Buy Me a Coffee☕
*Memos:
-
My post explains Vanishing Gradient Problem, Exploding Gradient Problem and Dying ReLU Problem.
-
My post explains layers in PyTorch.
-
My post explains activation functions in PyTorch.
-
My post explains loss functions in PyTorch.
-
My post explains optimizers in PyTorch.
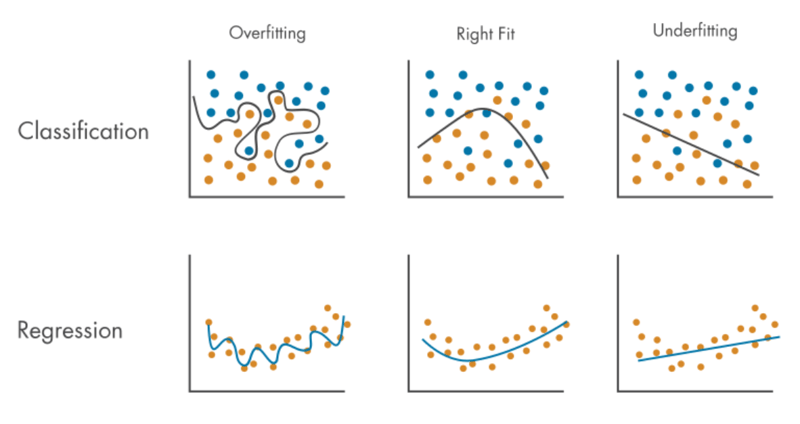
*Both overfitting and underfitting can be detected by Holdout Method or Cross Validation(K-Fold Cross-Validation). *Cross Validation is better.
Overfitting:
- is the problem which a model can make accurate predictions for train data a lot but a little for new data(including test data) so the model fits train data much more than new data.
- occurs because:
- train data is small(not enough) so the model can only learn a small number of patterns.
- train data is imbalanced(biased) having a lot of specific(limitted), similar or same data but not a lot of various data so the model can only learn a small number of patterns.
- train data has a lot of noise(noisy data) so the model learns the patterns of the noise a lot but not the patters of normal data. *Noise(noisy data) means outliers, anomalies or sometimes duplicated data.
- the training time is too long with a too large number of epochs.
- the model is too complex.
- can be mitigated by:
- larger train data.
- having a lot of various data.
- reduceing noise.
- shuffling dataset.
- stopping training early.
- Ensemble learning.
- Regularization to reduce model complexity:
*Memos:
- There is Dropout (Regularization). *My post explains Dropout layer.
- There is L1 Regularization also called L1 Norm or Lasso Regression.
- There is L2 Regularization also called L2 Norm or Ridge Regression.
-
My post explains linalg.norm().
-
My post explains linalg.vector_norm().
-
My post explains linalg.matrix_norm().
Underfitting:
- is the problem which a model cannot make accurate predictions both for train data and new data(including test data) a lot so the model doesn't fit both train data and new data.
- occurs because:
- the model is too simple(not complex enough).
- the training time is too short with a too small number of epochs.
- Excessive regularization(Dropout, L1 and L2 regularization) is applied.
- can be mitigated by:
- Increasing model complexity.
- Increasing the training time with a larger number of epochs.
- Decreasing regularization.
Overfitting and Underfitting are trade-off:
Too much overfitting mitigation(5., 6. and 7.) leads to underfitting with high bias and low variance while too much underfitting mitigation(1., 2. and 3.) leads to overfitting with low bias and high variance so their mitigation should be balanced as shown below:
*Memos:
- You can also say Bias and Variance are trade-off because reducing bias increases variance while reducing variance increases bias so they should be balanced. *Increasing model complexity reduces bias but increases variance while reducing model complexity reduces variance but increases bias.
- Low bias means high accuracy while high bias means low accuracy.
- Low variance means high precision while high variance means low precision.
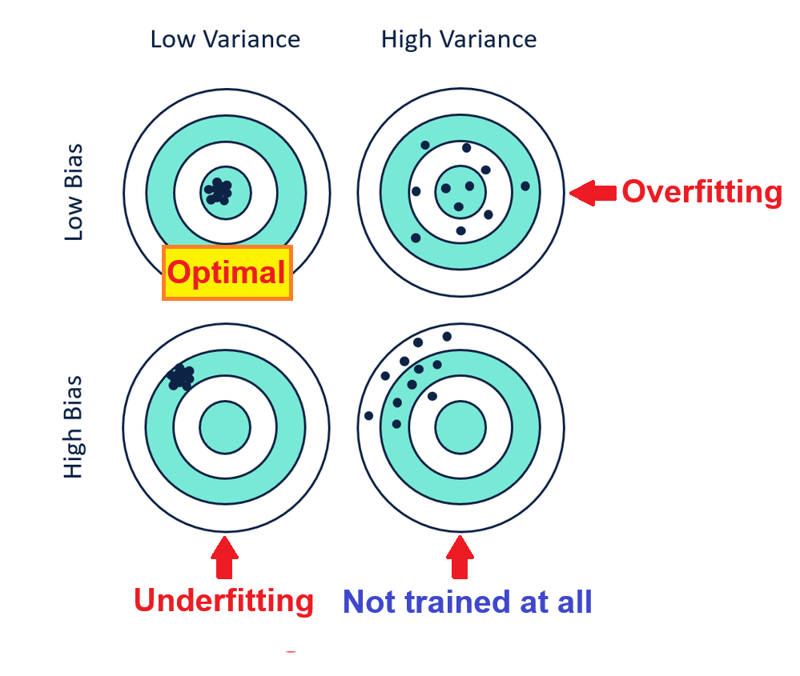
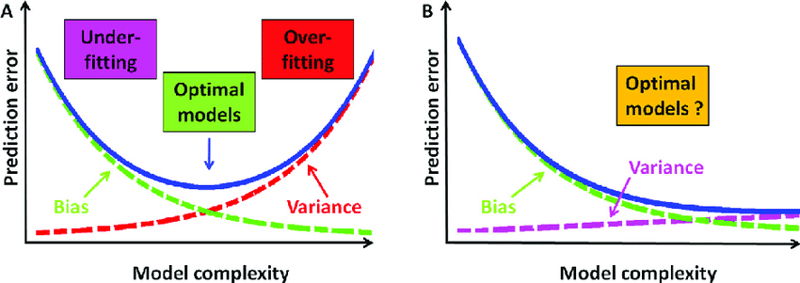
The above is the detailed content of Overfitting vs Underfitting. For more information, please follow other related articles on the PHP Chinese website!