ChatsAPI — The World's Fastest AI Agent Framework
GitHub: https://github.com/chatsapi/ChatsAPI
Library: https://pypi.org/project/chatsapi/
Artificial Intelligence has transformed industries, but deploying it effectively remains a daunting challenge. Complex frameworks, slow response times, and steep learning curves create barriers for businesses and developers alike. Enter ChatsAPI — a groundbreaking, high-performance AI agent framework designed to deliver unmatched speed, flexibility, and simplicity.
In this article, we’ll uncover what makes ChatsAPI unique, why it’s a game-changer, and how it empowers developers to build intelligent systems with unparalleled ease and efficiency.
What Makes ChatsAPI Unique?
ChatsAPI is not just another AI framework; it’s a revolution in AI-driven interactions. Here’s why:
- Unmatched Performance ChatsAPI leverages SBERT embeddings, HNSWlib, and BM25 Hybrid Search to deliver the fastest query-matching system ever built.
Speed: With sub-millisecond response times, ChatsAPI is the world’s fastest AI agent framework. Its HNSWlib-powered search ensures lightning-fast retrieval of routes and knowledge, even with large datasets.
Efficiency: The hybrid approach of SBERT and BM25 combines semantic understanding with traditional ranking systems, ensuring both speed and accuracy.
Seamless Integration with LLMs
ChatsAPI supports state-of-the-art Large Language Models (LLMs) like OpenAI, Gemini, LlamaAPI, and Ollama. It simplifies the complexity of integrating LLMs into your applications, allowing you to focus on building better experiences.Dynamic Route Matching
ChatsAPI uses natural language understanding (NLU) to dynamically match user queries to predefined routes with unparalleled precision.
Register routes effortlessly with decorators like @trigger.
Use parameter extraction with @extract to simplify input handling, no matter how complex your use case.
- Simplicity in Design We believe that power and simplicity can coexist. With ChatsAPI, developers can build robust AI-driven systems in minutes. No more wrestling with complicated setups or configurations.
The Advantages of ChatsAPI
High-Performance Query Handling
Traditional AI systems struggle with either speed or accuracy — ChatsAPI delivers both. Whether it’s finding the best match in a vast knowledge base or handling high volumes of queries, ChatsAPI excels.
Flexible Framework
ChatsAPI adapts to any use case, whether you’re building:
- Customer support chatbots.
- Intelligent search systems.
- AI-powered assistants for e-commerce, healthcare, or education.
Built for Developers
Designed by developers, for developers, ChatsAPI offers:
- Quick Start: Set up your environment, define routes, and go live in just a few steps.
- Customization: Tailor the behavior with decorators and fine-tune performance for your specific needs.
- Easy LLM Integration: Switch between supported LLMs like OpenAI or Gemini with minimal effort.
How Does ChatsAPI Work?
At its core, ChatsAPI operates through a three-step process:
- Register Routes: Use the @trigger decorator to define routes and associate them with your functions.
- Search and Match: ChatsAPI uses SBERT embeddings and BM25 Hybrid Search to match user inputs with the right routes dynamically.
- Extract Parameters: With the @extract decorator, ChatsAPI automatically extracts and validates parameters, making it easier to handle complex inputs.
The result? A system that’s fast, accurate, and ridiculously easy to use.
Use Cases
Customer Support
Automate customer interactions with blazing-fast query resolution. ChatsAPI ensures users get relevant answers instantly, improving satisfaction and reducing operational costs.Knowledge Base Search
Empower users to search vast knowledge bases with semantic understanding. The hybrid SBERT-BM25 approach ensures accurate, context-aware results.Conversational AI
Build conversational AI agents that understand and adapt to user inputs in real-time. ChatsAPI integrates seamlessly with top LLMs to deliver natural, engaging conversations.
Why Should You Care?
Other frameworks promise flexibility or performance — but none can deliver both like ChatsAPI. We’ve created a framework that’s:
- Faster than anything else in the market.
- Simpler to set up and use.
- Smarter, with its unique hybrid search engine that blends semantic and keyword-based approaches.
ChatsAPI empowers developers to unlock the full potential of AI, without the headaches of complexity or slow performance.
How to Get Started
Getting started with ChatsAPI is easy:
- Install the framework:
pip install chatsapi
- Define your routes:
from chatsapi import ChatsAPI chat = ChatsAPI() @chat.trigger("Hello") async def greet(input_text): return "Hi there!"
- Extract some data from the message
from chatsapi import ChatsAPI chat = ChatsAPI() @chat.trigger("Need help with account settings.") @chat.extract([ ("account_number", "Account number (a nine digit number)", int, None), ("holder_name", "Account holder's name (a person name)", str, None) ]) async def account_help(chat_message: str, extracted: dict): return {"message": chat_message, "extracted": extracted} Run your message (with no LLM) @app.post("/chat") async def message(request: RequestModel, response: Response): reply = await chat.run(request.message) return {"message": reply}
- Conversations (with LLM) — Full Example
import os from dotenv import load_dotenv from fastapi import FastAPI, Request, Response from pydantic import BaseModel from chatsapi.chatsapi import ChatsAPI # Load environment variables from .env file load_dotenv() app = FastAPI() # instantiate FastAPI or your web framework chat = ChatsAPI( # instantiate ChatsAPI llm_type="gemini", llm_model="models/gemini-pro", llm_api_key=os.getenv("GOOGLE_API_KEY"), ) # chat trigger - 1 @chat.trigger("Want to cancel a credit card.") @chat.extract([("card_number", "Credit card number (a 12 digit number)", str, None)]) async def cancel_credit_card(chat_message: str, extracted: dict): return {"message": chat_message, "extracted": extracted} # chat trigger - 2 @chat.trigger("Need help with account settings.") @chat.extract([ ("account_number", "Account number (a nine digit number)", int, None), ("holder_name", "Account holder's name (a person name)", str, None) ]) async def account_help(chat_message: str, extracted: dict): return {"message": chat_message, "extracted": extracted} # request model class RequestModel(BaseModel): message: str # chat conversation @app.post("/chat") async def message(request: RequestModel, response: Response, http_request: Request): session_id = http_request.cookies.get("session_id") reply = await chat.conversation(request.message, session_id) return {"message": f"{reply}"} # set chat session @app.post("/set-session") def set_session(response: Response): session_id = chat.set_session() response.set_cookie(key="session_id", value=session_id) return {"message": "Session set"} # end chat session @app.post("/end-session") def end_session(response: Response, http_request: Request): session_id = http_request.cookies.get("session_id") chat.end_session(session_id) response.delete_cookie("session_id") return {"message": "Session ended"}
- Routes adhering LLM queries — Single Query
await chat.query(request.message)
Benchmarks
Traditional LLM (API)-based methods typically take around four seconds per request. In contrast, ChatsAPI processes requests in under one second, often within milliseconds, without making any LLM API calls.
Performing a chat routing task within 472ms (no cache)
Performing a chat routing task within 21ms (after cache)
Performing a chat routing data extraction task within 862ms (no cache)
Demonstrating its conversational abilities with WhatsApp Cloud API
ChatsAPI — Feature Hierarchy
ChatsAPI is more than just a framework; it’s a paradigm shift in how we build and interact with AI systems. By combining speed, accuracy, and ease of use, ChatsAPI sets a new benchmark for AI agent frameworks.
Join the revolution today and see why ChatsAPI is transforming the AI landscape.
Ready to dive in? Get started with ChatsAPI now and experience the future of AI development.
The above is the detailed content of ChatsAPI — The World's Fastest AI Agent Framework. For more information, please follow other related articles on the PHP Chinese website!

Hot AI Tools

Undresser.AI Undress
AI-powered app for creating realistic nude photos

AI Clothes Remover
Online AI tool for removing clothes from photos.

Undress AI Tool
Undress images for free

Clothoff.io
AI clothes remover

Video Face Swap
Swap faces in any video effortlessly with our completely free AI face swap tool!

Hot Article

Hot Tools

Notepad++7.3.1
Easy-to-use and free code editor

SublimeText3 Chinese version
Chinese version, very easy to use

Zend Studio 13.0.1
Powerful PHP integrated development environment

Dreamweaver CS6
Visual web development tools

SublimeText3 Mac version
God-level code editing software (SublimeText3)

Hot Topics










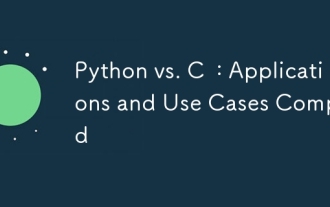
Python is suitable for data science, web development and automation tasks, while C is suitable for system programming, game development and embedded systems. Python is known for its simplicity and powerful ecosystem, while C is known for its high performance and underlying control capabilities.
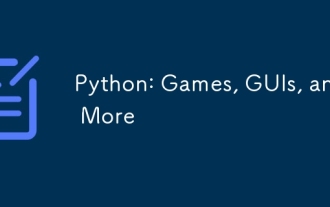
Python excels in gaming and GUI development. 1) Game development uses Pygame, providing drawing, audio and other functions, which are suitable for creating 2D games. 2) GUI development can choose Tkinter or PyQt. Tkinter is simple and easy to use, PyQt has rich functions and is suitable for professional development.
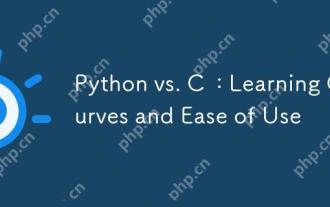
Python is easier to learn and use, while C is more powerful but complex. 1. Python syntax is concise and suitable for beginners. Dynamic typing and automatic memory management make it easy to use, but may cause runtime errors. 2.C provides low-level control and advanced features, suitable for high-performance applications, but has a high learning threshold and requires manual memory and type safety management.
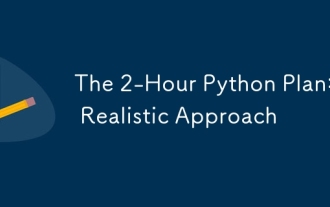
You can learn basic programming concepts and skills of Python within 2 hours. 1. Learn variables and data types, 2. Master control flow (conditional statements and loops), 3. Understand the definition and use of functions, 4. Quickly get started with Python programming through simple examples and code snippets.
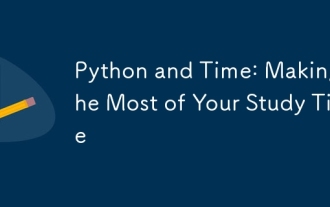
To maximize the efficiency of learning Python in a limited time, you can use Python's datetime, time, and schedule modules. 1. The datetime module is used to record and plan learning time. 2. The time module helps to set study and rest time. 3. The schedule module automatically arranges weekly learning tasks.
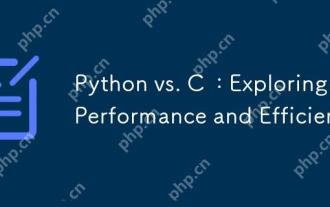
Python is better than C in development efficiency, but C is higher in execution performance. 1. Python's concise syntax and rich libraries improve development efficiency. 2.C's compilation-type characteristics and hardware control improve execution performance. When making a choice, you need to weigh the development speed and execution efficiency based on project needs.
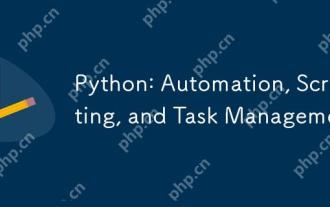
Python excels in automation, scripting, and task management. 1) Automation: File backup is realized through standard libraries such as os and shutil. 2) Script writing: Use the psutil library to monitor system resources. 3) Task management: Use the schedule library to schedule tasks. Python's ease of use and rich library support makes it the preferred tool in these areas.
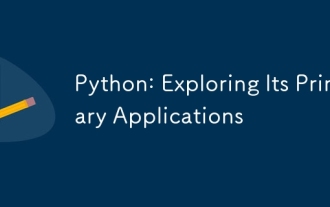
Python is widely used in the fields of web development, data science, machine learning, automation and scripting. 1) In web development, Django and Flask frameworks simplify the development process. 2) In the fields of data science and machine learning, NumPy, Pandas, Scikit-learn and TensorFlow libraries provide strong support. 3) In terms of automation and scripting, Python is suitable for tasks such as automated testing and system management.
