


Can Categorical Data Be Directly Processed by Machine Learning Classifiers?
Nov 11, 2024 pm 01:07 PMOne Hot Encoding in Python: A Comprehensive Guide
One hot encoding is a technique used to convert categorical data into binary vectors, enabling machine learning algorithms to process it effectively. When dealing with a classification problem where most of the variables are categorical, one hot encoding is often necessary for accurate predictions.
Can Data Be Passed to a Classifier Without Encoding?
No, it is generally not recommended to pass categorical data directly to a classifier. Most classifiers require numerical inputs, so one hot encoding or other encoding techniques are typically needed to represent categorical features as numbers.
One Hot Encoding Approaches
1. Using pandas.get_dummies()
import pandas as pd df = pd.DataFrame({ 'Gender': ['Male', 'Female', 'Other'], 'Age': [25, 30, 35] }) encoded_df = pd.get_dummies(df, columns=['Gender'])
2. Using Scikit-learn
from sklearn.preprocessing import OneHotEncoder encoder = OneHotEncoder() encoded_data = encoder.fit_transform(df[['Gender']])
Performance Issues with One Hot Encoding
- Large Data Size: One hot encoding can significantly increase the data size, especially with a high number of categorical features.
- Computational Cost: Transforming large datasets into one hot vectors can be computationally expensive.
Alternatives to One Hot Encoding
If one hot encoding is causing performance issues, consider the following alternatives:
- Label Encoding: Converts categorical labels into integers.
- Ordinal Encoding: Assigns ordered numerical values to categorical features based on their rank.
- CountVectorizer (Text Data): A technique specifically designed for text data that converts words or tokens into vectors based on their frequency.
Conclusion
One hot encoding is a valuable technique for handling categorical data in machine learning. By converting categorical features into one hot vectors, classifiers can process them as numerical inputs and make accurate predictions. However, it is important to consider the potential performance issues associated with one hot encoding and explore alternative encoding methods as needed.
The above is the detailed content of Can Categorical Data Be Directly Processed by Machine Learning Classifiers?. For more information, please follow other related articles on the PHP Chinese website!

Hot Article

Hot tools Tags

Hot Article

Hot Article Tags

Notepad++7.3.1
Easy-to-use and free code editor

SublimeText3 Chinese version
Chinese version, very easy to use

Zend Studio 13.0.1
Powerful PHP integrated development environment

Dreamweaver CS6
Visual web development tools

SublimeText3 Mac version
God-level code editing software (SublimeText3)

Hot Topics
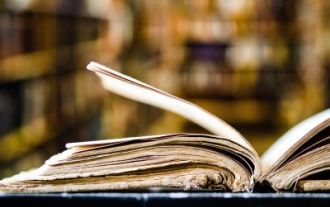
How to Use Python to Find the Zipf Distribution of a Text File
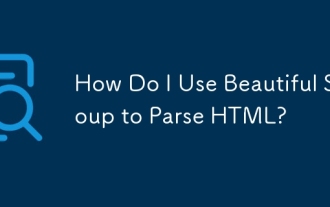
How Do I Use Beautiful Soup to Parse HTML?
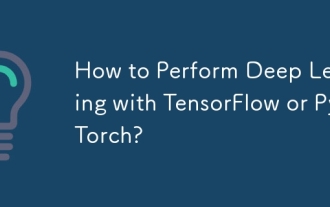
How to Perform Deep Learning with TensorFlow or PyTorch?
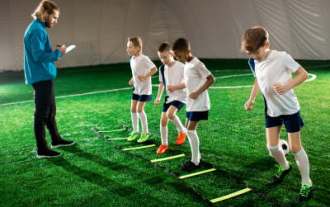
Introduction to Parallel and Concurrent Programming in Python
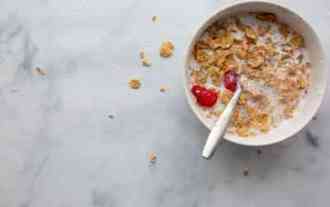
Serialization and Deserialization of Python Objects: Part 1
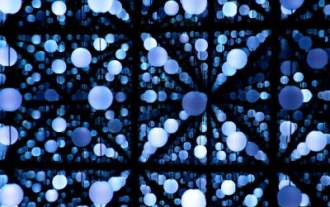
How to Implement Your Own Data Structure in Python
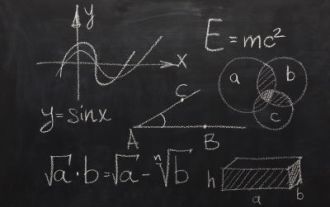
Mathematical Modules in Python: Statistics
