element return nums[nums.length - k]; };
Explanation:
- Sorting the Array: The array is sorted in ascending order using the sort method.
- Finding the kth Largest Element: The kth largest element is found by accessing the element at the index nums.length - k.
Time Complexity:
- Sorting: The time complexity of sorting an array is (O(nlog n)), where (n) is the length of the array.
- Accessing the Element: Accessing an element in an array is O(1).
So, the overall time complexity is O(n log n).
Space Complexity:
- In-Place Sorting: The sort method sorts the array in place, so the space complexity is O(1) for the sorting operation.
- Overall: Since we are not using any additional data structures, the overall space complexity is O(1).
✅ Better
var findKthLargest = function(nums, k) { // Create a min-heap using a priority queue let minHeap = new MinPriorityQueue(); // Add the first k elements to the heap for (let i = 0; i < k; i++) { //minHeap.enqueue(nums[i]): Adds the element nums[i] to the min-heap. minHeap.enqueue(nums[i]); } // Iterate through the remaining elements for (let i = k; i < nums.length; i++) { //minHeap.front().element: Retrieves the smallest element in the min-heap without removing it. if (minHeap.front().element < nums[i]) { // minHeap.dequeue(): Removes the smallest element from the min-heap. minHeap.dequeue(); // Add the current element minHeap.enqueue(nums[i]); } } // The root of the heap is the kth largest element return minHeap.front().element; };
Explanation:
- Initial Array: [2, 1, 6, 3, 5, 4]
- k = 3: We need to find the 3rd largest element.
Step 1: Add the first k elements to the min-heap
- Heap after adding 2: [2]
- Heap after adding 1: [1, 2]
- Heap after adding 6: [1, 2, 6]
Step 2: Iterate through the remaining elements
-
Current element = 3
- Heap before comparison: [1, 2, 6]
- Smallest element in heap (minHeap.front().element): 1
- Comparison: 3 > 1
- Action: Remove 1 and add 3
- Heap after update: [2, 6, 3]
-
Current element = 5
- Heap before comparison: [2, 6, 3]
- Smallest element in heap (minHeap.front().element): 2
- Comparison: 5 > 2
- Action: Remove 2 and add 5
- Heap after update: [3, 6, 5]
-
Current element = 4
- Heap before comparison: [3, 6, 5]
- Smallest element in heap (minHeap.front().element): 3
- Comparison: 4 > 3
- Action: Remove 3 and add 4
- Heap after update: [4, 6, 5]
- Heap: [4, 6, 5]
- 3rd largest element: 4 (the root of the heap)
- Heap Operations: Inserting and removing elements from the heap takes O(log k) time.
- Overall: Since we perform these operations for each of the n elements in the array, the overall time complexity is O(n log k).
- Heap Storage: The space complexity is O(k) because the heap stores at most k elements.
- Initial Array: [3, 2, 1, 5, 6, 4]
- k = 2: We need to find the 2nd largest element.
- Pivot element = 4
- Array after partitioning: [3, 2, 1, 4, 6, 5]
- Pivot index = 3
- Target index = 4 (since we need the 2nd largest element, which is the 4th index in 0-based indexing)
- Pivot index (3) < Target index (4): Search in the right partition [6, 5]
- Pivot element = 5
- Array after partitioning: [3, 2, 1, 4, 5, 6]
- Pivot index = 4
- Element at index 4: 5
- Average Case: The average time complexity of Quickselect is O(n).
- Worst Case: The worst-case time complexity is O(n^2), but this is rare with good pivot selection.
- In-Place: The space complexity is O(1) because the algorithm works in place.
Final Step: Return the root of the heap
Time Complexity:
Space Complexity:
✅ Best
Note: Even though Leetcode restricts quick select, you should remember this approach because it passes most test cases
//Quick Select Algo function quickSelect(list, left, right, k) if left = right return list[left] Select a pivotIndex between left and right pivotIndex := partition(list, left, right, pivotIndex) if k = pivotIndex return list[k] else if k < pivotIndex right := pivotIndex - 1 else left := pivotIndex + 1
Copy after loginvar findKthLargest = function(nums, k) { // Call the quickSelect function to find the kth largest element return quickSelect(nums, 0, nums.length - 1, nums.length - k); }; function quickSelect(nums, low, high, index) { // If the low and high pointers are the same, return the element at low if (low === high) return nums[low]; // Partition the array and get the pivot index let pivotIndex = partition(nums, low, high); // If the pivot index is the target index, return the element at pivot index if (pivotIndex === index) { return nums[pivotIndex]; } else if (pivotIndex > index) { // If the pivot index is greater than the target index, search in the left partition return quickSelect(nums, low, pivotIndex - 1, index); } else { // If the pivot index is less than the target index, search in the right partition return quickSelect(nums, pivotIndex + 1, high, index); } } function partition(nums, low, high) { // Choose the pivot element let pivot = nums[high]; let pointer = low; // Rearrange the elements based on the pivot for (let i = low; i < high; i++) { if (nums[i] <= pivot) { [nums[i], nums[pointer]] = [nums[pointer], nums[i]]; pointer++; } } // Place the pivot element in its correct position [nums[pointer], nums[high]] = [nums[high], nums[pointer]]; return pointer; }
Copy after loginExplanation:
Step 1: Partition the array
Step 2: Recursive Selection
Step 3: Partition the right partition
Final Step: Return the element at the target index
Time Complexity:
Space Complexity:
The above is the detailed content of Kth Largest Element in an Array. For more information, please follow other related articles on the PHP Chinese website!

Hot AI Tools

Undresser.AI Undress
AI-powered app for creating realistic nude photos

AI Clothes Remover
Online AI tool for removing clothes from photos.

Undress AI Tool
Undress images for free

Clothoff.io
AI clothes remover

Video Face Swap
Swap faces in any video effortlessly with our completely free AI face swap tool!

Hot Article

Hot Tools

Notepad++7.3.1
Easy-to-use and free code editor

SublimeText3 Chinese version
Chinese version, very easy to use

Zend Studio 13.0.1
Powerful PHP integrated development environment

Dreamweaver CS6
Visual web development tools

SublimeText3 Mac version
God-level code editing software (SublimeText3)

Hot Topics




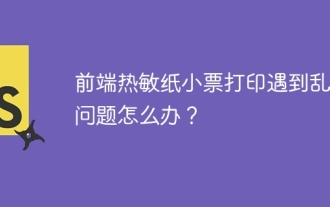
Frequently Asked Questions and Solutions for Front-end Thermal Paper Ticket Printing In Front-end Development, Ticket Printing is a common requirement. However, many developers are implementing...
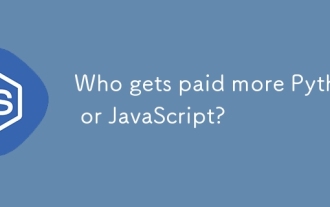
There is no absolute salary for Python and JavaScript developers, depending on skills and industry needs. 1. Python may be paid more in data science and machine learning. 2. JavaScript has great demand in front-end and full-stack development, and its salary is also considerable. 3. Influencing factors include experience, geographical location, company size and specific skills.
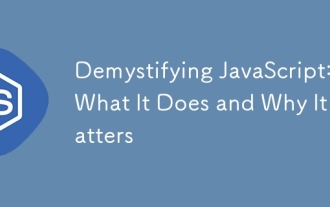
JavaScript is the cornerstone of modern web development, and its main functions include event-driven programming, dynamic content generation and asynchronous programming. 1) Event-driven programming allows web pages to change dynamically according to user operations. 2) Dynamic content generation allows page content to be adjusted according to conditions. 3) Asynchronous programming ensures that the user interface is not blocked. JavaScript is widely used in web interaction, single-page application and server-side development, greatly improving the flexibility of user experience and cross-platform development.
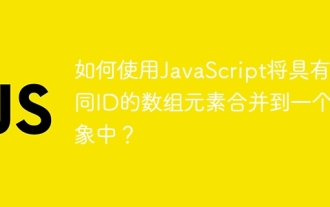
How to merge array elements with the same ID into one object in JavaScript? When processing data, we often encounter the need to have the same ID...
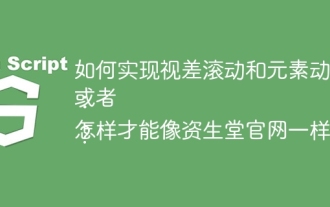
Discussion on the realization of parallax scrolling and element animation effects in this article will explore how to achieve similar to Shiseido official website (https://www.shiseido.co.jp/sb/wonderland/)...
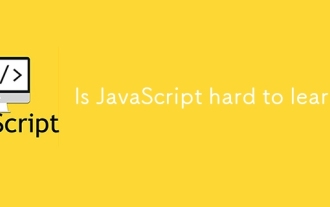
Learning JavaScript is not difficult, but it is challenging. 1) Understand basic concepts such as variables, data types, functions, etc. 2) Master asynchronous programming and implement it through event loops. 3) Use DOM operations and Promise to handle asynchronous requests. 4) Avoid common mistakes and use debugging techniques. 5) Optimize performance and follow best practices.
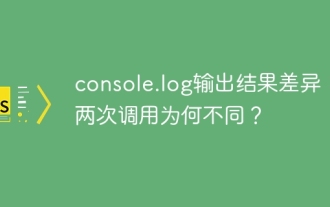
In-depth discussion of the root causes of the difference in console.log output. This article will analyze the differences in the output results of console.log function in a piece of code and explain the reasons behind it. �...
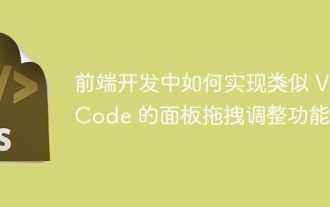
Explore the implementation of panel drag and drop adjustment function similar to VSCode in the front-end. In front-end development, how to implement VSCode similar to VSCode...
