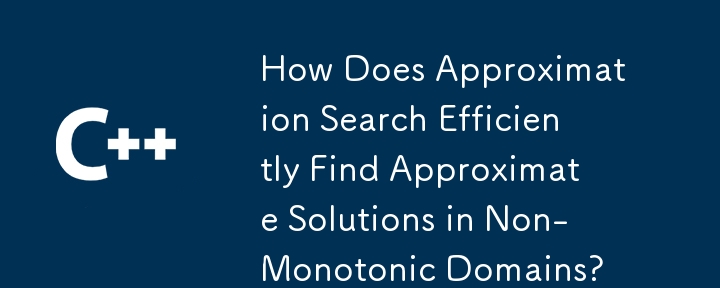
How Approximation Search Works
Approximation search, akin to binary search, enables the efficient approximation of values or parameters within a specified real domain. Unlike binary search, it operates independently of monotonic function restrictions.
Algorithm:
-
Probe evenly dispersed points: Calculate the distance/error for each point within the search interval.
-
Identify minimal error point: Determine the point with the lowest error.
-
Recursively increase accuracy: Adjust the search interval around the minimal error point and refine the search step size.
-
Final solution: Repeat until the desired accuracy is achieved.
Applicability:
Approximation search finds applications in various scenarios, including:
- Approximating solutions to transcendental equations
- Fitting polynomials or parametric functions
- Solving difficult equations when inverse functions are unavailable
- Non-monotonic or non-functional value approximations
Implementation:
The provided C code implements the approximation search algorithm:
class approx { ... };
...
for (aa.init(0.0,10.0,0.1,6,&ee); !aa.done; aa.step()) { ... }
Copy after login
Usage:
- Define an approx object (aa).
- Initialize it with parameters a0, a1, da, n, and a pointer to the error variable ee.
- Iterate through the loop to perform the approximation search. The final solution is stored in aa.a.
Key Points:
- Careful interval and step size selection is crucial.
- The algorithm explores the possibility of multiple solutions for non-functional fits through recursive subdivision.
- Nested multidimensional fits require careful consideration for performance.
The above is the detailed content of How Does Approximation Search Efficiently Find Approximate Solutions in Non-Monotonic Domains?. For more information, please follow other related articles on the PHP Chinese website!