Automating OG Images: From Manual Design to API-Driven Generation
The journey from manually creating OpenGraph images to implementing an automated API-driven system represents a critical evolution for growing web applications. Today, I'll share how I transformed this process at gleam.so, moving from individual Figma designs to an automated system handling thousands of images.
The Manual Phase: Understanding the Baseline
Initially, like many developers, I created OG images manually:
// Early implementation const getOGImage = (postId: string) => { return `/images/og/${postId}.png`; // Manually created in Figma };
This process typically involved:
- Opening Figma for each new image
- Adjusting text and elements
- Exporting to the correct size
- Uploading and linking the image
Average time per image: 15-20 minutes.
First Step: Templating System
The first automation step involved creating reusable templates:
interface OGTemplate { layout: string; styles: { title: TextStyle; description?: TextStyle; background: BackgroundStyle; }; dimensions: { width: number; height: number; }; } const generateFromTemplate = async ( template: OGTemplate, content: Content ): Promise<Buffer> => { const svg = renderTemplate(template, content); return convertToImage(svg); };
This reduced creation time to 5 minutes per image but still required manual intervention.
Building the API Layer
The next evolution introduced a proper API:
// api/og/route.ts import { ImageResponse } from '@vercel/og'; import { getTemplate } from '@/lib/templates'; export const config = { runtime: 'edge', }; export async function GET(request: Request) { try { const { searchParams } = new URL(request.url); const template = getTemplate(searchParams.get('template') || 'default'); const content = { title: searchParams.get('title'), description: searchParams.get('description'), }; const imageResponse = new ImageResponse( renderTemplate(template, content), { width: 1200, height: 630, } ); return imageResponse; } catch (error) { console.error('OG Generation failed:', error); return new Response('Failed to generate image', { status: 500 }); } }
Implementing Caching Layers
Performance optimization required multiple caching layers:
class OGCache { private readonly memory = new Map<string, Buffer>(); private readonly redis: Redis; private readonly cdn: CDNStorage; async getImage(key: string): Promise<Buffer | null> { // Memory cache if (this.memory.has(key)) { return this.memory.get(key); } // Redis cache const redisResult = await this.redis.get(key); if (redisResult) { this.memory.set(key, redisResult); return redisResult; } // CDN cache const cdnResult = await this.cdn.get(key); if (cdnResult) { await this.warmCache(key, cdnResult); return cdnResult; } return null; } }
Resource Optimization
Handling increased load required careful resource management:
class ResourceManager { private readonly queue: Queue; private readonly maxConcurrent = 50; private activeJobs = 0; async processRequest(params: GenerationParams): Promise<Buffer> { if (this.activeJobs >= this.maxConcurrent) { return this.queue.add(params); } this.activeJobs++; try { return await this.generateImage(params); } finally { this.activeJobs--; } } }
Integration Example
Here's how it all comes together in a Next.js application:
// components/OGImage.tsx export function OGImage({ title, description, template = 'default' }) { const ogUrl = useMemo(() => { const params = new URLSearchParams({ title, description, template, }); return `/api/og?${params.toString()}`; }, [title, description, template]); return ( <Head> <meta property="og:image" content={ogUrl} /> <meta property="og:image:width" content="1200" /> <meta property="og:image:height" content="630" /> </Head> ); }
Performance Results
The automated system achieved significant improvements:
- Generation time: <100ms (down from 15-20 minutes)
- Cache hit rate: 95%
- Error rate: <0.1%
- CPU usage: 15% of previous implementation
- Cost per image: $0.0001 (down from ~$5 in manual labor)
Key Learnings
Through this automation journey, several crucial insights emerged:
-
Image Generation Strategy
- Pre-warm caches for predictable content
- Implement fallbacks for failures
- Optimize template rendering first
-
Resource Management
- Implement request queuing
- Monitor memory usage
- Cache aggressively
-
Error Handling
- Provide fallback images
- Log failures comprehensively
- Monitor generation metrics
The Path Forward
The future of OG image automation lies in:
- AI-enhanced template selection
- Dynamic content optimization
- Predictive cache warming
- Real-time performance tuning
Simplifying Implementation
While building a custom solution offers valuable learning experiences, it requires significant development and maintenance effort. That's why I built gleam.so, which provides this entire automation stack as a service.
Now you can:
- Design templates visually
- Preview all options for free
- Generate images via API (Open beta-test for lifetime users)
- Focus on your core product
75% off lifetime access ending soon ✨
Share Your Experience
Have you automated your OG image generation? What challenges did you face? Share your experiences in the comments!
Part of the Making OpenGraph Work series. Follow for more web development insights!
The above is the detailed content of Automating OG Images: From Manual Design to API-Driven Generation. For more information, please follow other related articles on the PHP Chinese website!

Hot AI Tools

Undresser.AI Undress
AI-powered app for creating realistic nude photos

AI Clothes Remover
Online AI tool for removing clothes from photos.

Undress AI Tool
Undress images for free

Clothoff.io
AI clothes remover

AI Hentai Generator
Generate AI Hentai for free.

Hot Article

Hot Tools

Notepad++7.3.1
Easy-to-use and free code editor

SublimeText3 Chinese version
Chinese version, very easy to use

Zend Studio 13.0.1
Powerful PHP integrated development environment

Dreamweaver CS6
Visual web development tools

SublimeText3 Mac version
God-level code editing software (SublimeText3)

Hot Topics


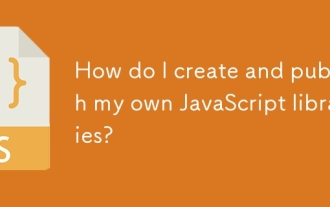
Article discusses creating, publishing, and maintaining JavaScript libraries, focusing on planning, development, testing, documentation, and promotion strategies.
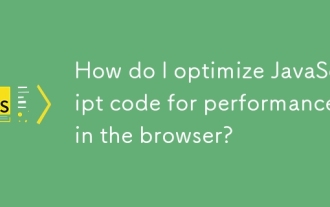
The article discusses strategies for optimizing JavaScript performance in browsers, focusing on reducing execution time and minimizing impact on page load speed.
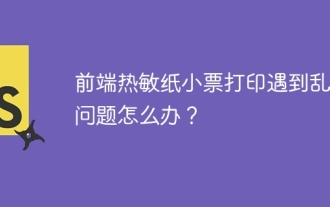
Frequently Asked Questions and Solutions for Front-end Thermal Paper Ticket Printing In Front-end Development, Ticket Printing is a common requirement. However, many developers are implementing...
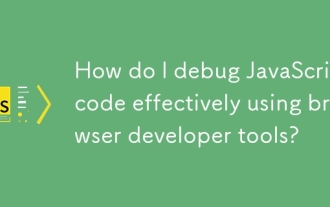
The article discusses effective JavaScript debugging using browser developer tools, focusing on setting breakpoints, using the console, and analyzing performance.
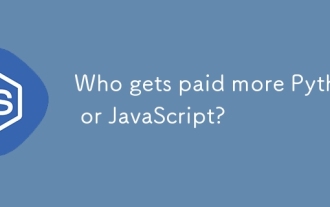
There is no absolute salary for Python and JavaScript developers, depending on skills and industry needs. 1. Python may be paid more in data science and machine learning. 2. JavaScript has great demand in front-end and full-stack development, and its salary is also considerable. 3. Influencing factors include experience, geographical location, company size and specific skills.
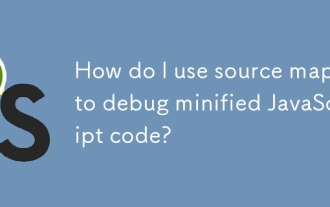
The article explains how to use source maps to debug minified JavaScript by mapping it back to the original code. It discusses enabling source maps, setting breakpoints, and using tools like Chrome DevTools and Webpack.
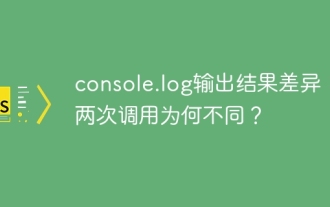
In-depth discussion of the root causes of the difference in console.log output. This article will analyze the differences in the output results of console.log function in a piece of code and explain the reasons behind it. �...
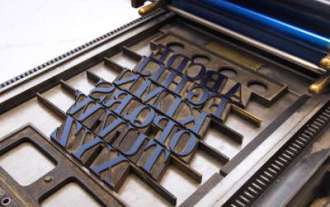
Once you have mastered the entry-level TypeScript tutorial, you should be able to write your own code in an IDE that supports TypeScript and compile it into JavaScript. This tutorial will dive into various data types in TypeScript. JavaScript has seven data types: Null, Undefined, Boolean, Number, String, Symbol (introduced by ES6) and Object. TypeScript defines more types on this basis, and this tutorial will cover all of them in detail. Null data type Like JavaScript, null in TypeScript
