How do you Convert VectorUDTs into Columns in PySpark?
Unraveling VectorUDTs into Columns Using PySpark
In PySpark, you may encounter the need to extract individual dimensions from vector columns stored as VectorUDTs. To accomplish this, you can leverage various approaches based on your Spark version.
Spark >= 3.0.0
PySpark 3.0.0 brings built-in functionality for this task:
<code class="python">from pyspark.ml.functions import vector_to_array df.withColumn("xs", vector_to_array("vector")).select(["word"] + [col("xs")[i] for i in range(3)])</code>
This concisely converts the vector into an array and projects the desired columns.
Spark < 3.0.0
Pre-3.0.0 Spark versions require more intricate approaches:
RDD Conversion:
<code class="python">df.rdd.map(lambda row: (row.word,) + tuple(row.vector.toArray().tolist())).toDF(["word"])</code>
UDF Method:
<code class="python">from pyspark.sql.functions import udf, col from pyspark.sql.types import ArrayType, DoubleType def to_array(col): return udf(lambda v: v.toArray().tolist(), ArrayType(DoubleType()))(col) df.withColumn("xs", to_array(col("vector"))).select(["word"] + [col("xs")[i] for i in range(3)])</code>
Note: For increased performance, ensure asNondeterministic is used with the UDF (requires Spark 2.3 ).
Scala Equivalent
For the Scala equivalent of these approaches, refer to "Spark Scala: How to convert Dataframe[vector] to DataFrame[f1:Double, ..., fn: Double)]."
The above is the detailed content of How do you Convert VectorUDTs into Columns in PySpark?. For more information, please follow other related articles on the PHP Chinese website!

Hot AI Tools

Undresser.AI Undress
AI-powered app for creating realistic nude photos

AI Clothes Remover
Online AI tool for removing clothes from photos.

Undress AI Tool
Undress images for free

Clothoff.io
AI clothes remover

Video Face Swap
Swap faces in any video effortlessly with our completely free AI face swap tool!

Hot Article

Hot Tools

Notepad++7.3.1
Easy-to-use and free code editor

SublimeText3 Chinese version
Chinese version, very easy to use

Zend Studio 13.0.1
Powerful PHP integrated development environment

Dreamweaver CS6
Visual web development tools

SublimeText3 Mac version
God-level code editing software (SublimeText3)

Hot Topics










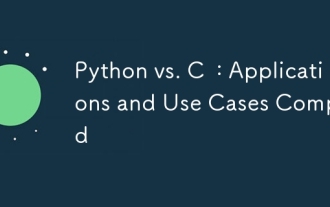
Python is suitable for data science, web development and automation tasks, while C is suitable for system programming, game development and embedded systems. Python is known for its simplicity and powerful ecosystem, while C is known for its high performance and underlying control capabilities.
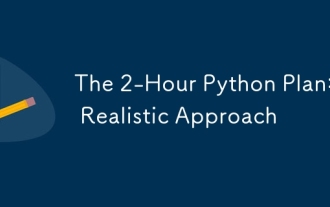
You can learn basic programming concepts and skills of Python within 2 hours. 1. Learn variables and data types, 2. Master control flow (conditional statements and loops), 3. Understand the definition and use of functions, 4. Quickly get started with Python programming through simple examples and code snippets.
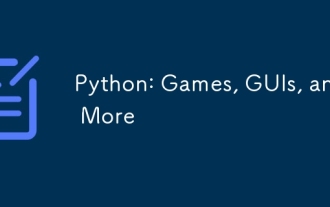
Python excels in gaming and GUI development. 1) Game development uses Pygame, providing drawing, audio and other functions, which are suitable for creating 2D games. 2) GUI development can choose Tkinter or PyQt. Tkinter is simple and easy to use, PyQt has rich functions and is suitable for professional development.
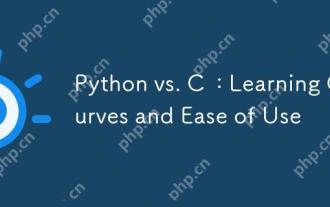
Python is easier to learn and use, while C is more powerful but complex. 1. Python syntax is concise and suitable for beginners. Dynamic typing and automatic memory management make it easy to use, but may cause runtime errors. 2.C provides low-level control and advanced features, suitable for high-performance applications, but has a high learning threshold and requires manual memory and type safety management.
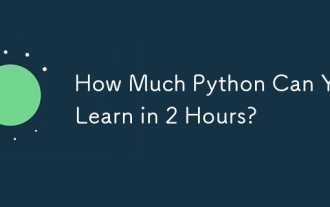
You can learn the basics of Python within two hours. 1. Learn variables and data types, 2. Master control structures such as if statements and loops, 3. Understand the definition and use of functions. These will help you start writing simple Python programs.
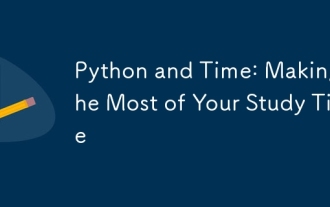
To maximize the efficiency of learning Python in a limited time, you can use Python's datetime, time, and schedule modules. 1. The datetime module is used to record and plan learning time. 2. The time module helps to set study and rest time. 3. The schedule module automatically arranges weekly learning tasks.
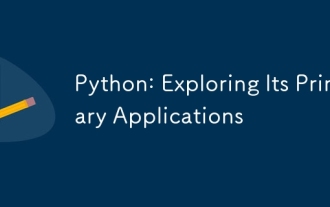
Python is widely used in the fields of web development, data science, machine learning, automation and scripting. 1) In web development, Django and Flask frameworks simplify the development process. 2) In the fields of data science and machine learning, NumPy, Pandas, Scikit-learn and TensorFlow libraries provide strong support. 3) In terms of automation and scripting, Python is suitable for tasks such as automated testing and system management.
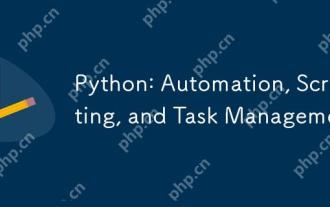
Python excels in automation, scripting, and task management. 1) Automation: File backup is realized through standard libraries such as os and shutil. 2) Script writing: Use the psutil library to monitor system resources. 3) Task management: Use the schedule library to schedule tasks. Python's ease of use and rich library support makes it the preferred tool in these areas.
