


How Can I Efficiently Manage and Process 'Large Data' with Pandas?
Workflows for "Large Data" in Pandas
When dealing with datasets too large to fit in memory but small enough for a hard drive, it is essential to establish effective workflows to manage "large data." This article explores best practices for importing, querying, and updating data using tools like HDFStore and MongoDB.
Workflow for Large Data Manipulation with Pandas
Loading Flat Files into a Permanent Database Structure
To load flat files into a permanent on-disk database, consider using HDFStore. This allows you to store large datasets on disk and retrieve only the necessary portions into Pandas dataframes for analysis.
Querying the Database to Retrieve Data for Pandas
Once the data is stored, queries can be executed to retrieve data subsets. MongoDB is an alternative option that simplifies this process.
Updating the Database After Manipulating Pieces in Pandas
To update the database with new data from Pandas, append the new columns to the existing database structure using HDFStore. However, it is crucial to consider data types when appending new columns, as this can affect efficiency.
Real-World Example
The following example demonstrates a typical scenario where these workflows are applied:
- Import large flat files: Iteratively import large flat-file data into a permanent on-disk database structure.
- Query pandas dataframes: Query the database to retrieve subsets of data into memory-efficient Pandas dataframes.
- Create new columns: Perform operations on the selected columns to create new compound columns.
- Append new columns: Append the newly created columns to the database structure using, for example, HDFStore.
Additional Considerations
When working with large data, it is important to define a structured workflow, such as the one described above. This helps minimize complications and enhances data management efficiency.
Another key aspect is understanding the nature of your data and the operations being performed. For instance, if row-wise operations are being conducted, storing data in row-wise format (e.g., using pytables) can improve efficiency.
It is also crucial to determine the optimal balance between storage efficiency and query performance. Employing compression techniques and establishing data columns can optimize storage space and expedite row-level subsetting.
By adhering to these best practices when working with large data in Pandas, you can streamline your data analysis processes and achieve better performance and reliability.
The above is the detailed content of How Can I Efficiently Manage and Process 'Large Data' with Pandas?. For more information, please follow other related articles on the PHP Chinese website!

Hot AI Tools

Undresser.AI Undress
AI-powered app for creating realistic nude photos

AI Clothes Remover
Online AI tool for removing clothes from photos.

Undress AI Tool
Undress images for free

Clothoff.io
AI clothes remover

Video Face Swap
Swap faces in any video effortlessly with our completely free AI face swap tool!

Hot Article

Hot Tools

Notepad++7.3.1
Easy-to-use and free code editor

SublimeText3 Chinese version
Chinese version, very easy to use

Zend Studio 13.0.1
Powerful PHP integrated development environment

Dreamweaver CS6
Visual web development tools

SublimeText3 Mac version
God-level code editing software (SublimeText3)

Hot Topics
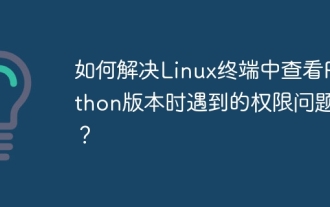
Solution to permission issues when viewing Python version in Linux terminal When you try to view Python version in Linux terminal, enter python...
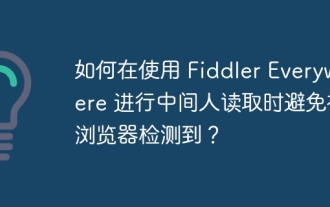
How to avoid being detected when using FiddlerEverywhere for man-in-the-middle readings When you use FiddlerEverywhere...
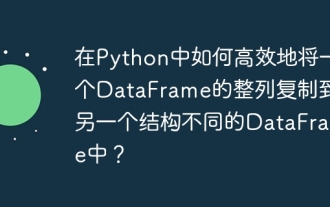
When using Python's pandas library, how to copy whole columns between two DataFrames with different structures is a common problem. Suppose we have two Dats...
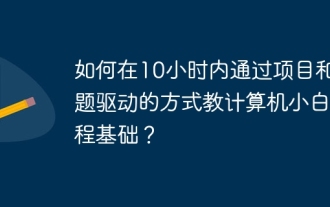
How to teach computer novice programming basics within 10 hours? If you only have 10 hours to teach computer novice some programming knowledge, what would you choose to teach...
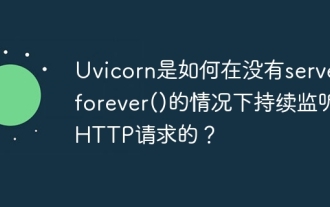
How does Uvicorn continuously listen for HTTP requests? Uvicorn is a lightweight web server based on ASGI. One of its core functions is to listen for HTTP requests and proceed...
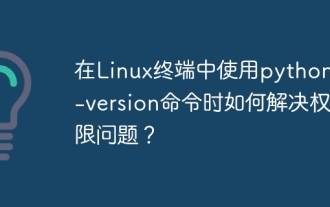
Using python in Linux terminal...
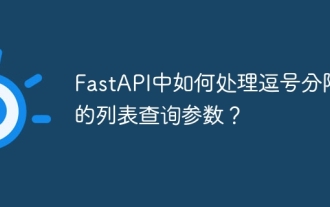
Fastapi ...
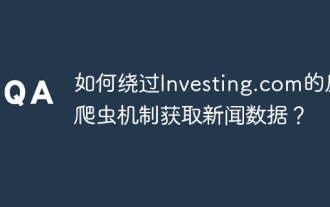
Understanding the anti-crawling strategy of Investing.com Many people often try to crawl news data from Investing.com (https://cn.investing.com/news/latest-news)...
