


Exploring the Magic of Hz: Building a Music Frequency Analyzer
In the realm of music and sound, there's a fascinating debate about frequency that has captured the attention of musicians, historians, and scientists alike. At the heart of this discussion lies the number 432 Hz, often referred to as the "natural frequency of the universe." Today, I'll take you through my journey of building a web application that analyzes audio files to determine if they're tuned to this mystical frequency.
The Historical Context
Before we dive into the technical details, let's understand why 432 Hz matters. This frequency wasn't chosen arbitrarily – it has deep historical roots. Musical legends like Bach and Beethoven tuned their instruments to A=432 Hz, considering it the natural tuning that resonates with the universe itself.
However, this changed during World War II when the standard was shifted to 440 Hz. Some argue that 440 Hz creates a subtle sense of tension and anxiety, comparing it to radio static. In contrast, 432 Hz is said to promote harmony and a natural flow in music. Whether you believe in these effects or not, the technical challenge of analyzing audio frequencies remains fascinating.
Technical Overview
Our application is built using modern web technologies and scientific computing libraries:
- Backend: FastAPI (Python)
- Audio Processing: pydub, numpy, scipy
- Frontend: Web interface for file uploads
- Analysis: Fast Fourier Transform (FFT) for frequency detection
The Science Behind Frequency Analysis
At the core of our application lies the Fast Fourier Transform (FFT) algorithm. FFT transforms our audio signal from the time domain to the frequency domain, allowing us to identify the dominant frequencies in a piece of music.
Here's how the analysis works:
- Audio Input Processing
audio = AudioSegment.from_file(io.BytesIO(file_content)).set_channels(1) # Convert to mono samples = np.array(audio.get_array_of_samples()) sample_rate = audio.frame_rate
- Frequency Analysis
fft_vals = rfft(samples) fft_freqs = rfftfreq(len(samples), d=1/sample_rate) dominant_freq = fft_freqs[np.argmax(np.abs(fft_vals))]
- Result Interpretation
tolerance = 5 # Hz result = ( f"The dominant frequency is {dominant_freq:.2f} Hz, " f"{'close to' if abs(dominant_freq - 432) <= tolerance else 'not close to'} 432Hz." )
Technical Implementation Details
Backend Architecture
Our FastAPI backend handles the heavy lifting of audio processing. Here are the key features:
-
File Validation
- Ensures uploaded files are audio formats
- Limits file size to 20MB
- Validates audio stream integrity
-
Audio Processing Pipeline
- Converts audio to mono for consistent analysis
- Extracts raw samples for FFT processing
- Applies FFT to identify frequency components
-
Error Handling
- Graceful handling of invalid files
- Clear error messages for unsupported formats
- Robust exception handling for processing errors
API Design
The API is simple yet effective:
audio = AudioSegment.from_file(io.BytesIO(file_content)).set_channels(1) # Convert to mono samples = np.array(audio.get_array_of_samples()) sample_rate = audio.frame_rate
User Experience
The application provides a straightforward interface:
- Upload any supported audio file
- Receive instant analysis of the dominant frequency
- Get clear feedback on how close the frequency is to 432 Hz
- View detailed interpretation of the frequency's meaning and significance
Frequency Interpretation
One of the key features is the intelligent interpretation of frequencies. The application not only tells you the dominant frequency but also explains its significance:
fft_vals = rfft(samples) fft_freqs = rfftfreq(len(samples), d=1/sample_rate) dominant_freq = fft_freqs[np.argmax(np.abs(fft_vals))]
The interpretation system provides context for different frequency ranges:
- 432 Hz (±5 Hz): Explains the historical significance and natural alignment
- 440 Hz (±5 Hz): Details about the modern standard tuning
- Below 432 Hz: Information about lower frequency characteristics
- Above 432 Hz: Insights into higher frequency properties
This feature helps users understand not just the numerical value of the frequency, but also its musical and historical context, making the tool more educational and engaging.
Technical Challenges and Solutions
Challenge 1: Audio Format Compatibility
- Solution: Using pydub for broad format support
- Implemented format validation before processing
Challenge 2: Processing Large Files
- Solution: Implemented file size limits
- Added streaming support for efficient memory usage
Challenge 3: Accuracy vs. Performance
- Solution: Balanced FFT window size
- Implemented tolerance range for practical results
Future Improvements
-
Enhanced Analysis
- Multiple frequency detection
- Harmonic analysis
- Time-based frequency tracking
-
User Features
- Batch file processing
- Frequency visualization
- Audio pitch shifting to 432 Hz
Conclusion
Building this frequency analyzer has been an exciting journey through the intersection of music, history, and technology. Whether you're a musician interested in the 432 Hz phenomenon or a developer curious about audio processing, I hope this project provides valuable insights into how we can analyze and understand the frequencies that make up our musical world.
The complete source code is available on GitHub, and I welcome contributions and suggestions for improvements. Feel free to experiment with different audio files and explore the fascinating world of frequency analysis!
Note: This project is open-source and available for educational purposes. The frequency analysis is meant for experimental use and may not be suitable for professional audio tuning applications.
reyesvicente
/
432Hz-Frequency-Checker
This project checks if the frequency of a song is 432Hz or not.
This project checks if the frequency of a song is 432Hz or not.
Why 432Hz?
432Hz is considered the natural frequency of the universe, embraced by great composers like Bach and Beethoven to craft music that touches the soul. This indicates that the universal music scale utilized 432A for tuning their instruments. However, during World War II, this was altered to 440Hz, which resembles the static of a radio—disorienting and unsettling. In contrast, 432Hz fosters harmony and a sense of flow. It’s the ideal frequency, one that feels organic and uplifting! Nature truly is wonderful!
Run the backend:
audio = AudioSegment.from_file(io.BytesIO(file_content)).set_channels(1) # Convert to mono samples = np.array(audio.get_array_of_samples()) sample_rate = audio.frame_rate
Run the frontend
fft_vals = rfft(samples) fft_freqs = rfftfreq(len(samples), d=1/sample_rate) dominant_freq = fft_freqs[np.argmax(np.abs(fft_vals))]
The above is the detailed content of Exploring the Magic of Hz: Building a Music Frequency Analyzer. For more information, please follow other related articles on the PHP Chinese website!

Hot AI Tools

Undresser.AI Undress
AI-powered app for creating realistic nude photos

AI Clothes Remover
Online AI tool for removing clothes from photos.

Undress AI Tool
Undress images for free

Clothoff.io
AI clothes remover

Video Face Swap
Swap faces in any video effortlessly with our completely free AI face swap tool!

Hot Article

Hot Tools

Notepad++7.3.1
Easy-to-use and free code editor

SublimeText3 Chinese version
Chinese version, very easy to use

Zend Studio 13.0.1
Powerful PHP integrated development environment

Dreamweaver CS6
Visual web development tools

SublimeText3 Mac version
God-level code editing software (SublimeText3)

Hot Topics










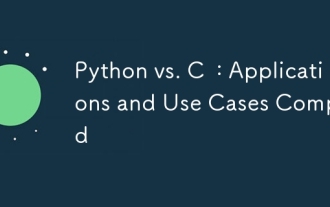
Python is suitable for data science, web development and automation tasks, while C is suitable for system programming, game development and embedded systems. Python is known for its simplicity and powerful ecosystem, while C is known for its high performance and underlying control capabilities.
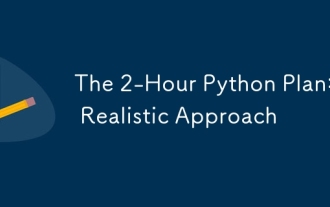
You can learn basic programming concepts and skills of Python within 2 hours. 1. Learn variables and data types, 2. Master control flow (conditional statements and loops), 3. Understand the definition and use of functions, 4. Quickly get started with Python programming through simple examples and code snippets.
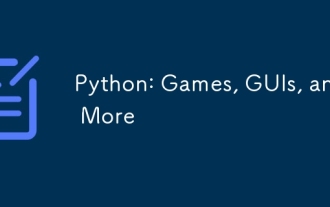
Python excels in gaming and GUI development. 1) Game development uses Pygame, providing drawing, audio and other functions, which are suitable for creating 2D games. 2) GUI development can choose Tkinter or PyQt. Tkinter is simple and easy to use, PyQt has rich functions and is suitable for professional development.
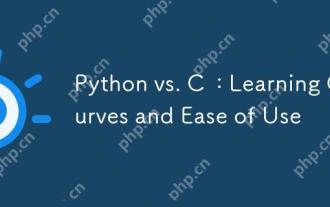
Python is easier to learn and use, while C is more powerful but complex. 1. Python syntax is concise and suitable for beginners. Dynamic typing and automatic memory management make it easy to use, but may cause runtime errors. 2.C provides low-level control and advanced features, suitable for high-performance applications, but has a high learning threshold and requires manual memory and type safety management.
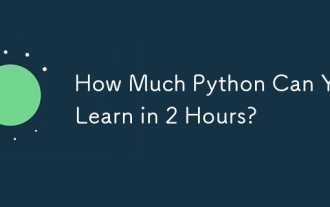
You can learn the basics of Python within two hours. 1. Learn variables and data types, 2. Master control structures such as if statements and loops, 3. Understand the definition and use of functions. These will help you start writing simple Python programs.
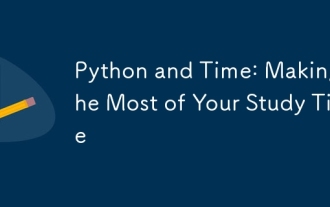
To maximize the efficiency of learning Python in a limited time, you can use Python's datetime, time, and schedule modules. 1. The datetime module is used to record and plan learning time. 2. The time module helps to set study and rest time. 3. The schedule module automatically arranges weekly learning tasks.
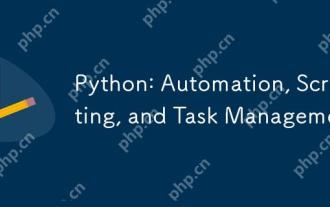
Python excels in automation, scripting, and task management. 1) Automation: File backup is realized through standard libraries such as os and shutil. 2) Script writing: Use the psutil library to monitor system resources. 3) Task management: Use the schedule library to schedule tasks. Python's ease of use and rich library support makes it the preferred tool in these areas.
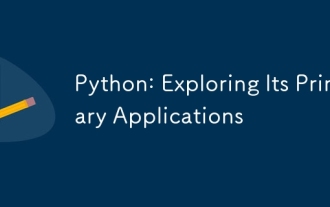
Python is widely used in the fields of web development, data science, machine learning, automation and scripting. 1) In web development, Django and Flask frameworks simplify the development process. 2) In the fields of data science and machine learning, NumPy, Pandas, Scikit-learn and TensorFlow libraries provide strong support. 3) In terms of automation and scripting, Python is suitable for tasks such as automated testing and system management.
