


How to Efficiently Extract Rows from One Pandas DataFrame that are Absent in Another?
Retrieving Rows from One Dataframe that are Excluded from Another
In pandas, it is common to have multiple dataframes with potentially overlapping data. One task that frequently arises is isolating rows from one dataframe that are not present in another. This operation is particularly useful when working with subsets or filtering data.
Problem Formulation:
Given two pandas dataframes, where df1 contains a superset of rows compared to df2, we aim to obtain the rows in df1 that are not found in df2. The example below illustrates this scenario with a simple case:
import pandas as pd df1 = pd.DataFrame(data={'col1': [1, 2, 3, 4, 5], 'col2': [10, 11, 12, 13, 14]}) df2 = pd.DataFrame(data={'col1': [1, 2, 3], 'col2': [10, 11, 12]}) print(df1) print(df2) # Expected result: # col1 col2 # 3 4 13 # 4 5 14
Solution:
To effectively address this problem, we employ a technique known as a left join. This operation merges df1 and df2 while ensuring that all rows from df1 are retained. Additionally, we include an indicator column to identify the origin of each row after the merge. By leveraging the unique rows from df2 and excluding duplicates, we achieve the desired result.
The python code below implements this solution:
df_all = df1.merge(df2.drop_duplicates(), on=['col1', 'col2'], how='left', indicator=True) result = df_all[df_all['_merge'] == 'left_only']
Explanation:
- Left Join: The merge function performs a left join between df1 and df2.drop_duplicates(). This operation merges rows from df1 with rows from df2 based on the matching values in columns col1 and col2.
- Merge Indicator: The indicator parameter is set to True to include an extra column named _merge in the resulting dataframe df_all. This column indicates the origin of each row: 'both' for rows that exist in both df1 and df2, 'left_only' for rows exclusive to df1, and 'right_only' for rows exclusive to df2.
- Filter by 'left_only': To isolate rows from df1 that are not in df2, we filter the df_all dataframe by checking rows with _merge equal to 'left_only'. This gives us the desired result.
Avoiding Common Pitfalls:
It is important to note that some solutions may incorrectly check for individual column values instead of matching rows as a whole. Such approaches may lead to incorrect results, as illustrated in the example below:
~df1.col1.isin(common.col1) & ~df1.col2.isin(common.col2)
This code does not consider the joint occurrence of values in rows and may produce incorrect results when rows in df1 have values that appear individually in df2 but not in the same row.
By adopting the left join approach described above, we ensure that the derived rows are correctly identified as exclusive to df1. This technique provides a reliable and efficient solution to extracting rows that are present in one dataframe but not in another.
The above is the detailed content of How to Efficiently Extract Rows from One Pandas DataFrame that are Absent in Another?. For more information, please follow other related articles on the PHP Chinese website!

Hot AI Tools

Undresser.AI Undress
AI-powered app for creating realistic nude photos

AI Clothes Remover
Online AI tool for removing clothes from photos.

Undress AI Tool
Undress images for free

Clothoff.io
AI clothes remover

Video Face Swap
Swap faces in any video effortlessly with our completely free AI face swap tool!

Hot Article

Hot Tools

Notepad++7.3.1
Easy-to-use and free code editor

SublimeText3 Chinese version
Chinese version, very easy to use

Zend Studio 13.0.1
Powerful PHP integrated development environment

Dreamweaver CS6
Visual web development tools

SublimeText3 Mac version
God-level code editing software (SublimeText3)

Hot Topics










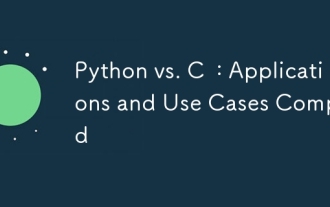
Python is suitable for data science, web development and automation tasks, while C is suitable for system programming, game development and embedded systems. Python is known for its simplicity and powerful ecosystem, while C is known for its high performance and underlying control capabilities.
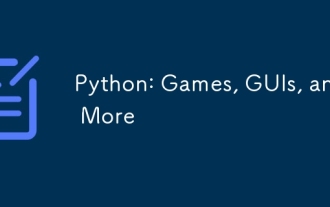
Python excels in gaming and GUI development. 1) Game development uses Pygame, providing drawing, audio and other functions, which are suitable for creating 2D games. 2) GUI development can choose Tkinter or PyQt. Tkinter is simple and easy to use, PyQt has rich functions and is suitable for professional development.
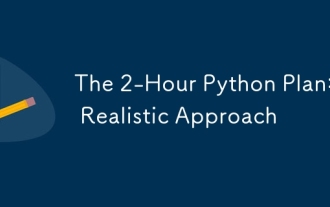
You can learn basic programming concepts and skills of Python within 2 hours. 1. Learn variables and data types, 2. Master control flow (conditional statements and loops), 3. Understand the definition and use of functions, 4. Quickly get started with Python programming through simple examples and code snippets.
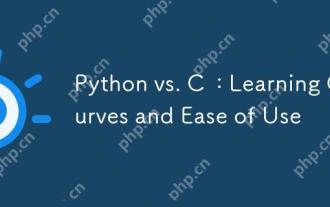
Python is easier to learn and use, while C is more powerful but complex. 1. Python syntax is concise and suitable for beginners. Dynamic typing and automatic memory management make it easy to use, but may cause runtime errors. 2.C provides low-level control and advanced features, suitable for high-performance applications, but has a high learning threshold and requires manual memory and type safety management.
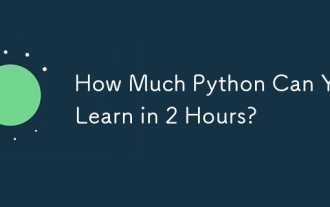
You can learn the basics of Python within two hours. 1. Learn variables and data types, 2. Master control structures such as if statements and loops, 3. Understand the definition and use of functions. These will help you start writing simple Python programs.
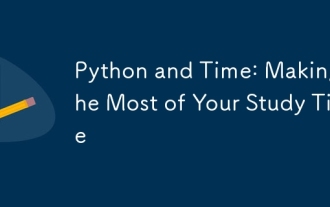
To maximize the efficiency of learning Python in a limited time, you can use Python's datetime, time, and schedule modules. 1. The datetime module is used to record and plan learning time. 2. The time module helps to set study and rest time. 3. The schedule module automatically arranges weekly learning tasks.
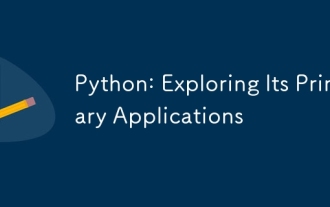
Python is widely used in the fields of web development, data science, machine learning, automation and scripting. 1) In web development, Django and Flask frameworks simplify the development process. 2) In the fields of data science and machine learning, NumPy, Pandas, Scikit-learn and TensorFlow libraries provide strong support. 3) In terms of automation and scripting, Python is suitable for tasks such as automated testing and system management.
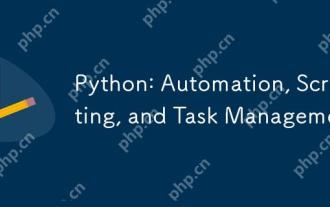
Python excels in automation, scripting, and task management. 1) Automation: File backup is realized through standard libraries such as os and shutil. 2) Script writing: Use the psutil library to monitor system resources. 3) Task management: Use the schedule library to schedule tasks. Python's ease of use and rich library support makes it the preferred tool in these areas.
