Agent Tool Development Guide: From Design to Optimization
1. Introduction
Imagine you're assembling a super-intelligent robot butler (Agent). This robot needs various tools to help you complete tasks - just like Doraemon's 4D pocket. This article will teach you how to create these powerful tools to make your AI butler more capable and efficient.
2. Two Core Tool Design Patterns
2.1 Synchronous Tools: Instant Response Mode
Think of using a self-service coffee machine:
- Insert coins and press the "Americano" button
- Wait for a few seconds
- Coffee flows out, ready to drink
This is a typical synchronous tool pattern. The Agent calls the tool and waits for immediate results - quick and simple.
class WeatherTool(BaseTool): """Weather Query Tool - Synchronous Mode""" async def execute(self, city: str) -> dict: # Simple and direct like pressing a coffee machine button weather_data = await self.weather_api.get_current(city) return { "status": "success", "data": { "temperature": weather_data.temp, "humidity": weather_data.humidity, "description": weather_data.desc } }
Use cases:
- Quick queries: weather, exchange rates, simple calculations
- Simple operations: sending messages, switch controls
- Real-time feedback: verification code checks, balance inquiries
2.2 Asynchronous Tools: Task Tracking Mode
Imagine ordering food through a delivery APP:
- After placing an order, the APP gives you an order number
- You can check the order status anytime
- The APP notifies you when delivery is complete
This is how asynchronous tools work, perfect for tasks that take longer to process.
class DocumentAnalysisTool(BaseTool): """Document Analysis Tool - Asynchronous Mode""" async def start_task(self, file_path: str) -> str: # Like placing a food delivery order, returns a task ID task_id = str(uuid.uuid4()) await self.task_queue.put({ "task_id": task_id, "file_path": file_path, "status": "processing" }) return task_id async def get_status(self, task_id: str) -> dict: # Like checking food delivery status task = await self.task_store.get(task_id) return { "task_id": task_id, "status": task["status"], "progress": task.get("progress", 0), "result": task.get("result", None) }
Use cases:
- Time-consuming operations: large file processing, data analysis
- Multi-step tasks: video rendering, report generation
- Progress tracking needed: model training, batch processing
3. Tool Interface Standardization: Establishing Universal Specifications
Just like all electrical appliances follow unified socket standards, our tool interfaces need standardization. This ensures all tools work perfectly with the Agent.
3.1 Tool Description Specifications
Imagine writing a product manual, you need to clearly tell users:
- What the tool does
- What parameters are needed
- What results will be returned
from pydantic import BaseModel, Field class ToolSchema(BaseModel): """Tool Manual Template""" name: str = Field(..., description="Tool name") description: str = Field(..., description="Tool purpose description") parameters: dict = Field(..., description="Required parameters") required: List[str] = Field(default_factory=list, description="Required parameters") class Config: schema_extra = { "example": { "name": "Weather Query", "description": "Query weather information for specified city", "parameters": { "type": "object", "properties": { "city": { "type": "string", "description": "City name" } } }, "required": ["city"] } }
3.2 Unified Base Class
Just like all electrical appliances need power switches and power interfaces, all tools need to follow basic specifications:
class BaseTool(ABC): """Base template for all tools""" @abstractmethod def get_schema(self) -> ToolSchema: """Tool manual""" pass def validate_input(self, params: Dict) -> Dict: """Parameter check, like a fuse in electrical appliances""" return ToolSchema(**params).dict() @abstractmethod async def execute(self, **kwargs) -> Dict: """Actual functionality execution""" pass
4. Error Handling: Making Tools More Reliable
Just like household appliances need protection against water, shock, and overload, tools need comprehensive protection mechanisms.
4.1 Error Classification and Handling
Imagine handling express delivery:
- Wrong address → Parameter error
- System maintenance → Service temporarily unavailable
- Courier too busy → Need rate limiting and retry
class WeatherTool(BaseTool): """Weather Query Tool - Synchronous Mode""" async def execute(self, city: str) -> dict: # Simple and direct like pressing a coffee machine button weather_data = await self.weather_api.get_current(city) return { "status": "success", "data": { "temperature": weather_data.temp, "humidity": weather_data.humidity, "description": weather_data.desc } }
4.2 Retry Mechanism
Like automatically arranging a second delivery when the first attempt fails:
class DocumentAnalysisTool(BaseTool): """Document Analysis Tool - Asynchronous Mode""" async def start_task(self, file_path: str) -> str: # Like placing a food delivery order, returns a task ID task_id = str(uuid.uuid4()) await self.task_queue.put({ "task_id": task_id, "file_path": file_path, "status": "processing" }) return task_id async def get_status(self, task_id: str) -> dict: # Like checking food delivery status task = await self.task_store.get(task_id) return { "task_id": task_id, "status": task["status"], "progress": task.get("progress", 0), "result": task.get("result", None) }
5. Performance Optimization: Making Tools More Efficient
5.1 Caching Mechanism
Like a convenience store placing popular items in prominent positions:
from pydantic import BaseModel, Field class ToolSchema(BaseModel): """Tool Manual Template""" name: str = Field(..., description="Tool name") description: str = Field(..., description="Tool purpose description") parameters: dict = Field(..., description="Required parameters") required: List[str] = Field(default_factory=list, description="Required parameters") class Config: schema_extra = { "example": { "name": "Weather Query", "description": "Query weather information for specified city", "parameters": { "type": "object", "properties": { "city": { "type": "string", "description": "City name" } } }, "required": ["city"] } }
5.2 Concurrency Control
Like a hospital's appointment system, controlling the number of simultaneous services:
class BaseTool(ABC): """Base template for all tools""" @abstractmethod def get_schema(self) -> ToolSchema: """Tool manual""" pass def validate_input(self, params: Dict) -> Dict: """Parameter check, like a fuse in electrical appliances""" return ToolSchema(**params).dict() @abstractmethod async def execute(self, **kwargs) -> Dict: """Actual functionality execution""" pass
6. Testing and Documentation: Ensuring Tool Reliability
6.1 Unit Testing
Like quality inspection before a new product launch:
class ToolError(Exception): """Tool error base class""" def __init__(self, message: str, error_code: str, retry_after: Optional[int] = None): self.message = message self.error_code = error_code self.retry_after = retry_after @error_handler async def execute(self, **kwargs): try: # Execute specific operation result = await self._do_work(**kwargs) return {"status": "success", "data": result} except ValidationError: # Parameter error, like wrong address return {"status": "error", "code": "INVALID_PARAMS"} except RateLimitError as e: # Need rate limiting, like courier too busy return { "status": "error", "code": "RATE_LIMIT", "retry_after": e.retry_after }
6.2 Documentation Standards
Like writing a detailed and clear product manual:
class RetryableTool(BaseTool): @retry( stop=stop_after_attempt(3), # Maximum 3 retries wait=wait_exponential(multiplier=1, min=4, max=10) # Increasing wait time ) async def execute_with_retry(self, **kwargs): return await self.execute(**kwargs)
7. Summary
Developing good Agent tools is like crafting a perfect toolbox:
- Proper tool classification - Sync/Async each has its use
- Standardized interfaces - Easy for unified management
- Protection mechanisms - Handle various exceptions
- Pursuit of efficiency - Cache when needed, rate limit when necessary
- Quality focus - Thorough testing, clear documentation
Remember: Good tools can make Agents twice as effective, while poor tools will limit Agents at every turn.
The above is the detailed content of Agent Tool Development Guide: From Design to Optimization. For more information, please follow other related articles on the PHP Chinese website!

Hot AI Tools

Undresser.AI Undress
AI-powered app for creating realistic nude photos

AI Clothes Remover
Online AI tool for removing clothes from photos.

Undress AI Tool
Undress images for free

Clothoff.io
AI clothes remover

Video Face Swap
Swap faces in any video effortlessly with our completely free AI face swap tool!

Hot Article

Hot Tools

Notepad++7.3.1
Easy-to-use and free code editor

SublimeText3 Chinese version
Chinese version, very easy to use

Zend Studio 13.0.1
Powerful PHP integrated development environment

Dreamweaver CS6
Visual web development tools

SublimeText3 Mac version
God-level code editing software (SublimeText3)

Hot Topics










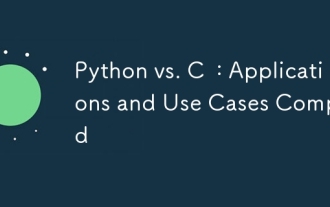
Python is suitable for data science, web development and automation tasks, while C is suitable for system programming, game development and embedded systems. Python is known for its simplicity and powerful ecosystem, while C is known for its high performance and underlying control capabilities.
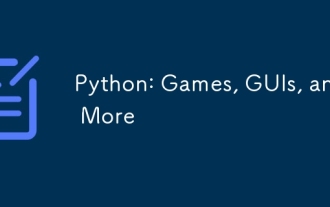
Python excels in gaming and GUI development. 1) Game development uses Pygame, providing drawing, audio and other functions, which are suitable for creating 2D games. 2) GUI development can choose Tkinter or PyQt. Tkinter is simple and easy to use, PyQt has rich functions and is suitable for professional development.
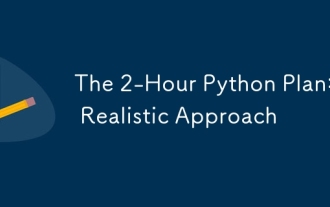
You can learn basic programming concepts and skills of Python within 2 hours. 1. Learn variables and data types, 2. Master control flow (conditional statements and loops), 3. Understand the definition and use of functions, 4. Quickly get started with Python programming through simple examples and code snippets.
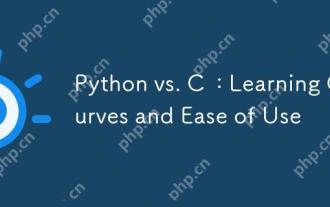
Python is easier to learn and use, while C is more powerful but complex. 1. Python syntax is concise and suitable for beginners. Dynamic typing and automatic memory management make it easy to use, but may cause runtime errors. 2.C provides low-level control and advanced features, suitable for high-performance applications, but has a high learning threshold and requires manual memory and type safety management.
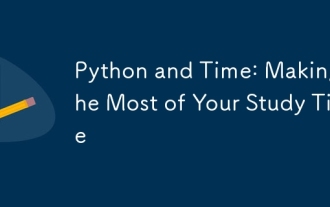
To maximize the efficiency of learning Python in a limited time, you can use Python's datetime, time, and schedule modules. 1. The datetime module is used to record and plan learning time. 2. The time module helps to set study and rest time. 3. The schedule module automatically arranges weekly learning tasks.
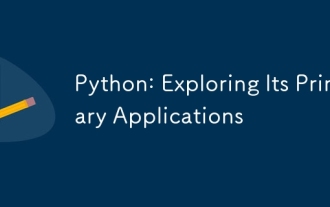
Python is widely used in the fields of web development, data science, machine learning, automation and scripting. 1) In web development, Django and Flask frameworks simplify the development process. 2) In the fields of data science and machine learning, NumPy, Pandas, Scikit-learn and TensorFlow libraries provide strong support. 3) In terms of automation and scripting, Python is suitable for tasks such as automated testing and system management.
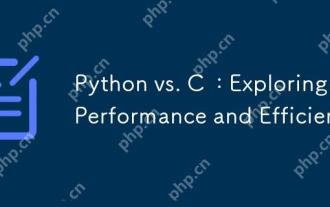
Python is better than C in development efficiency, but C is higher in execution performance. 1. Python's concise syntax and rich libraries improve development efficiency. 2.C's compilation-type characteristics and hardware control improve execution performance. When making a choice, you need to weigh the development speed and execution efficiency based on project needs.
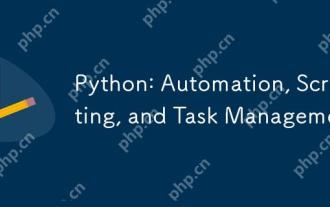
Python excels in automation, scripting, and task management. 1) Automation: File backup is realized through standard libraries such as os and shutil. 2) Script writing: Use the psutil library to monitor system resources. 3) Task management: Use the schedule library to schedule tasks. Python's ease of use and rich library support makes it the preferred tool in these areas.
