Big O Notation: A Simple Guide
Big O Notation is a mathematical concept used to describe the performance or complexity of an algorithm in terms of time and space as the input size grows. It helps us understand how the runtime of an algorithm increases with larger inputs, allowing for a more standardized comparison of different algorithms.
Why Use Big O Notation?
When comparing algorithms, relying solely on execution time can be misleading. For example, one algorithm might process a massive dataset in one hour, while another takes four hours. However, the execution time can vary based on the machine and other running processes. Instead, we use Big O Notation to focus on the number of operations performed, which provides a more consistent measure of efficiency.
Example: Summing Numbers
Let’s explore two ways to calculate the sum of all numbers from 1 to n:
Option 1: Using a Loop
function addUpTo(n) { let total = 0; for (let i = 1; i <= n; i++) { total += i; } return total; }
Option 2: Using a Formula
function addUpTo(n) { return n * (n + 1) / 2; }
Analyzing the Complexity
In Option 1, if n is 100, the loop runs 100 times. In contrast, Option 2 always executes a fixed number of operations (multiplication, addition, and division). Thus:
- Option 1 is O(n): The time complexity grows linearly with n.
- Option 2 is O(1): The time complexity remains constant, regardless of the input size.
Disclaimer
While Option 2 involves three operations (multiplication, addition, division), we focus on the general trend in Big O analysis. Thus, instead of expressing it as O(3n), we simplify it to O(n). Similarly, O(n 10) simplifies to O(n), and O(n^2 5n 8) simplifies to O(n^2). In Big O Notation, we consider the worst-case scenario, where the highest-order term has the greatest impact on performance.
There are other forms of notation beyond the common complexities listed above, such as logarithmic time complexity expressed as O(log n).
What Is Big O Notation?
Big O Notation allows us to formalize the growth of an algorithm’s runtime based on input size. Rather than focusing on specific operation counts, we categorize algorithms into broader classes including:
- Constant Time: O(1) - The algorithm's performance does not change with the input size.
- Linear Time: O(n) - The performance grows linearly with the input size.
- Quadratic Time: O(n^2) - The performance grows quadratically as the input size increases.
Example of O(n^2)
Consider the following function, which prints all pairs of numbers from 0 to n:
function addUpTo(n) { let total = 0; for (let i = 1; i <= n; i++) { total += i; } return total; }
In this case, the function has two nested loops, so when nnn increases, the number of operations increases quadratically. For n= 2, there are 4 operations, and for n=3, there are 9 operations, leading to O(n^2).
Another Example: Count Up and Down
function addUpTo(n) { return n * (n + 1) / 2; }
At first glance, one might think this is O(n^2) because it contains two loops. However, both loops run independently and scale linearly with n. Thus, the overall time complexity is O(n).
Simplifying the Analysis
Analyzing every aspect of code complexity can be complex, but some general rules can simplify things:
- Arithmetic operations are considered constant time.
- Variable assignments are constant time.
- Accessing elements in an array (by index) or object (by key) is constant time.
- For a loop, the complexity is the length of the loop multiplied by the complexity of what happens inside the loop.
Space Complexity
While we've focused on time complexity, it's also possible to calculate space (memory) complexity using Big O. Some people include input size in their calculations, but it’s often more useful to focus solely on the space required by the algorithm itself.
Rules for Space Complexity (based on JavaScript):
- Most primitive values (booleans, numbers, etc.) are constant space.
- Strings require O(n) space (where n is the string length).
- Reference types (arrays, objects) are generally O(n), where n is the length of the array or the number of keys in the object.
An Example
function printAllPairs(n) { for (var i = 0; i < n; i++) { for (var j = 0; j < n; j++) { console.log(i, j); } } }
In this function, the space complexity is O(1) because we use a constant amount of space (two variables) regardless of the input size.
For a function that creates a new array:
function countUpAndDown(n) { console.log("Going up!"); for (var i = 0; i < n; i++) { console.log(i); } console.log("At the top!\nGoing down..."); for (var j = n - 1; j >= 0; j--) { console.log(j); } console.log("Back down. Bye!"); }
Here, the space complexity is O(n) because we allocate space for a new array that grows with the size of the input array.
Conclusion
Big O Notation provides a framework for analyzing the efficiency of algorithms in a way that is independent of hardware and specific implementation details. Understanding these concepts is crucial for developing efficient code, especially as data sizes grow. By focusing on how performance scales, developers can make informed choices about which algorithms to use in their applications.
The above is the detailed content of Big O Notation: A Simple Guide. For more information, please follow other related articles on the PHP Chinese website!

Hot AI Tools

Undresser.AI Undress
AI-powered app for creating realistic nude photos

AI Clothes Remover
Online AI tool for removing clothes from photos.

Undress AI Tool
Undress images for free

Clothoff.io
AI clothes remover

Video Face Swap
Swap faces in any video effortlessly with our completely free AI face swap tool!

Hot Article

Hot Tools

Notepad++7.3.1
Easy-to-use and free code editor

SublimeText3 Chinese version
Chinese version, very easy to use

Zend Studio 13.0.1
Powerful PHP integrated development environment

Dreamweaver CS6
Visual web development tools

SublimeText3 Mac version
God-level code editing software (SublimeText3)

Hot Topics
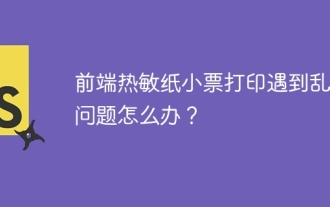
Frequently Asked Questions and Solutions for Front-end Thermal Paper Ticket Printing In Front-end Development, Ticket Printing is a common requirement. However, many developers are implementing...
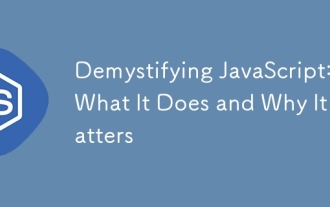
JavaScript is the cornerstone of modern web development, and its main functions include event-driven programming, dynamic content generation and asynchronous programming. 1) Event-driven programming allows web pages to change dynamically according to user operations. 2) Dynamic content generation allows page content to be adjusted according to conditions. 3) Asynchronous programming ensures that the user interface is not blocked. JavaScript is widely used in web interaction, single-page application and server-side development, greatly improving the flexibility of user experience and cross-platform development.
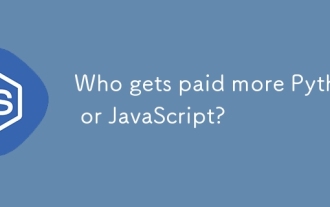
There is no absolute salary for Python and JavaScript developers, depending on skills and industry needs. 1. Python may be paid more in data science and machine learning. 2. JavaScript has great demand in front-end and full-stack development, and its salary is also considerable. 3. Influencing factors include experience, geographical location, company size and specific skills.
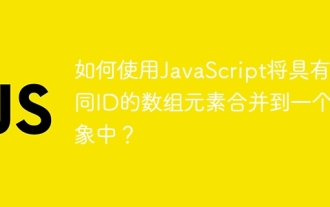
How to merge array elements with the same ID into one object in JavaScript? When processing data, we often encounter the need to have the same ID...
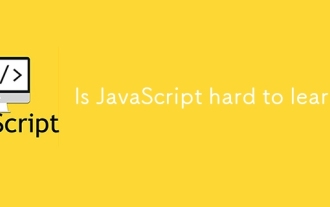
Learning JavaScript is not difficult, but it is challenging. 1) Understand basic concepts such as variables, data types, functions, etc. 2) Master asynchronous programming and implement it through event loops. 3) Use DOM operations and Promise to handle asynchronous requests. 4) Avoid common mistakes and use debugging techniques. 5) Optimize performance and follow best practices.
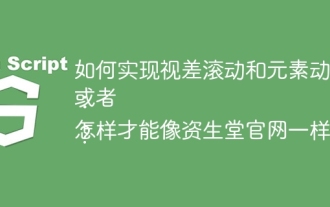
Discussion on the realization of parallax scrolling and element animation effects in this article will explore how to achieve similar to Shiseido official website (https://www.shiseido.co.jp/sb/wonderland/)...
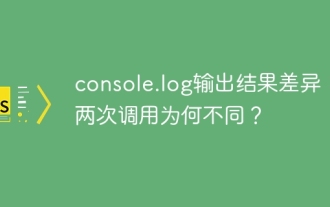
In-depth discussion of the root causes of the difference in console.log output. This article will analyze the differences in the output results of console.log function in a piece of code and explain the reasons behind it. �...
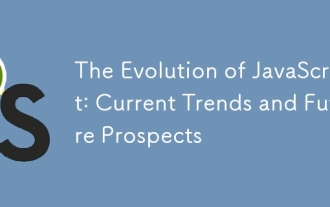
The latest trends in JavaScript include the rise of TypeScript, the popularity of modern frameworks and libraries, and the application of WebAssembly. Future prospects cover more powerful type systems, the development of server-side JavaScript, the expansion of artificial intelligence and machine learning, and the potential of IoT and edge computing.
