


How to Efficiently Remove Punctuation from Large Text Datasets in Pandas?
How to Remove Punctuation Efficiently with Pandas
Problem:
When pre-processing text data, it's essential to remove punctuation to prepare it for analysis. This task involves identifying and filtering out any character defined as punctuation.
Challenges:
In situations where you're working with a massive amount of text, using built-in functions like pandas' str.replace can be computationally expensive. This becomes especially important when dealing with hundreds of thousands of records.
Solutions:
This question explores several performant alternatives to str.replace when dealing with large text datasets:
1. Regex.sub:
Utilizes the sub function from the re library with a pre-compiled regex pattern. This method offers a significant performance improvement over str.replace.
2. str.translate:
Leverages Python's str.translate function, which is implemented in C and known for its speed. The process involves converting the input strings into one large string, applying translation to remove punctuation, and then splitting the result to reconstruct the original strings.
3. Other Considerations:
- Handling NaNs: List comprehension methods like regex.sub don't work with NaNs. You'll need to handle them separately by identifying their indices and applying the substitution only to non-null values.
- DataFrames: To apply these methods to entire DataFrames, you can flatten the values and perform the substitution on the flattened array before reshaping it back to the original shape.
Performance Analysis:
Through benchmarking, it's found that str.translate consistently outperforms the other methods, especially for larger datasets. It's important to consider the tradeoff between performance and memory usage, as str.translate requires more memory.
Conclusion:
The appropriate method for removing punctuation depends on the specific requirements of your situation. If performance is the top priority, str.translate provides the best option. However, if memory usage is a concern, other methods like regex.sub can be more suitable.
The above is the detailed content of How to Efficiently Remove Punctuation from Large Text Datasets in Pandas?. For more information, please follow other related articles on the PHP Chinese website!

Hot AI Tools

Undresser.AI Undress
AI-powered app for creating realistic nude photos

AI Clothes Remover
Online AI tool for removing clothes from photos.

Undress AI Tool
Undress images for free

Clothoff.io
AI clothes remover

AI Hentai Generator
Generate AI Hentai for free.

Hot Article

Hot Tools

Notepad++7.3.1
Easy-to-use and free code editor

SublimeText3 Chinese version
Chinese version, very easy to use

Zend Studio 13.0.1
Powerful PHP integrated development environment

Dreamweaver CS6
Visual web development tools

SublimeText3 Mac version
God-level code editing software (SublimeText3)

Hot Topics


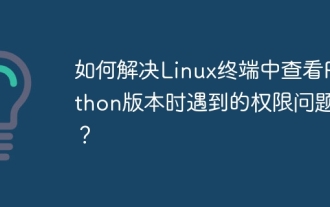
Solution to permission issues when viewing Python version in Linux terminal When you try to view Python version in Linux terminal, enter python...
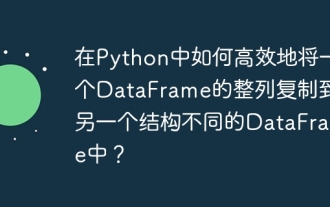
When using Python's pandas library, how to copy whole columns between two DataFrames with different structures is a common problem. Suppose we have two Dats...
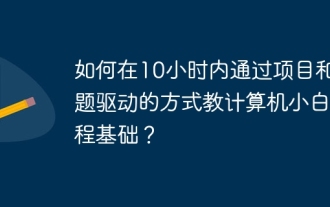
How to teach computer novice programming basics within 10 hours? If you only have 10 hours to teach computer novice some programming knowledge, what would you choose to teach...
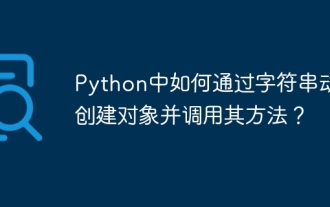
In Python, how to dynamically create an object through a string and call its methods? This is a common programming requirement, especially if it needs to be configured or run...
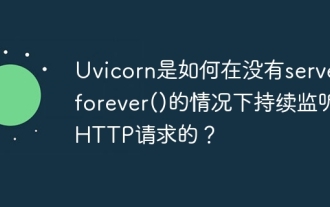
How does Uvicorn continuously listen for HTTP requests? Uvicorn is a lightweight web server based on ASGI. One of its core functions is to listen for HTTP requests and proceed...
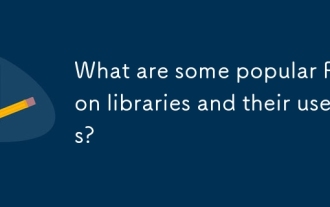
The article discusses popular Python libraries like NumPy, Pandas, Matplotlib, Scikit-learn, TensorFlow, Django, Flask, and Requests, detailing their uses in scientific computing, data analysis, visualization, machine learning, web development, and H
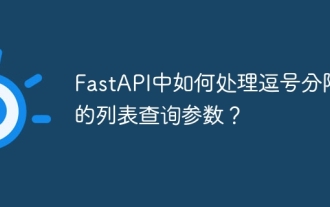
Fastapi ...
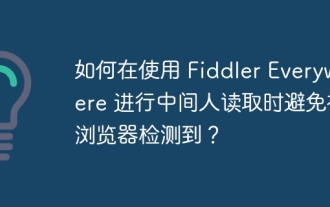
How to avoid being detected when using FiddlerEverywhere for man-in-the-middle readings When you use FiddlerEverywhere...
